INTRODUCTION
Over several decades, the identification of individuals at high risk for AD has been both a priority and a challenge. Neuropsychology has been at the forefront of this effort (Butters, Delis, & Lucas, Reference Butters, Delis and Lucas1995; Salmon & Bondi, Reference Salmon and Bondi2009; Bondi et al., Reference Bondi, Edmonds and Salmon2017; Han et al., Reference Duke Han, Nguyen, Stricker and Nation2017). The neuropsychological tests that most consistently and accurately predict incident AD are tests of learning and retention (Albert et al., Reference Albert, Moss, Tanzi and Jones2001; Linn et al., Reference Linn, Wolf, Bachman, Knoefel, Cobb, Belanger and D’Agostino1995; Elias et al., Reference Elias, Beiser, Wolf, Au, White and D’Agostino2000; Jacobs et al., Reference Jacobs, Sano, Dooneief, Marder, Bell and Stern1995; Tierney et al., Reference Tierney, Szalai, Snow and Fisher1996; Chen et al., Reference Chen, Ratcliff, Belle, Cauley, DeKosky and Ganguli2001). The earliest studies focused on the inability to learn new information by testing immediate recall of word lists (Miller et al., Reference Miller1971; Weingartner et al, Reference Weingartner, Kaye, Smallberg, Ebert, Gillin and Sitaram1981). Subsequent studies demonstrated that in addition to the hallmark learning deficit, retention tested at delays from 10 to 30 min was also impaired (Larrabee et al., Reference Larrabee, Youngjohn, Sudilovsky and Crook1993; Moss, Albert, Butters, & Payne, Reference Moss, Albert, Butters and Payne1986; Welsh, Butters, Hughes, Mohs, & Heyman, Reference Welsh, Butters, Hughes, Mohs and Heyman1991) In some studies, learning measures outperformed retention measures in predicting incident AD, whereas the reverse was observed in other studies. Nonetheless, both learning and retention deficits are present in preclinical AD (Bondi et al., 1994; Reference Bondi, Salmon, Galasko, Thomas and Thal1999; Grober et al., Reference Grober, Lipton, Hall and Crystal2000).
Previously, we argued that the retention deficit in AD is best examined with memory tests like the Free and Cued selective Reminding test (FCSRT) that control initial encoding in order to obtain maximum learning, the basis for subsequent retention (Grober & Kawas, Reference Grober and Kawas1997). Measuring retention of inadequately learned material can lead to contradictory results as studies of forgetting have shown (e.g., Becker et al., Reference Becker, Boller, Saxton and McGonigle-Gibson1987; Moss et al., Reference Moss, Albert, Butters and Payne1986). AD participants in the preclinical stage recalled significantly fewer words than matched controls indicating an impairment of learning; their retention, measured by percent retained of initial learning, was identical to that of controls. A retention deficit was documented three years later for AD participants but not for controls, whose retention was still perfect. We concluded that a retention deficit was not present in preclinical AD when hallmark learning deficits can be documented (Grober & Kawas, Reference Grober and Kawas1997).
We revisited this issue by examining the trajectories of declines in learning and retention of initially clinically normal participants at baseline in the Baltimore Longitudinal Study of Aging (BLSA) who went on to develop AD dementia over 10 years of follow-up (Grober et al., Reference Grober, Hall, Lipton, Zonderman, Resnick and Kawas2008; Reference Grober, An, Lipton, Kawas and Resnick2019). Learning was defined by the sum of free recall (FR) over the three test trials on the picture version of the test with immediate recall (pFCSRT + IR). Retention was defined by delayed free recall (DFR) tested 15–20 min after learning. Learning and retention displayed similar profiles of decline in the years prior to the clinical diagnosis of AD with a first acceleration of decline (change point) at 6.6–7.3 years prior to diagnosis and a second at 1.9–2.9 years prior to diagnosis. The change points for learning and retention were not significantly different. Retention defined by savings, percent retained of learning, had only one change point at 5.3 years. These analyses included only persons who prospectively developed AD dementia and did not address predictive validity.
