Introduction
Post-traumatic stress disorder (PTSD) is a prevalent psychiatric disorder that occurs in some individuals after a traumatic event (Duncan et al., Reference Duncan, Ratanatharathorn, Aiello, Almli, Amstadter, Ashley-Koch and Koenen2018). PTSD poses strong social impacts: suicide, hospitalization, and substance use (Davidson, Reference Davidson2000). Although scholars have now begun to prioritize PTSD in ameliorating various social burdens, fundamental questions remain on the genetic etiology of PTSD.
Gastrointestinal tract (GIT) disorders frequently co-occur with psychiatric diagnoses marked by irritability, fearfulness, hypervigilance, and physiological mobilization (Kolacz, Kovacic, & Porges, Reference Kolacz, Kovacic and Porges2019). GIT-psychiatric comorbidities emerge in a range of psychiatric disorders: PTSD, anxiety, and depression (Graff, Walker, & Bernstein, Reference Graff, Walker and Bernstein2009; Grinsvall, Tornblom, Tack, Van Oudenhove, & Simren, Reference Grinsvall, Tornblom, Tack, Van Oudenhove and Simren2018; Hejazi & McCallum, Reference Hejazi and McCallum2014; Henningsen, Zimmermann, & Sattel, Reference Henningsen, Zimmermann and Sattel2003). Growing evidence suggest traumatic experiences are related to GIT disorders: a history of abuse exacerbates symptoms of irritable bowel disease (IBD) (Drossman et al., Reference Drossman, Tack, Ford, Szigethy, Törnblom and Van Oudenhove2018; Leserman, Reference Leserman2005); acute early-life stressors induce long-term sensory and motor digestive changes in irritable bowel syndrome (IBS) (O'Mahony, Hyland, Dinan, & Cryan, Reference O'Mahony, Hyland, Dinan and Cryan2011; Vannucchi & Evangelista, Reference Vannucchi and Evangelista2018). However, whether GIT disorders are a risk factor for PTSD and vice versa are unclear.
Large-scale genome-wide association studies (GWASs) have documented a growing number of single-nucleotide polymorphisms (SNPs), genes, and susceptibility loci respectively for PTSD and GIT disorders (An et al., Reference An, Gharahkhani, Law, Ong, Han, Olsen and MacGregor2019; Nievergelt et al., Reference Nievergelt, Maihofer, Klengel, Atkinson, Chen, Choi and Koenen2019; Watanabe et al., Reference Watanabe, Stringer, Frei, Umicevic Mirkov, de Leeuw, Polderman and Posthuma2019; Wu et al., Reference Wu, Murray, Byrne, Sidorenko, Visscher and Wray2021), providing a basis to evaluate the genetic etiology of PTSD and GIT disorders including gastroesophageal reflux disease (GORD), peptic ulcer disease (PUD), PUD and/or GORD and/or medications (PGM, an alternative combination of diagnosis and/or treatments for PUD and/or GORD), IBS, and IBD. Nevertheless, no study has reported their shared genetic architectures.
Here, we performed a comprehensive genome-wide cross-trait analysis to investigate the genetic overlaps and the causal relationships between PTSD and GIT disorders. We quantified the global and local genetic correlations, analyzed partitioned heritability and cell-type-specific enrichment, identified pleiotropic loci, performed functional annotations, conducted gene-level analyses, and inferred putative causal associations.
