Introduction
Population dynamics is concerned with changes in a population over time. Understanding these dynamics facilitates the early detection of growing or declining trends in a population. If necessary, appropriate control or protection strategies can then be introduced timeously to deal with undesirable trends (Mertens, Reference Mertens1985; Jolles, Reference Jolles2007). This is needed both for the conservation of African buffalo (Syncerus caffer) and for designing optimal strategies for their harvesting (e.g. hunting) or even ranching (Chapters 13 and 16).
Several studies (e.g. Sinclair, Reference Sinclair1977; Mertens, Reference Mertens1985) collected data in the field and subsequently processed these data to produce life tables of buffalo populations. For humans, actuaries use life tables to determine the expected life expectancy of an individual, and hence perhaps the appropriate life insurance premium for that individual to pay. Due to practical difficulties in collecting data, life tables for buffalo are generally less accurate than those for humans. More importantly, in wildlife management the purpose of life tables is different. Forecasting is essential for good management and life table data can be used in a model to project a population into the future. Even for this purpose, however, the utility of such a model is limited. Population dynamics of buffalo populations are frequently event-driven. For example, droughts, disease and poaching may invalidate any forecast based on life tables. Moreover, wildlife managers require scenario-based rough estimates rather than precise population projections into the future. Roughly what proportion of a herd will die if a severe drought occurs next year? Hence, should any intervention be considered? Indeed, for a herd of 500 buffalo, the answer to this last question is not likely to differ whether there is an expected loss of 105 or 125 animals.
Investigating the dynamics of a population usually involves the use of mathematical models. There is a diverse array of such models. Therefore, before commencing any formulation or use of a model it is important to be very clear about its purpose. What are the questions for which one seeks answers? What understanding of system behaviour is sought? Only once the aims and the purpose of the model are clear can the appropriate type of model be chosen.
In this chapter, we first discuss various exogenous factors that disrupt population trajectories. We focus particularly on drought and disease. This is followed by a discussion on the constraints that these factors play in the types and goals of models that can provide any useful insight. Finally, we show the importance of age structure in determining population dynamics. We will illustrate how aggregated population data might in fact mislead observers.
Factors Affecting Population Dynamics
Droughts
The rinderpest outbreak in Africa in the 1890s reached southern Africa around 1896, peaked in 1897–1898 and severely impacted artiodactyl populations in Africa. Stevenson-Hamilton (Reference Stevenson-Hamilton1929) reported that the disease ‘…reduced the already much depleted buffalo herds to about a dozen individuals’ in what is now Kruger National Park (KNP). Impressive conservation efforts during the early twentieth century and the recovery of rangelands following the large-scale, disease-related grazer die-off contributed to the recovery of game populations. However, African buffalo remain susceptible to drought (Sinclair, Reference Sinclair1977; Smuts, Reference Smuts1982; Walker et al., Reference Walker, Emslie, Owen-Smith and Scholes1987; Prins, Reference Prins1996; Peel and Smit, Reference Peel and Smit2020; Smit et al., Reference Smit, Peel and Ferreira2020). As the doyen of South African nature conservation, James Stevenson-Hamilton observed, ‘During the severe drought at the end of 1935, when the rains failed completely, and the Lower Sabie area was quite bare of grass, the buffaloes suffered terribly’ (Stevenson-Hamilton, Reference Stevenson-Hamilton1947, p. 85). This pattern of sensitivity to drought is further illustrated by, among others, Walker et al. (Reference Walker, Emslie, Owen-Smith and Scholes1987) describing the impact of the severe 1981–1984 drought, exacerbated by water provision, that affected most of southern Africa, causing extensive grass mortality and grazer mortality in particular. Peel and Smit (Reference Peel and Smit2020) describe declines in the buffalo population of between 77 per cent in an area that was minimally managed and declines of 29 per cent and 35 per cent, respectively, in areas where management removals were carried out before the 2014/15–2016/17 drought developed fully. Furthermore, regional buffalo population declines were highest in areas with a high density of artificially provided water points and associated low herbaceous biomass (Smit et al., Reference Smit, Peel and Ferreira2020).