In predictive validity studies, the conventional practice has been to rely on retention rather than learning for identifying mild cognitive impairment (MCI) and dementia (Welsh et al., Reference Welsh, Butters, Hughes, Mohs and Heyman1991; Jack et al., Reference Jack, Wiste, Therneau, Weigand, Knopman, Mielke and Petersen2019). In choosing between measures of learning and retention, evidence which favors retention is required, to offset increment in patient and tester burden. We sought to clarify this issue by comparing the predictive validity of learning and retention measures for the identification of BLSA participants who developed incident MCI over 10 years of follow-up. If retention (DFR) adds predictive value beyond learning (FR), that would justify measuring it. Cox proportional hazards models were used to answer these questions.
METHODS
Participants
The primary analyses were based on data from 1422 BLSA participants without MCI at baseline who underwent longitudinal assessments with the pFCSRT + IR between July 1985 and December 2015. At enrollment, BLSA participants meet rigorous screening criteria for health and functional status. All participants had at least one follow-up assessment after baseline. All available visits were included in the analysis. The event being modeled was incident MCI. All analyses were repeated on a subsample of 1283 participants who had Apolipoprotein E (APOE) ϵ4 genotype to determine whether APOE ϵ4 carriers were at increased risk of incident MCI. The BLSA study is approved by the local institutional review board, and all participants gave written informed consent before each assessment.
MCI Diagnosis
Clinical and neuropsychological data from each participant were reviewed at a consensus case conference if their Clinical Dementia Rating score (CDR: Morris, Reference Morris1993) was greater than or equal to .5 or if they made more than three errors on the Blessed Information-Memory-Concentration (BIMC) Test (Blessed et al., Reference Blessed, Tomlinson and Roth1968). MCI was defined using the Petersen criteria (Petersen et al., Reference Petersen, Smith, Waring, Ivnik, Tangalos and Kokmen1999). Diagnoses of dementia and clinical AD were based on criteria outlined in the Diagnostic and Statistical Manual of Mental Disorders, third edition, revised (American Psychiatric Association, 1987) and the National Institute of Neurological and Communication Disorders and Stroke – Alzheimer’s Disease and Related Disorders (McKhann, Drachman, Folstein, et al., Reference McKhann, Drachman, Folstein, Katzman, Price and Stadlan1984). Diagnoses relied on clinical history, informant report, and a broad battery of neurocognitive tests that included pFCSRT + IR scores.
Because knowledge of scores on the pFCSRT + IR may have compromised the test’s independence as a predictor, we performed a sensitivity analysis using an alternative definition of the cognitive impairment defined as four or more errors on the Blessed Information Memory Concentration (BIMC) Test. Persons with incident BIMC scores of 5 to 8 indicate very high risk for incident AD (Katzman et al., Reference Katzman, Aronson, Fuld, Kawas, Brown, Morgenstern and Ooi1989). Analyses were repeated using this alternative end point.
pFCSRT+IR
Before the pFCSRT + IR was administered (Grober and Buschke, Reference Grober and Buschke1987), the 16-line drawings used in the test were presented for naming. The study phase followed in which participants were asked to search a card containing four of the drawings (e.g., grapes) for an item that goes with a unique category cue (e.g., fruit). After all-four items were identified, immediate recall of just those four items was tested by FR followed by cued recall for missed items. When cued recall failed, the participant was told the name of the item. The study phase was repeated for all 16 drawings. The test phase consisted of three trials of FR each followed by cued recall for items not retrieved by FR. The learning measure was the sum of FR (maximum = 48). The retention measure was DFR tested 15–20 min after learning without representation of the items (maximum = 16). Retention defined by the savings method was not used as a predictor because its decline occurred later in the predementia phase and so would not be sensitive to early disease (Grober & Kawas, Reference Grober and Kawas1997; Grober et al., Reference Grober, An, Lipton, Kawas and Resnick2019).