Methods
GWAS summary statistics
Overall study design is shown in Fig. 1. GWAS data are summarized in online Supplementary Table S1. We obtained GWAS summary statistics for PTSD from the Psychiatric Genomics Consortium (PGC) comprising 23 212 cases and 151 447 controls of European ancestry (Nievergelt et al., Reference Nievergelt, Maihofer, Klengel, Atkinson, Chen, Choi and Koenen2019). In the original study, PTSD assessment was based on lifetime or current PTSD, and the unaffected control individuals did not have a PTSD diagnosis; PTSD was diagnosed using various instruments and different versions of the Diagnostic and Statistical Manual of Mental Disorders; quality control was performed by PGC pipeline and dataset was imputed by the 1000 Genomes European panel. We obtained GWAS for GIT traits including PUD (16 666 cases and 439 661 controls), GORD (54 854 cases and 401 473 controls), IBS (28 518 cases and 426 803 controls), and IBD (7045 cases and 449 282 controls) from a recent publication (Wu et al., Reference Wu, Murray, Byrne, Sidorenko, Visscher and Wray2021). Clinically, PUD medications are indicated in GORD and gastritis. Accordingly, GWAS combining diagnosis for PGM commonly used for these disorders was conducted, and we used this GWAS data (90 175 cases and 366 152 controls) as a proxy for PUD or GORD (Wu et al., Reference Wu, Murray, Byrne, Sidorenko, Visscher and Wray2021). The criteria for GIT disorders are: diagnosis phenotypes from death register, self-report, hospital admission, or primary care record; treatment phenotypes based on the operation and medication-taking code. Dataset was imputed to a mixed panel of UK10K+1000 Genomes and Haplotype Reference Consortium (Bycroft et al., Reference Bycroft, Freeman, Petkova, Band, Elliott, Sharp and Marchini2018).

Figure 1. Overall study design of genome-wide cross-trait analysis.
Genetic correlation
We conducted a post-GWAS global correlation analysis by linkage disequilibrium (LD) score regression (LDSC) (Bulik-Sullivan et al., Reference Bulik-Sullivan, Finucane, Anttila, Gusev, Day, Loh and Neale2015). LDSC estimates correlation between true causal effects of two traits (ranging from −1 to 1). SNPs in a high LD region would have a larger χ2, and a similar relationship would appear when single-study statistics are replaced by the product of z-scores from two studies of traits with correlation. We performed LDSC with unconstrained intercept using pre-computed LD scores from the 1000 Genomes Project Phase 3 (European ancestry), and restricted our analysis to only haplotype map 3 (HapMap3) SNPs to minimize bias of low imputation quality (Bulik-Sullivan et al., Reference Bulik-Sullivan, Finucane, Anttila, Gusev, Day, Loh and Neale2015). We set Bonferroni-corrected significant threshold at a p value of 0.01 (0.05/5 = 0.01).
We calculated pairwise local correlations for PTSD and GIT disorders in 2343 pre-specified LD-independent segments using the SUPER GeNetic cOVariance Analyzer (SUPERGNOVA), which identifies locally associated small contiguous genomic regions using GWAS summary statistics and a reference panel as input data, and provides p values (P SUPERGNOVA) between pairs of traits in local regions (Zhang et al., Reference Zhang, Lu, Ye, Huang, Liu, Wu and Zhao2021). Here, we removed SNPs with missing values, used the 1000 Genomes Project, with rare variants (minor allele frequency < 5%) filtered out, as the reference panel to generate the genome partition files in SUPERGNOVA. P SUPERGNOVA < 1 × 10−4 was used as a cut-off value to indicate significance (Chen, Wang, Huang, & Jia, Reference Chen, Wang, Huang and Jia2022).
SNP heritability and cell-type-specific enrichment
We partitioned SNP heritability of PTSD and GIT disorders respectively by stratified LDSC, to determine if SNPs sharing high heritability are clustered in the 24 main functional annotations (Finucane et al., Reference Finucane, Bulik-Sullivan, Gusev, Trynka, Reshef, Loh and Price2015). We retrieved LD scores, regression weights, and allele frequencies from European samples (https://alkesgroup.broadinstitute.org/LDSCORE). We assumed the estimates to be significant if p value surpasses an α level of 0.002, derived by Bonferroni correction.