Stevenson-Hamilton (Reference Stevenson-Hamilton1947, p. 85) further states, ‘Such few calves as were born did not survive…’. This is a very pertinent observation, yet more detailed observations appear to indicate that recently weaned juveniles are the first to die, followed by suckling calves, both groups being very susceptible to drought conditions (Prins and Peel, personal observations at numerous places during some droughts). During the 1969–1970 drought in KNP, Smuts (Reference Smuts1982) once observed five buffalo calves dying while the herd was moving between two water points some 9 km apart. Further, observations of calves wandering through the veld alone were common, as they could not keep up with the herds moving over large areas in search of food and water. The proportions of calf and juvenile groups in the buffalo population were extremely low after the 2014/15–2015/16 drought, and these statistics feature in our detailed discussion of individual protected areas.
We examined three protected area (PA) scenarios under varying environmental and management regimes in the same semi-arid savanna (Lehmann et al., Reference Lehmann, Archibald, Hoffmann and Bond2011; Luvhuno et al., Reference Luvhuno, Biggs, Stevens and Esler2018) in the Lowveld of South Africa as described by Stevenson-Hamilton (Reference Stevenson-Hamilton1947). The three PAs represent an environmental gradient with decreasing long-term mean annual rainfall from south to north (PA 1: 631 mm, PA 2: 552 mm and PA 3: 430 mm). While focusing on what we consider the ‘long’ 2014/15 and 2015/16 droughts, it is important to describe the conditions prior to the onset of the drought. For all three PAs, the two-year pre-drought mean varied from wet (PA 1 and PA 2) to very wet (PA 3). The rainfall received as a percentage of the long-term mean annual rainfall in year one of the drought varied between 54 per cent for the wetter south to around 77 per cent for the ‘drier’ central and northern areas. The second year of the drought showed the same pattern for the PAs, with the ‘wetter’ southern PA 1 receiving 38 per cent, the central fenced PA 2 57 per cent and the drier northern PA 3 65 per cent of the mean annual rainfall, respectively. The post-drought rainfall was at the mean annual rainfall for PA 1 and marginally below the mean for PA 2 and PA 3. Rainfall correlates well with the grass standing crop (GSC) – the two-year pre-drought mean for the GSC ranged from 123 per cent for PA 1, 159 per cent for PA 2 and 152 per cent for PA 3. The entry point to the drought in terms of grass availability was thus very favourable (Figure 7.1a–f). Year 1 of the drought was characterized by marked declines in the GSC, ranging from 70, 67 to 72 per cent of the long-term mean for PAs 1–3, respectively. The second drought year precipitated an almost complete failure of the grassland crop with the mean GSC ranging from 7, 6 to 9 per cent for PAs 1–3, respectively. Recovery of the GSC post-drought again tracked a return to improved rainfall conditions (Figure 7.1a–f). Similar precipitous declines in standing crop (and subsequent yearling mortality) were observed in Tarangire National Park (Tanzania) in 1992 when minimal perennial grass cover was left, and in KNP in 1993 and again in 2004 with similar effects (Prins, personal observation). The periodicity of the droughts in the southern African climatic system is well illustrated in Malherbe et al. (Reference Malherbe, Smit, Wessels and Beukes2020), where it appears to be associated with the Southern Atlantic Oscillation (see also Gandiwa et al., Reference Gandiwa, Heitkönig, Eilers and Prins2016 and Poshiwa et al., Reference Poshiwa, Groeneveld and Heitkönig2013). Droughts in East Africa do not show clear periodicity (Prins and Loth, Reference Prins and Loth1988) because rainfall there is driven much more by monsoonal effects over the Indian Ocean.