Statistical Analysis
The outcome we modeled was time to incident MCI within 10 years of baseline pFCSRT + IR. Some individuals were never observed to have MCI; they were assessed as normal one assessment and as having dementia the next. For those individuals we assume that the onset of MCI was unobserved and occurred prior to or simultaneously with the onset of dementia. As a proxy for time to MCI in those individuals, we use time to dementia. Cox proportional hazards models were used to evaluate the effect of baseline learning (FR) and retention (DFR) on risk of MCI adjusting for age, sex, and education. The effects of the predictors were reported as hazard ratios, the ratio of the hazard rate of MCI incidence corresponding to 1 unit difference in the predictor for continuous variable, and in the exposed versus the reference group (e.g., APOE ϵ4 carriers versus noncarriers). Because learning and retention scores from the same test are highly correlated (r = 0.65), we sought to determine if each score made an independent contribution to prediction of incident MCI. A sensitivity analysis further adjusting for APOE ϵ4 genotype was performed to evaluate whether APOE ϵ4 genotype (ϵ4 carriers vs. noncarriers) altered the findings. These analyses were repeated using four or more errors on the BIMC as the outcome as a sensitivity analysis. The partial likelihood ratio test (Cox, Reference Cox1972) was used to compare nested models (model with DFR and FR vs FR only, adjusting for covariates). A measure of explained variation, defined as the ratio of distance measures between the survival processes and the fitted survival curves with and without predictors in the model, was also reported (Schemper & Henderson, Reference Schemper and Henderson2000).
RESULTS
Of 1422 participants free of dementia and MCI at baseline, 187 developed MCI over a median of 8.1 years of follow-up (Table 1). The incidence rate was 1.75% per year. Dementia developed in 88 participants. The group that developed MCI was older (77.1 ± 6.8 vs 68.4 ± 7.9 years, Cohen’s d = 1.1, p < .0001), had shorter follow-up time (4.9 ± 2.4 vs 7.5 ± 3.0, Cohen’s d = −0.9, p < .0001), and worse performance on FR (29.5 ± 5.7 vs 32.7 ± 5.0, Cohen’s d = −0.6, p < .0001) and DFR (10.9 ± 2.5 vs 12.1 ± 2.1, Cohen’s d = −0.6, p < .0001), but did not significantly differ in sex (57.8% vs 53.4% men, p = 0.270) or education (16.8 ± 2.7 vs 16.7 ± 2.7 years, Cohen’s d = 0.03, p = 0.494). About 80.3% of the participants were Caucasian, 15.9% were African American, and 3.8% were other races.
Kaplan–Meier curves for incident MCI by FR and DFR are presented in Figure 1 to graphically illustrate our findings. We dichotomized FR using the cutoff 30 (>30 vs <=30) as >30 indicates intact memory in the stages of objective memory impairment (SOMI) system (Grober et al., Reference Grober, Qi, Kuo, Hassenstab, Perrin and Lipton2021a) and dichotomized DFR using cut-off 11 (>11 vs <= 11) which is the lower quantile. In these figures, better performance on FR (HR = 0.32, p < .0001) and DFR (HR = 0.37, p < .0001) showed lower risk of MCI.