We performed heritability partitioning for the 396 annotations constructed by the Roadmap project for six chromatin marks (‘DHS’, ‘H3K27ac’, ‘H3K36me3’, ‘H3K4me1’, ‘H3K4me3’, ‘H3K9ac’) in a set of 88 primary cell types or tissues (Finucane et al., Reference Finucane, Reshef, Anttila, Slowikowski, Gusev, Byrnes and Price2018). Each annotation corresponds to a chromatin mark in a single-cell type. These 396 cell-type-specific annotations belong to nine groups: adipose, central nervous system, digestive system, cardiovascular, musculoskeletal, and connective tissue, immune and blood, liver, pancreas, and other. We calculated annotation-specific enrichment values for each trait using LDSC, and performed visualization by hierarchical clustering. We used adjusted p value to correct for multiple testing.
Cross-trait meta-analysis
Multi-trait analysis of GWAS (MTAG) applies generalized inverse-variance-weighted meta-analysis for multiple correlated traits, and detects genetic associations for each trait by borrowing correlations among correlated traits to boost statistical power (Guo et al., Reference Guo, Gong, Li, Liu, Yan, Wang and Yuan2022; Tadros et al., Reference Tadros, Francis, Xu, Vermeer, Harper, Huurman and Bezzina2021; Yang et al., Reference Yang, Musco, Simpson-Yap, Zhu, Wang, Lin and Zhou2021). We performed MTAG and implemented options that assume equal SNP heritability for each trait (Turley et al., Reference Turley, Walters, Maghzian, Okbay, Lee, Fontana and Benjamin2018). We denoted the single-trait GWAS statistics as GWASPTSD, GWASPUD, GWASGORD, GWASPGM, GWASIBS, and GWASIBD, respectively, and the PTSD statistics from MTAG analysis as MTAGPTSD. Using functional mapping and annotation (FUMA) platform (Watanabe, Taskesen, van Bochoven, & Posthuma, Reference Watanabe, Taskesen, van Bochoven and Posthuma2017), we identified genome-wide significant SNPs of MTAGPTSD (p < 5.0 × 10−8; pairwise R 2 < 0.6 in a 1 Mb window), and conducted gene-set and tissue expression analyses by multi-marker analysis of genomic annotation (MAGMA v.1.08, implemented in FUMA). For gene-set analysis, we used gene ontology (GO) gene sets from the Molecular Signatures Database (MSigDB, v.6.2). For tissue analysis, we used data from Genotype-Tissue Expression project (GTEx, v.8). For comparison, we additionally performed annotations of the significant SNPs identified from GWASPTSD using the FUMA platform.
To determine if assumption violations biased the MTAG results, we performed pleiotropic analysis under composite null hypothesis (PLACO) as a sensitivity analysis (Ray & Chatterjee, Reference Ray and Chatterjee2020). PLACO identifies pleiotropic loci by testing the composite null hypothesis that a locus is associated with zero or one of the traits (Ray et al., Reference Ray, Venkataraghavan, Zhang, Leslie, Hetmanski, Weinberg and Beaty2021). We prioritized independent SNPs with genome-wide significance in both MTAG and PLACO (P PLACO < 5 × 10−8, and trait-specific P MTAG for PTSD < 1 × 10−5), following identification by LD clumping (r 2 < 0.2 within 500-kilobase windows) in ‘PLINK v1.9’ (https://www.cog-genomics.org/plink/1.9), based on 1000 Genomes reference data (Purcell et al., Reference Purcell, Neale, Todd-Brown, Thomas, Ferreira, Bender and Sham2007).
Functional annotation
To annotate functions of the identified pleiotropic SNPs, we used Ensembl variant effect predictor and human variation annotation database (http://www.licpathway.net/VARAdb) (Pan et al., Reference Pan, Liu, Bai, Han, Jiang, Ai and Li2021; Zerbino et al., Reference Zerbino, Achuthan, Akanni, Amode, Barrell, Bhai and Flicek2018). We then queried these loci in the GWAS catalogue (https://www.ebi.ac.uk/gwas) to determine their identity in PTSD and GIT disorders.