Figure 7.1(a–f) Buffalo population data and rangeland conditions pre-, during and post-drought on three protected areas in the Lowveld of South Africa.
We evaluated how buffalo numbers changed after a drought event in the three PAs located in the Lowveld of South Africa and located along a south–north gradient. In our study, we considered buffalo numbers and the susceptible calf groups (up to 2 years old). Buffalo numbers were calculated as a percentage of the pre-drought number (Figure 7.1a, c and e) and for calves as a percentage of the population as a whole (Figure 7.1b, d and f).
In PA 1 (Figure 7.1a – wetter, and open to KNP), the population maintained itself in the first year of the drought, followed by a marked decline in the second year, but the population remained healthy. It was only during the winter of the second year of drought with the collapse of the grass layer that the numbers declined spectacularly. The latter was a result of a combination of movement out of the heavily stocked PA 1 into the adjacent KNP (less artificial water point provision and lower animal densities), and drought-related mortality through starvation and predation due to the animals’ weakened condition. The proportion of calves present in the population was maintained during the first year of the drought (favourable drought entry conditions), declined markedly in the second year and plummeted in the first post-drought year, a function of the die-off in the winter of year two and reflected in recovery year two post-drought as the rangelands recovered (Figure 7.1b). The recovery of the ‘rested’ rangeland in these wetter southern areas was illustrated by the return to pre-drought calf proportions in the second post-drought year. The overall reduced population size after the drought indicates that the older age classes also lost individuals, likely caused by increased predation and starvation.
In PA 2 (Figure 7.1c – intermediate rainfall and fenced), 98 buffalo (11 per cent of the population) were removed on recommendation between 2014 and 2015 with an additional 28 animals removed in 2016 (11 per cent of a much-reduced population). While these removals were commendable, they did not prevent marked declines in year one of the drought and steep declines in the following two years resulting in levels of around 20 per cent of pre-drought numbers up to three years post-drought. We consider that this situation was due to the heavy prevailing stocking rates at the start of the drought and rapid declines in the grass layer at the onset of the drought due to the fenced situation, resulting in drought-related mortality through predation on weakened animals and starvation. The proportion of calves present in the population was maintained in the first year of the drought (favourable drought entry conditions), declined steeply in the second year of drought and year one post-drought (to 0 per cent) with minimal recovery in year two post-drought and improvement in only year three post-drought but to levels still below pre-drought (Figure 7.1d). The population continued to recover slowly due to numbers below the exponential phase of the growth curve in this fenced PA. Without supplementation from outside sources, the rangeland would have rested in this wetter, albeit fenced central area. We would expect the population to recover, the rate dependent on the prevailing rainfall conditions and associated range condition that in turn affects how quickly the growth curve reaches exponential.
In PA 3 (Figure 7.1e, drier open to KNP) there was a decline in the number of buffalo in the first year of the drought followed by an increase in the second year of drought. This is anomalous as the grass layer was already severely limiting and probably the result of buffalo moving through the area at the time of the count from the surrounding drier waterless areas where conditions were more severe. The steep decline in the buffalo population was therefore only recorded in the first-year post-drought and continued in the second-year post-drought due to a continued decline in the grass layer combined with movement out of the PA (see e.g. Hilbers et al., Reference Hilbers, van Langevelde and Prins2015), predation due to the animals being in a weakened condition and starvation. This pattern was only reversed in the third-year post-drought. Although the buffalo population ‘increased’ in year two of the drought, the proportion of calves was very low, supporting the argument that the animals present in this PA were in all likelihood moving through the area in search of water and grazing. The proportion of calves present in the population was maintained in the first year of the drought (favourable drought entry conditions), declined steeply in the second year of drought and continued to decline markedly for the three years post-drought (3, 2.6 and 0 per cent; Figure 7.1f). We contend that the slower recovery of the population is a function of the slower recovery in the calf component and even greater susceptibility to drought in these drier, less-resilient areas.