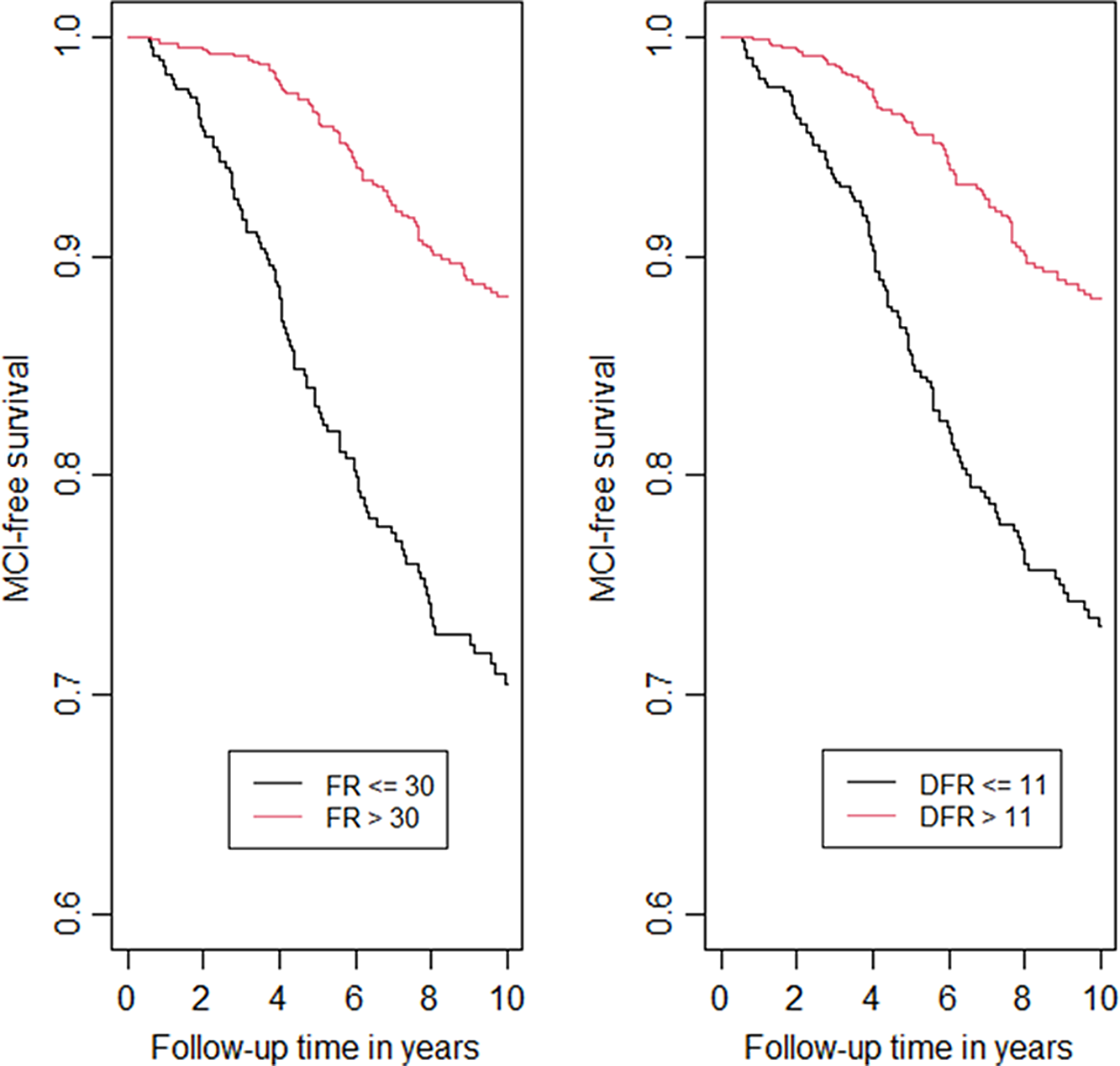
Fig. 1. Kaplan–Meier curves for incident MCI by FR and DFR.
The hazard ratios for incident MCI with using learning (model 1) and retention (model 2) in separate models and in the same analysis (model 3), adjusted for covariates, are shown in Table 2. Age was a significant predictor of incident MCI in all models. Both FR and DFR were significant predictors of incident MCI in separate models: for each SD increase in FR, risk of MCI decreased (HR = 0.66); for each SD increase in DFR, MCI risk also decreased (HR = 0.68). When both FR and DFR were examined simultaneously, both FR (HR = 0.77) and DFR (HR = 0.81) remained significant, and there was no significant difference between the magnitudes of the two effects (p = 0.755). Using the measure of explained variation, the percent explained by FR and covariates is 16.1; the addition of DFR increased the explained variation to 17.0, an increase of 0.9%. For the comparison of adding DFR to the model with FR and covariates, partial likelihood test showed that the addition of DFR significantly improved the model fit (p value = 0.018). The results were not materially different when four or more errors on the BIMC was the outcome event being modeled in 1303 eligible participants with BIMC <= 3 at baseline and follow-up assessment. Using this end point, 261 incident cases developed (Table 3).