We assessed GO biological process functional categories and Kyoto encyclopedia of genes and genomes pathways for enriched pleiotropic SNPs between PTSD and five GIT traits. We performed tissue enrichment using ‘GENE2FUNC’ function in the FUMA platform with 30 general tissue types from GTEx v.8 (GTEx Consortium et al., 2017; Watanabe et al., Reference Watanabe, Taskesen, van Bochoven and Posthuma2017). We applied Benjamini–Hochberg procedure to correct for multiple testing.
Gene-level analysis
Using methods with different assumptions to obtain overlapped signals can avoid the risk of false discoveries. Thus, we applied three parallel gene-level analyses with distinct principles: MAGMA, genome-wide complex trait analysis-fast set-based association analysis (GCTA-fastBAT), and transcriptome-wide association study (TWAS) analysis.
MAGMA uses a multiple regression structure to allow generalized analysis of continuous properties of genes and simultaneous analysis of multiple gene-sets and other gene properties (de Leeuw, Mooij, Heskes, & Posthuma, Reference de Leeuw, Mooij, Heskes and Posthuma2015). Here, we submitted summary statistics for MTAGPTSD in MAGMA to analyze all protein-coding genes to which at least one SNP is annotated within a 0 kb interval. We applied Bonferroni correction for multiple testing based on the number of genes tested (P MAGMA = 2.66 × 10−6).
GCTA-fastBAT analyzes human complex traits using summary-level data from GWAS and LD data from a reference sample with individual-level genotypes (Bakshi et al., Reference Bakshi, Zhu, Vinkhuyzen, Hill, McRae, Visscher and Yang2016). Here, we conducted a gene-based analysis by GCTA-fastBAT using MTAGPTSD for all 24 763 genes and LD information from 1000 Genomes Project Phase 3. We only analyzed the SNPs located within the gene to examine the gene–trait associations. We set Bonferroni-corrected significance at P fastBAT < 2.02 × 10−6 (0.05/24 763).
TWAS integrates GWAS and expression quantitative trait locus (eQTL) data to identify tissue-specific gene–trait associations (Hu et al., Reference Hu, Li, Lu, Weng, Wang, Zekavat and Zhao2019). We used MTAGPTSD and the unified test for molecular signatures (UTMOST) program combined with generalized Berk–Jones (GBJ) models in a two-stage TWAS analysis (https://github.com/Joker-Jerome/UTMOST). We used GBJ models with transcriptome data derived from 44 different tissues (GTEx, v.6). We set Bonferroni-corrected significance at P TWAS < 3.24 × 10−6 (0.05/15 430).
Causal inference
We conducted Mendelian randomization (MR) analysis with R packages ‘TwoSampleMR’ and ‘MR-PRESSO’. We used five MR methods: inverse-variance weighted (IVW) (Burgess, Butterworth, & Thompson, Reference Burgess, Butterworth and Thompson2013), MR–Egger (Burgess & Thompson, Reference Burgess and Thompson2017), weighted median (Bowden, Davey Smith, Haycock, & Burgess, Reference Bowden, Davey Smith, Haycock and Burgess2016), weighted mode (Hartwig, Davey Smith, & Bowden, Reference Hartwig, Davey Smith and Bowden2017), and MR-PRESSO (Verbanck, Chen, Neale, & Do, Reference Verbanck, Chen, Neale and Do2018), with different assumptions in the extent and nature of horizontal pleiotropy. We applied the IVW method as our primary approach (Burgess, Scott, Timpson, Davey Smith, & Thompson, Reference Burgess, Scott, Timpson, Davey Smith and Thompson2015), and we considered relationships with consistent evidence of causality in all MR methods to be more reliable and noteworthy.