Disease
Buffalo are remarkably resistant to indigenous diseases, but can be negatively affected by anthrax (Bacillus anthracis; e.g. Hugh-Jones and De Vos, Reference Hugh-Jones and De Vos2002; Clegg et al., Reference Clegg, Turnbull, Foggin and Lindeque2007). They are not known to succumb easily to different forms of trypanosomes transmitted by tsetse flies (see Garcia et al., Reference Garcia, Rodrigues and Rodrigues2018). These parasitic forms, some of which may cause sleeping sickness in humans, are destroyed by phagocytes (Young et al., Reference Young, Kanhai and Stagg1975), but other forms of trypanotolerance may occur (e.g. Murray et al., Reference Murray, Trail, Davis and Black1984). They are also resistant to other diseases like corridor disease caused by Theileria p. parva and East Coast fever caused by T. p. lawrenci. East Coast fever was introduced into South Africa in 1902 by infected cattle imported from East Africa to restock depleted cattle numbers after the rinderpest epidemic of 1896 (Lawrence, Reference Lawrence1979). The subsequent epidemic lead to an estimated 5.5 million cattle deaths (Potgieter et al., Reference Potgieter, Stoltsz, Blouin and Roos1988) but no buffalo epidemic was reported. Buffalo are also resistant to Anaplasma forms (Sisson, Reference Sisson2017). For an overview of other diseases, see Chapters 9 and 12. Some exotic diseases, however, were disastrous and buffalo had no immunity or resistance. Rinderpest was the most notorious, with a death toll exceeding 90 per cent (Prins, Reference Prins1996, p. 122 and references therein). The disease has been eradicated. Other exotic diseases, however, did not affect buffalo too badly. A case in point is bovine tuberculosis (bTB). We thus think that diseases exert a rather constant pressure in contrast to droughts that are punctuated events with frequently severe consequences for young buffalo in particular.
Implications for Modelling
Clearly, there are many factors that may cause major losses in population numbers. Furthermore, it is rare to find two consecutive decades within which such a loss does not occur. Consequently, a population in the wild will mostly, if not always, be in some transitional stage. Thus, transient dynamics will dominate rather than any form of density-dependent growth or equilibrium, and concepts of carrying capacity become irrelevant. In such situations, any analysis involving aggregation of age groups may lead to incorrect conclusions. Population trajectories are affected by how age structures are distributed. Two populations that have the same total number of head at some point in time might exhibit very different dynamic behaviour for several years thereafter due to the populations having different age structures. Distinguishing a population by age groups is therefore important both from a data and a modelling perspective, and the more precise, the better. In the following sections, we will explore these issues further.
Modelling
We constructed a model to gain insight into the relationship between aggregated population data, such as that available from a field survey, and the age structure of a population. We looked at the implications for forecasting population trends as well as for drawing conclusions about possible density effects. To have a clear, uncluttered focus on this, we considered a population for which the following assumptions hold for the solution period:
No migration
No losses due to disease, predation, culling, poaching or other exogenous factors
No constraining limits related to space and grazing resources within the time
A population large enough so that we can ignore stochastic changes in vital rates.
We also assumed that there would be enough males in a population to service the females. In this case, the population dynamics are determined by the females.
Leslie Model
Let x(t) denote the vector whose ith element, xi(t), is the female population in age group i at time t. We can project the population in each age group forward one year by the following equation:

The matrix A is known as a Leslie matrix (Caswell, Reference Caswell2001). With subscripts denoting the age groups, a Leslie matrix comprises the following:
The first row of A contains the specific fecundity rates
The ith row of A contains the survival rate si – 1 in column i – 1
All other elements of the matrix A are zero.
Thus:

Stationary Age-Structure
From mathematical theory (Caswell, Reference Caswell2001), we know that applying equation (7.1) repeatedly for t = 1, 2, 3, … will eventually lead to a stationary age structure. By this, we mean that the population in each age group as a proportion of the total population remains constant even as the total population might grow or decline. These proportions (i.e., the age structure) can be obtained by calculating the eigenvector of matrix A corresponding to its largest eigenvalue, λ. Furthermore, the value of λ yields information about the annual growth rate of the population. If λ = 1, the population will remain constant, whereas a value of λ greater than or less than one indicates that the population is growing or declining, respectively.
Ricker Model
A population subject to density-dependent growth is frequently modelled using the Ricker model:

where p is the population, r is the intrinsic annual growth rate and K is the maximum stocking rate, often referred to as the carrying capacity. For unbounded populations, K is infinitely large. In this case, the relationship between λ and r is given by:

This quantity will be referred to simply as the growth rate for the remainder of this chapter.
Data
Data from three different studies were used in the analyses that follow: namely, Serengeti National Park (Sinclair, Reference Sinclair1977), Hluhluwe–iMfolozi Park (Jolles, Reference Jolles2007) and Virunga National Park (Mertens, Reference Mertens1985). These data are shown in Figure 7.2 (precise values are given in the Appendix, Table A7.1). Small adjustments have been made to the age groups in Jolles (Reference Jolles2007) to facilitate comparison.
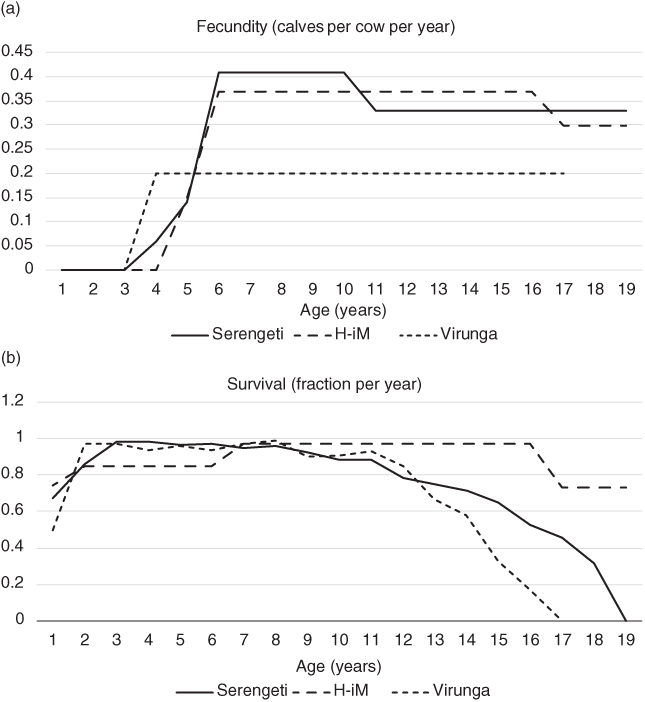
Figure 7.2 Specific fecundity (a) and survival rates (b) for buffalo as given in three different studies: Serengeti (Sinclair, Reference Sinclair1977), Hluhluwe–iMfolozi (Jolles, Reference Jolles2007) and Virunga (Mertens, Reference Mertens1985). The age group refers to the age of the animals in a cohort.
Analysis
There are three parts to this section. First, we explore the population dynamics using the Serengeti data and make some observations. In the second and third parts, we examine some of the conclusions drawn in the studies of Jolles (Reference Jolles2007) and Mertens (Reference Mertens1985), respectively.
Serengeti
The data in Sinclair (Reference Sinclair1977) were a result of several years of work in the Serengeti during the 1960s. It is the most cited work on the fecundity and survival rates of the African buffalo.
Substituting the Serengeti fecundity and survival rates into the matrix (7.2), we find the largest eigenvalue λ and the corresponding eigenvector. The eigenvector corresponds to the stationary age distribution, which we will use as starting values for simulating the population dynamics. The eigenvalue λ = 1.054 implies a growth rate of 5.4 per cent for this stationary age distribution. However, as discussed, populations are subject to frequent disruptions. After such a disruption, how long will it take before the population returns to this stationary age distribution? We consider two plausible scenarios to explore this question.
Serengeti Scenario 1 – A Short Drought
Starting with a stationary age distribution, a population is subject to a short, acute drought of 6 months. This results in the deaths of a major proportion of the recently weaned calves. Specifically, in our model, 75 per cent of the second and third age classes die immediately after the start of year 2.
To simplify the visual representation of the results we aggregate the 19 age groups as follows: calves (group 1), juveniles (groups 2–4), subadults (group 5) and adults (groups 6–19). A division of adults into ‘young’ cows (groups 6–10) and ‘old’ cows is used for some results. This division corresponds to specific fecundity rates as given by Sinclair and shown in Figure 7.2.
It can be observed in Figure 7.3a that more than a decade after the drought event, the population has not yet returned to its previous growth rate. In Figure 7.3b, we note that the age structure of the population is also unsettled. A consequence of this is that the number of calves produced per cow fluctuates as shown in Figure 7.4a. Why should this be, given that the specific fecundity rate for each year-age group is constant?
Dividing the ‘adult’ group into ‘young’ (groups 6–10) and ‘old’ (groups 11–19), we observe in Figure 7.4b that the proportion of cows in each group fluctuates over the solution period. As the ‘young’ and ‘old’ cows have different specific fecundity rates of 0.41 and 0.33, respectively, changes in the proportions of each group cause a change in the average number of calves produced per adult cow.

(a) Growth rate

(b) age structure.
Figure 7.3 The effect of a short, acute drought on the dynamics of a population initially in a stable age distribution.

(a) Fluctuations in the rate of calf production by a herd of buffalo cows.

(b) Changes in the proportion of high fecundity ‘young’ cows to ‘old’ cows.
Serengeti Scenario 2 – A Long Drought
Starting with a stationary age distribution, a population is subjected to a severe drought lasting 2 years. This results in high mortality for recently weaned calves. Specifically, we impose in the model a die-off of 75 per cent of females in groups 2 and 3 in year 2. This is followed in year 3 by a complete die-off of all calves and juveniles, that is all animals in groups 1–4, and 50 per cent of cows staying barren.
In some respects, Figures 7.5 and 7.6 show exaggerated versions of the effects already noted in scenario 1, but there is more to learn from these results.