Table 1. Baseline characteristics

Table 2. Hazard ratios for incident MCI using baseline FR and DFR as predictors, adjusting for demographic covariates including age at baseline, sex, and education

Model 1: FR on incident MCI adjusting for age, sex, and education; Model 2: DFR on incident MCI adjusting for age, sex, and education; Model 3: FR and DFR on incident MCI adjusting for age, sex, and education.
Table 3. Hazard ratios for incident MCI defined by four or more errors on the BIMC using baseline FR and DFR as predictors, adjusting for demographic covariates including age at baseline, sex and education

Model 1: FR on incident MCI adjusting for age, sex, and education; Model 2: DFR on incident MCI adjusting for age, sex, and education; Model 3: FR and DFR on incident MCI adjusting for age, sex, and education.
Of the 1283 participants who had APOE information, 36% of the incident MCI participants were APOE ϵ4 carriers compared to 25% of the non-cases (p = 0.005). APOE ϵ4 carriers developed incident MCI at more than twice the rate of noncarriers (HR = 2.75). The addition of APOE status as a covariate in the Cox models did not materially change the HRs for FR or DFR for predicting incident MCI (Table 4).
Table 4. Hazard ratios for incident MCI using baseline FR and DFR as predictors, adjusting for age at baseline, sex, education, and APOE ϵ4 carrier status

Model 1: FR on incident MCI adjusting for age, sex, education, and ApoE4 (carriers vs noncarriers); Model 2: DFR on incident MCI adjusting for age, sex, education, and ApoE4; Model 3: FR and DFR on incident MCI adjusting for age, sex, education, and ApoE4.
DISCUSSION
We sought to determine whether the conventional practice of relying on retention rather than learning for identifying MCI was justified when learning and retention were measured with the pFCSRT + IR. We compared their predictive validity for risk of incident MCI among 1422 BLSA participants who were clinically normal at baseline. Totally, 187 participants developed MCI over a median of 8.1 years of follow-up. FR (learning) and DFR (retention) each predicted incident MCI with similar hazard ratios, adjusting for age, sex, and education. When examined simultaneously, both remained significant with similar magnitude of effect: around 20% decrease in the risk of MCI corresponding to 1 SD increase in FR or DFR, confirming the predictive value of both learning and retention measures. The addition of DFR to the model including FR and covariates increased the explained variation by 0.9% and significantly increased the partial likelihood. Age was a significant predictor in all models, whereas sex and years of education were not significant predictors in any model. Analyses were repeated with four or more errors on the BIMC as the end point as a sensitivity analysis to avoid diagnostic circularity given inclusion of pFCSRT + IR in diagnostic consensus conferences. The results were not materially different. APOE ϵ4 carriers developed incident MCI at rates in line with published studies (Dang et al., Reference Dang, Harrington, Lim, Ames, Hassenstab, Laws and Maruff2018). Importantly, adjusting for APOE ϵ4 allele did not diminish the relationship between FR or DFR and incident MCI.
The incidence rate for MCI was 1.75% per year which is low in comparison to the incidence rate in other longitudinal studies (Kantarci et al., Reference Kantarci, Weigand, Przybelski, Preboske, Pankratz, Vemuri and Jack2013; Machulda et al., Reference Machulda, Pankratz, Christianson, Ivnik, Mielke, Roberts and Petersen2013). The low incidence rate most likely reflects the strict health and functional criteria at BLSA enrollment and the continuous enrollment of BLSA participants.