IVW assumes if an uncorrelated pleiotropy exists, a mean of zero adds noise only to regressions with multiplicative random effects. MR–Egger allows the presence of directional (i.e. non-zero mean) uncorrelated pleiotropy, and adds an intercept to the IVW regression to exclude confounding. Weighted median measures the median rather than the mean of SNP ratio, and identifies true causality if ≤50% of the weights are from invalid SNPs. Weighted model divides SNPs into groups based on estimated effects, assesses evidence of causality using only the group with the most SNPs, essentially relaxing the hypothesis, and identifies true effects even if most tools are invalid. In these MR analyses, we used independent SNPs (LD clumping r 2 < 0.05 within 1000-kb windows using PLINK v1.9) associated with the ‘exposure’ trait (p < 1 × 10−5) as instrumental variables, and merged with SNPs from the ‘outcome’ trait in Harmonizing data step. To avoid sample overlapping effect, we used the function ‘power_prune’ from R package ‘TwoSampleMR’ in Harmonizing data step.
Results
Genetic correlations
With unconstrained intercept, we found positive global correlations of PTSD with PUD (r g = 0.5261, p = 9.3549 × 10−7), GORD (r g = 0.3977, p = 5.2225 × 10−9), PGM (r g = 0.5236, p = 1.2506 × 10−15), IBS (r g = 0.4185, p = 8.8245 × 10−6), and a marginal correlation with IBD (r g = 0.179, p = 0.0558) (Table 1). We identified no statistically significant local correlation of PTSD with GIT disorders in specific genomic regions (online Supplementary Data files S1–S5).
Table 1. Genome-wide global correlations between PTSD and GIT disorders by unconstrained LD score regression

PTSD, post-traumatic stress disorder; PUD, peptic ulcer disease; PGM, PUD and/or GORD and/or medications; GORD, gastroesophageal reflux disease; IBS, irritable bowel syndrome; IBD, inflammatory bowel disease; r g, genetic correlation, se, standard error; GIT, gastrointestinal tract.
Partitioned SNP heritability and cell-type-specific enrichment
In partitioned SNP heritability analysis, we found significant enrichments of the functional categories ‘conserved’, ‘FetalDHS’, ‘H3K27ac’, ‘H3K4me1’, ‘H3K4me3’, ‘Intron’, ‘SuperEnhancer’, ‘TSS’, and ‘WeakEnhancer’ for PGM, ‘conserved’ for GORD and IBS, and ‘Enhancer’, ‘super Enhancer’, ‘TFBS’, and ‘H3K27ac’ for IBD. We observed no functional categories enrichment for PUD and PTSD (online Supplementary Table S2).
Partitioning SNP heritability using 396 cell-type-specific annotations, we found significant enrichments for GORD and IBS in the central nervous and digestive systems: brain dorsolateral prefrontal cortex, brain inferior temporal lobe, and fetal stomach. We observed significant enrichments for IBD in the blood and immune system: primary natural killer cells and primary T helper 17 cells. We found insignificant enrichments for PUD, while enrichments are clustered closely to GORD/IBS at each chromatin mark in the central nervous and digestive systems. Comparing enrichments for all GIT disorders, we observed differential patterns, where only IBD is enriched in the immune system (online Supplementary Data files S6–S11).
Cross-trait meta-analysis and pleiotropic loci
In meta-analysis of GWASPTSD, GWASPUD, GWASGORD, GWASPGM, GWASIBS, and GWASIBD using MTAG, we obtained a total of 6 443 162 SNPs, and we identified 541 genome-wide significant SNPs in MTAGPTSD with FUMA annotations (online Supplementary Data file S12). The Manhattan plots for GWASPTSD and MTAGPTSD are shown in online Supplementary Fig. S1. We found the number of risk loci increases from two in GWASPTSD to seven in MTAGPTSD, and the number of lead SNPs (P MTAG < 5 × 10−8 and R 2 < 0.1) increases from two in GWASPTSD to eight in MTAGPTSD (online Supplementary Tables S3 and S4). In MAGMA analysis for MTAGPTSD, we identified significant gene-sets linked to regulation of biosynthetic process and regulation of gene expression function, and we associated MTAGPTSD expressions in brain tissues (brain frontal cortex BA9, brain anterior cingulate cortex BA24, brain cortex, brain cerebellar hemisphere, brain cerebellum, brain hypothalamus) (online Supplementary Data files S13 and S14).