Figure 7.5 Fluctuations in (a) population and growth rate and (b) age structure.

(a) Number of calves produced per adult cow each year.

(b) Composition of the adult female population.
Suppose a field study commences in year 4 and is concluded in year 10. Further, suppose that no other data were available other than that obtained over the six-year study period. Observing the population trajectory (Figure 7.5a) over this period, it is easy to conclude (wrongly) that population growth is density-dependent. In fact, a Ricker model with K = 95 and r = 0.61 gives an almost perfect fit to these data. The declining number of calves produced per cow over this period (Figure 7.6a) would tend to confirm a conclusion that the population is subject to density-dependent growth. And yet there is no density effect built into the model that generated these results as can be seen from the population trajectory beyond year 11. The transient dynamics of a disturbed population can easily mislead.
Discussion of the Serengeti Cases
Even a short disturbance (6-month drought) affects the population dynamics for more than a decade.
A study of 6 years may produce trajectories that lead to misconceptions about the longer-term prospects of a population.
With no density constraints on a population, transient changes in the age structure may lead to a population trajectory mimicking density-dependent growth over a period lasting a few years.
Specific fecundity rates cannot be accurately determined using aggregated adult age groups for populations in transition.
Hluhluwe–iMfolozi
Demographic data from 826 buffalo in 12 herds in the Hluhluwe–iMfolozi Park, captured in 2001–2002, were used by Jolles (Reference Jolles2007) to parameterize an age-structured population model. The stationary age distribution predicted by the model is, according to Jolles, ‘very similar to the age distribution observed in the captured population sample’. Jolles goes on to use some of her results to provide evidence of density-dependent population growth. We analyse whether these conclusions stand up to further scrutiny.
The first problem we come across is in the age-structured population data given in Jolles (Reference Jolles2007) and shown in Table 7.1. Let us assume that the given number of subadults (= 42) is correct. The survival rate for the juvenile group is given as 0.85. Thus, to get 42 subadults we would need to have 42/0.85 = 49.4 juveniles in the age range from 3.5 to 4.5 years. Similarly, the population in the age range from 5.5 to 6.5 years would be 42 × 0.85 = 35.7 and the next year class would be 35.7 × 0.97 = 34.6. We can continue in this way and determine the numbers in all age groups. Then aggregating these into broader groups, we get the ‘Revised’ values given in Table 7.1. As can be seen, these numbers differ significantly from the values reported by Jolles.
Table 7.1 Age structure for African buffalo in Hluhluwe-iMfolozi Park, South Africa as reported by Jolles (Reference Jolles2007). The revised estimates for the number of buffalo in the different age classes (N) were obtained by assuming the subadult number of 42 (the number published) is correct and then using the survival data provided in the same article for each year-age group to generate the numbers in the ‘Revised’ column as described in the previous paragraph.
Age (years) | Jolles (N) | Revised (N) |
---|---|---|
Calves (<1) | 136 | 109 |
Juveniles (1 = 4.5) | 366 | 256 |
Subadults (>4.5–5.5) | 42 | 42 |
Mature (>5.5) | 247 | 408 |
Is it possible to understand the discrepancy in the two sets of values in Table 7.1 using the simple model presented earlier by equations 7.1 and 7.2? The first step to take is to substitute the fecundity and survival rates determined by Jolles, given in Figure 7.2, into the Leslie matrix (equation 7.2).
The largest eigenvalue of the Leslie matrix in this case indicates a growth rate of 3.4 per cent per annum. This is less than the average 6.8 per cent recorded over the period from 1957 to 2004. The associated stationary age structure is given in Table 7.2 where it is compared with the field data. This difference suggests that a stationary age distribution had not been attained at the time the field data were recorded. This is not surprising given droughts and removals over this period.
Table 7.2 The stationary age structure for Hluhluwe–iMfolozi Park, South Africa as calculated on the basis of the Leslie model compared with field data reported by Jolles (Reference Jolles2007). Values shown (rounded to the nearest integer) are percentages of the total population.
Age (years) | Leslie model (%) | Data (Jolles) (%) |
---|---|---|
Calves (<1) | 17 | 17 |
Juveniles (1 = 4.5) | 30 | 46 |
Subadults (>4.5–5.5) | 7 | 5 |
Mature (>5.5) | 47 | 31 |
Let us now impose on this system the same drought described in Serengeti – scenario 2 to investigate the population dynamics. For this, we used equation 7.1 with the same Leslie matrix comprising the fecundity and survival rates. We assume an initial population of 100 head and determine the age distribution from the eigenvector of the Leslie matrix. Some results are shown in Figure 7.7.