The FCSRT has been widely used to identify prevalent dementia, incident dementia and AD, and MCI (Auriacombe et al., Reference Auriacombe, Helmer, Amieva, Berr, Dubois and Dartigues2010; Di Stefano et al., Reference Di Stefano, Epelbaum, Coley, Cantet, Ousset, Hampel and Andrieu2015; Derby et al., Reference Derby, Burns, Wang, Katz, Zimmerman, L’Italien and Lipton2013; Katz et al., Reference Katz, Lipton, Hall, Zimmerman, Sanders, Verghese and Derby2012; Sarazin et al., Reference Sarazin, Berr, De Rotrou, Fabrigoule, Pasquier, Legrain and Dubois2007). pFCSRT + IR measures, specifically FR, total recall (TR; sum of FR and cued recall) and their combination (FR + TR) are components in the preclinical Alzheimer’s disease clinical composite (PACC) for detecting cognitive change that also includes Logical Memory, Digit Symbol Substitution Test and the Mini Mental State Exam (Donohue et al., Reference Donohue, Sperling, Salmon, Rentz, Raman, Thomas and Aisen2014; Papp et al., Reference Papp, Rentz, Mormino, Schultz, Amariglio, Quiroz and Sperling2017). When the PACC was administered annually to 277 clinically normal participants in the Harvard Aging Brain Study (HABS) grouped according to threshold levels of amyloid imaging and followed for up to 5 years (Mormino et al., Reference Mormino, Papp, Rentz, Donohue, Amariglio, Quiroz and Sperling2017), all combinations including FR resulted in larger magnitude of effect for differences between Aβ groups over three and five years of follow-up than any other PACC component. FR alone or combined with total recall was the only individual component to show differences between the Aβ+ group who progressed to CDR 0.5 versus those that remained stable.
The failure of clinical trials targeted to decreasing the accumulation of Aβ pathology in cognitively normal adults prompted examination of cognitive decline that occurs within the normal range of the amyloid imaging tracer, 18F-florbetapir (Insel et al., Reference Insel, Donohue, Sperling, Hansson and Mattsson-Carlgren2020). Continuous levels of the tracer were associated with the individual PACC components of 4432 cognitively unimpaired adults screened for inclusion in the A4 trial (Sperling et al., Reference Sperling, Rentz, Johnson, Karlawish, Donohue, Salmon and Aisen2014). The magnitude of the decrease in FR and FR + TR scores at subclinical levels of tracer uptake, standard uptake volume ratio (SUVR = 1.10) compared to normal levels (SUVR = 0.78) was more than twice that of the other PACC components with a larger magnitude of effect than the PACC itself. Though the decline in pFCSRT + IR performance in the subthreshold range of Aβ was small, it marks the start of episodic memory impairment that is the hallmark of AD.
The decline of FR in the preclinical course of AD was associated with the progression of neurofibrillary tangle (NFT) pathology defined by Braak stage in 300+ cases from Washington University clinic-neuropathologic cohort (Grober et al., Reference Grober, Qi, Kuo, Hassenstab, Perrin and Lipton2021b). Compared with cases with limited NFT pathology (Braak stage 0 and I), FR of cases with Braak stage III pathology was significantly lower and continued to decline at similar rates in successive Braak stages. Unlike FR, Mini Mental State Exam and CDR sum of boxes scores did not decline until Braak stage IV. We suggest that FR performance may be useful in predicting tau positivity in observational studies and in clinical trials (Grober et al., Reference Grober, Qi, Kuo, Hassenstab, Perrin and Lipton2021b).
Other studies have compared measures of learning and retention as predictors of incident AD, with varying results. Differences in the patterns of results can be observed for the same test. When the California Verbal Learning Test (CVLT) measures were used to predict incident dementia in 133 participants without dementia at baseline, neither the short nor long delay measures improved prediction over learning, as measured by the sum of FR over five trials (Bondi et al., Reference Bondi, Salmon, Galasko, Thomas and Thal1999). In another comparison of CVLT measures in predicting MCI or dementia, learning was the most powerful predictor of all the measures, but predictive value was enhanced by adding delayed story recall (Rabin et al., Reference Rabin, Paré, Saykin, Brown, Wishart, Flashman and Santulli2009). The varying results even for the same test are not surprising when the factors that determine predictive value are considered: the stage of an individual with respect to the multiyear process of cognitive decline that precedes dementia (Bilgel et al., Reference Bilgel, An, Lang, Prince, Ferrucci, Jedynak and Resnick2014); the psychometric properties of the particular test being used (Grober, Ocepek-Welikson, & Teresi, Reference Grober, Ocepek-Welikson and Teresi2009); and the composition of the sample that does not go on to develop dementia.
The most recent example of neuropsychology’s contribution to the identification of individuals at high risk of AD examined trajectories of 35 neuropsychological tests in an APOE-ϵ4-enriched cohort of 784 cognitively normal participants to determine how far in advance of incident MCI cognitive decline can be identified (Caselli et al., Reference Caselli, Langlais, Dueck, Chen, Su, Locke and Reiman2020). Sixty-five participants developed amnestic MCI during an average follow-up of 9.5 years at mean age of 73. The rate of decline of 34 of the 35 tests was steeper among MCI converters relative to nonconverters following the inflection point when performance of the two groups diverged. Multiple episodic memory tests (Auditory Verbal Learning Test and Selective Reminding Test) displayed the earliest inflection points, nearly 20 years in advance of MCI diagnosis, with retention decline beginning a year earlier than learning decline (age 54 versus 55). These findings challenge the current disease model of preclinical AD wherein cognition begins to decline after sufficient amyloid and tau deposition has occurred (Sperling et al., Reference Sperling, Aisen, Beckett, Bennett, Craft, Fagan and Phelps2011; Jack et al., Reference Jack, Knopman, Jagust, Petersen, Weiner, Aisen and Trojanowski2013).
The strength of our data set is the sizable and well-characterized cohort of incident MCI cases and the large number of assessments available over more than 20 years of follow-up. However, generalizability is limited due to the high educational level of the BLSA cohort. The similarity of the findings based on clinical conference diagnosis, where circularity is possible, and an outcome based on a BIMC cut point for impairment, adds confidence that our findings are not an artifact of our diagnostic procedures.
The benefits of testing retention after initial learning may depend on the particular memory test being used and the stage of disease. Our results using the pFCSRT + IR suggest that the practice of preferring retention over learning to predict incident MCI merits reconsideration since both independently predict the outcome in the presence of the other with a similar magnitude of effect. Adding retention to the model that included learning increased the explained variation by about 1%. Thus, the decision to include DFR in the assessment may depend on the setting. In the clinic, if there is time to extend the assessment by 20 min to capture DFR, the additional information may be warranted. In a telephone or web-based assessment where time may be more limited and patient burden a greater concern, adding DFR may be inadvisable given its marginal enhancement in predicting MCI.
Funding Statement
This study was supported in part by the Intramural Research Program, National Institute on Aging, NIH and the NIH: 2PO1 AG003949.
Conflicts of Interest
The FCSRT + IR is copyrighted by the Albert Einstein College of Medicine and is made freely available for noncommercial purposes. Dr. Ellen Grober receives a small percentage of any royalties on the FCSRT + IR when it is used for commercial purposes.
Dr. Cuiling has no disclosures. Dr. Susan Resnick has no disclosures other than being an employee of the NIA. Dr. Claudia Kawas has no disclosures. Dr. Melissa Kitner-Triolo has no disclosures.
Dr. Richard B. Lipton is the Edwin S. Lowe Professor of Neurology at the Albert Einstein College of Medicine in New York. He receives research support from the NIH: 2PO1 AG003949 (mPI), 5U10 NS077308 (PI), R21 AG056920 (Investigator), 1RF1 AG057531 (Site PI), RF1 AG054548 (Investigator), 1RO1 AG048642 (Investigator), R56 AG057548 (Investigator), U01062370 (Investigator), RO1 AG060933 (Investigator), K23 NS09610 (Mentor), K23AG049466 (Mentor), K23 NS107643 (Mentor). He also receives support from the Migraine Research Foundation and the National Headache Foundation. He serves on the editorial board of Neurology, senior advisor to Headache, and associate editor to Cephalalgia. He has reviewed for the NIA and NINDS, holds stock options in eNeura Therapeutics and Biohaven Holdings; serves as consultant, advisory board member, or has received honoraria from: American Academy of Neurology, Alder, Allergan, American Headache Society, Amgen, Avanir, Biohaven, Biovision, Boston Scientific, Dr. Reddy’s, Electrocore, Eli Lilly, eNeura Therapeutics, GlaxoSmithKline, Merck, Pernix, Pfizer, Supernus, Teva, Trigemina, Vector, Vedanta. He receives royalties from Wolff’s Headache 7th and 8th Edition, Oxford Press University, 2009, Wiley and Informa.