(a) Fluctuations in the population and its growth rate.

(b) The relative proportion of ‘adult’ cows (6–16 years old) to ‘senescent’ cows (17+ years).
We observe in Figure 7.7a that during years 4–11 the population displays a trajectory that might easily be interpreted as density-dependent growth. Applying a Ricker model with K = 117 and r = 0.45 gives an almost perfect fit to the population trajectory over this 7-year period, and yet the population trajectory has been generated by a model that has no density-dependence as is apparent in later years. How can we explain this?
The changes in growth rate observed in Figure 7.7a are purely a result of changes in the age structure. It can be seen in Figure 7.7b that the proportion of ‘adult’ cows (6–16 years old) is depressed between the years 5 and 17. Recall from Figure 7.2 that ‘adult’ cows in Hluhluwe have a specific fecundity rate of 0.37 compared with only 0.3 for ‘senescent’ cows. Therefore, the number of calves produced by a population, and thus the growth rate of the whole population, will be affected by the ratio of ‘adult’ cows to ‘senescent’ cows.
Finally, it should be noted in Figure 7.7b that the population has not settled into a stationary age structure nearly two decades after the drought.
Discussion of the Hluhluwe–iMfolozi Case
If we have accurate fecundity and survival rates, we can easily test whether a population has attained a stationary age structure. The number in any age group multiplied by the survival rate should give the number in the next age group. This will be true for all age groups if we have a stationary age structure.
It takes nearly two decades after a severe year drought before the population settles into a stationary age structure.
Due to the frequency of droughts a population is likely to always be in a transient state.
For populations in a transient state, determining vital rates from aggregated data can be misleading. Furthermore, even a field study with a 7-year duration might yield data that lead to an incorrect conclusion of density-dependent growth.
Virunga
In an analysis of buffalo in Virunga National Park in the Democratic Republic of the Congo (then Zaire), Mertens concluded in 1985 that the structure of the population had remained approximately stable over the past 25 years. Let us investigate this further. We apply to the matrix A, given in equation 7.1, the lifetable data constructed by Mertens (Figure 7.2), which differs to some extent from that of Sinclair (Reference Sinclair1977).
As described previously, the stationary age structure can be obtained by calculating the eigenvector of matrix A corresponding to its largest eigenvalue, λ. Furthermore, the value of λ can be used in equation 7.4 to calculate the growth rate of the population.
For the data given in Figure 7.2, we obtain the value of the largest eigenvalue λ = 0.974. This implies that the population will experience a continuing decline once it has settled into a stationary age structure. Although Mertens provides vital rates for 17 age groups, his population counts and age structure are given in only three groups: ‘young’ (0–1 years), ‘immatures’ (1–4 years) and ‘adults’ (4+ years). Clustering age groups from the eigenvector to enable a direct comparison with Mertens’ data, we get Table 7.3.
Table 7.3 Comparison of the stationary age structure of African buffalo in Virunga National Park, Democratic Republic of Congo obtained by our model with the field data of Mertens (Reference Mertens1985).
Age groups (year) | Model (%) | Mertens (%) |
---|---|---|
<1 (Young) | 14.5 | 8.7 |
=1–4 (Immatures) | 22.2 | 15.9 |
>4–17 (Adults) | 63.3 | 75.4 |
The two age structures in Table 7.3 differ. To explore this, we first note that the age structure reported by Mertens shows that the proportion of each group differs by less than 3 per cent from two previous findings at Virunga National Park in 1960 and 1978–1979. Therefore, we set initial population values close to those of Mertens and used the Virunga vital rates given in Figure 7.2. We then applied equation 7.1 repeatedly to project the population forward in time. The simulations were performed for 30 years, but it is clear from the results that the age groups stabilized after about 25 years as shown in Figure 7.8.

Figure 7.8 Changing age structure over 30 years. Compared with the initial structure at t = 1, both juveniles and subadults ended up representing higher percentages of the population, while adults are lower.
In reporting his data, Mertens allowed for the possibility that his fecundity data were a bit too low and that Sinclair’s values might be more accurate. We thus replaced Virunga fecundity data in Figure 7.2 with those for Serengeti to determine whether this reduces the difference between the model output and the field data. In fact, this replacement increased the discrepancy further, as shown in Table 7.4 (Model 1 as compared to the output based on Merten’s data). Using Serengeti data for both fecundity and survival also did not improve matters (Model 2 in Table 7.4).
Table 7.4 Comparison of the stationary age structure determined from population counts in Virunga National Park, the Democratic Republic of Congo by Mertens (Reference Mertens1985) and that obtained using a Leslie matrix model. Model 1 used Mertens’ survival data but with Sinclair’s (Reference Sinclair1977) fecundity data. Model 2 used Sinclair’s data on fecundity as well as on survival.
Age group (year) | Mertens (%) | Model 1 (%) | Model 2 (%) |
---|---|---|---|
<1 (Young) | 8.7 | 19 | 18 |
=1–4 (Immatures) | 15.9 | 26.3 | 29 |
>4–17 (Adults) | 75.4 | 54.7 | 53 |
Discussion of the Virunga Case
Starting with the age structure recorded, it takes nearly two decades before the population settles. It then settles into an age structure that differs from that recorded by Mertens.
Given this inconsistency and the frequent disturbances to buffalo populations, it seems unlikely that the age structure of the Virunga population was stable over 25 years as claimed. We conclude this, even though the broad population structure recorded by Mertens was similar to that recorded 25 years earlier.
Conclusion
We have noted that factors such as disease, predation, poaching and droughts all have severe effects on buffalo populations, but that droughts (because they occur punctuated in time) have the most intriguing consequences. These events happen frequently. Three major droughts, for example, have occurred in less than four decades in the Greater Kruger National Park (Malherbe et al., Reference Malherbe, Smit, Wessels and Beukes2020; Smit et al., Reference Smit, Peel and Ferreira2020) and severe droughts were also reported by Stevenson-Hamilton (Reference Stevenson-Hamilton1947) decades previously. Such droughts may increase in frequency with climate change in some parts of the range of the African buffalo, but perhaps not in others. With such disruptions, populations are always in a state of transition (and in a form of non-equilibrium dynamics: see for example Ellis and Swift, Reference Ellis and Swift1988; Desta and Coppock, Reference Desta and Coppock2002; Vetter, Reference Vetter2005). Their age structures are thus always changing. Even without further disruptions, it might take more than a couple of decades before a population settles into a stationary age structure.
In this chapter, we have shown the importance of detailed age structure modelling in determining population trajectories. Time series analysis of aggregated populations may lead to significantly incorrect conclusions. This includes, for example, misleading ‘evidence’ for density-dependence for a case where, in fact, there was no such effect at all.
A concern highlighted by this analysis is that in the field it is often difficult to distinguish the exact ages of individuals. This might also have implications for calculating vital rates. Suppose fecundity starts declining from age 15 and we cannot distinguish between 14- and 16-year-olds. Then simply counting calves and adults will not give a specific fecundity rate that will hold for a different mix of 14- and 16-year-olds.
We also have shown that vital rates determine an age structure. If the survival rate, say 0.9, in a particular age group with 100 individuals is known, then the next older age group should comprise 90 individuals a year later. If the population is in equilibrium and hence the age structure is stationary, the older group will contain 90 individuals now. Thus, if numbers in the older group differ from 90 then we know that the population is still in a state of transition.
In conclusion, we contend that understanding the population dynamics of buffalo requires detailed knowledge of the age structure. Furthermore, any data on vital rates should record the extent to which age groups have been aggregated. Any studies on population dynamics using these data should explore the full range of disaggregation possible that is consistent with the aggregated data. Only then can firm statements be made about the population dynamics. Indeed, while it is easy to do some arm-waving about ‘climate change’ and stating that ‘there will be more droughts in Africa’, it is much more difficult to replace comfortable thinking about ‘carrying capacity’ by the realization that animal populations in many parts of Africa are in a state of non-equilibrium dynamics, and that a drought some years ago is still playing havoc with the population dynamics. Nonetheless, choosing the wrong (mathematical) model for predicting future numbers, setting offtake numbers (e.g. for hunting quota) or even temporary destocking strategies may have severe repercussions for the sustainability of a harvesting operation, for example, or for calculating gene flow in a population.