Using both MTAG and PLACO, we identified seven loci with a genome-wide significance in the meta-analysis of PTSD and PGM (rs13107325, rs1632855, rs1800628, rs2188100, rs3129953, rs6973700, and rs73154693); three in the meta-analysis of PTSD and GORD (rs13107325, rs1632855, and rs3132450); and one in the meta-analysis of PTSD and IBS/IBD (rs4937872 and rs114969413, respectively) (online Supplementary Tables S5 and S6). We observed no genome-wide significant locus in the meta-analysis of PTSD and PUD. We queried each locus for pleiotropic associations with other traits via GWAS catalogue, and identified proximal genes: SLC39A8, MUC21, MUC22, TNF, LTB, FAM209A/FAM209B, and PRRC2A (online Supplementary Tables S7 and S8). In functional and tissue enrichment analysis of proximal genes, we found that PTSD shares ‘immune response related regulation’ with PGM and IBD, and ‘protein processing’ and ‘regulation of acute inflammatory response’ with IBD (online Supplementary Tables S9 and S10). Using GTEx data, we found significant enriched expression of PTSD- and PGM-shared genes in blood, and PTSD- and IBD-shared genes in brain and liver (online Supplementary Figs S2 and S3). We found no statistically significant enrichment for other traits.
Gene-level analysis
In MAGMA, we observed 35 PTSD-related genes that survive correction for multiple testing; in GCTA-fastBAT, we found 93 genes at the significant level (P fastBAT < 2.02 × 10−6); in TWAS combining eQTL data from 44 tissues, we revealed 31 genes with Bonferroni-corrected significance (online Supplementary Tables S11–S13).
We checked the overlapping genes identified by MAGMA, GCTA-fastBAT, and TWAS, and identified five PTSD-associated candidates: ABT1 (P MAGMA = 7.76 × 10−7, P fastBAT = 1.38 × 10−8, P TWAS = 1.57 × 10−6), BTN3A2 (P MAGMA = 2.60 × 10−8, P fastBAT = 7.52 × 10−10, P TWAS = 9.94 × 10−8), HIST1H3J (P MAGMA = 1.25 × 10−8, P fastBAT = 1.15 × 10−6, P TWAS = 1.87 × 10−8), ZKSCAN4 (P MAGMA = 4.22 × 10−7, P fastBAT = 8.89 × 10−8, P TWAS = 2.95 × 10−12), and ZKSCAN8 (P MAGMA = 4.72 × 10−8, P fastBAT = 2.60 × 10−8, P TWAS = 5.71 × 10−7). For the proximal genes of pleiotropic loci identified by our cross-trait meta-analysis, we found PRRC2A (MAGMA and GCTA-fastBAT), MUC21 and MUC22 (GCTA-fastBAT) are significant in our gene-level analysis.
Causal associations
In IVW MR analysis, we found significant causal effects of PGM, GORD, IBS, and IBD on PTSD (Fig. 2), estimates remain directionally consistent in MR–Egger and weighted median despite larger statistical uncertainties, and MR-PRESSO sensitivity analysis supports a causal association of four GIT disorders with PTSD (online Supplementary Table S14). In reverse-direction MR analysis, we found no statistically significant causal effects of PTSD on GIT disorders, except for GORD (Fig. 3 and online Supplementary Table S14).

Figure 2. MR analyses for causal effects of GIT disorders on PTSD. Boxes represent the point estimates of causal effects, and error bars represent 95% confidence intervals. Inverse-variance-weighted approach was adopted as the primary analysis. PTSD, post-traumatic stress disorder; PUD, peptic ulcer disease; PGM, PUD and/or GORD and/or medications; GORD, gastroesophageal reflux disease; IBS, irritable bowel syndrome; IBD, inflammatory bowel disease; GIT, gastrointestinal tract.
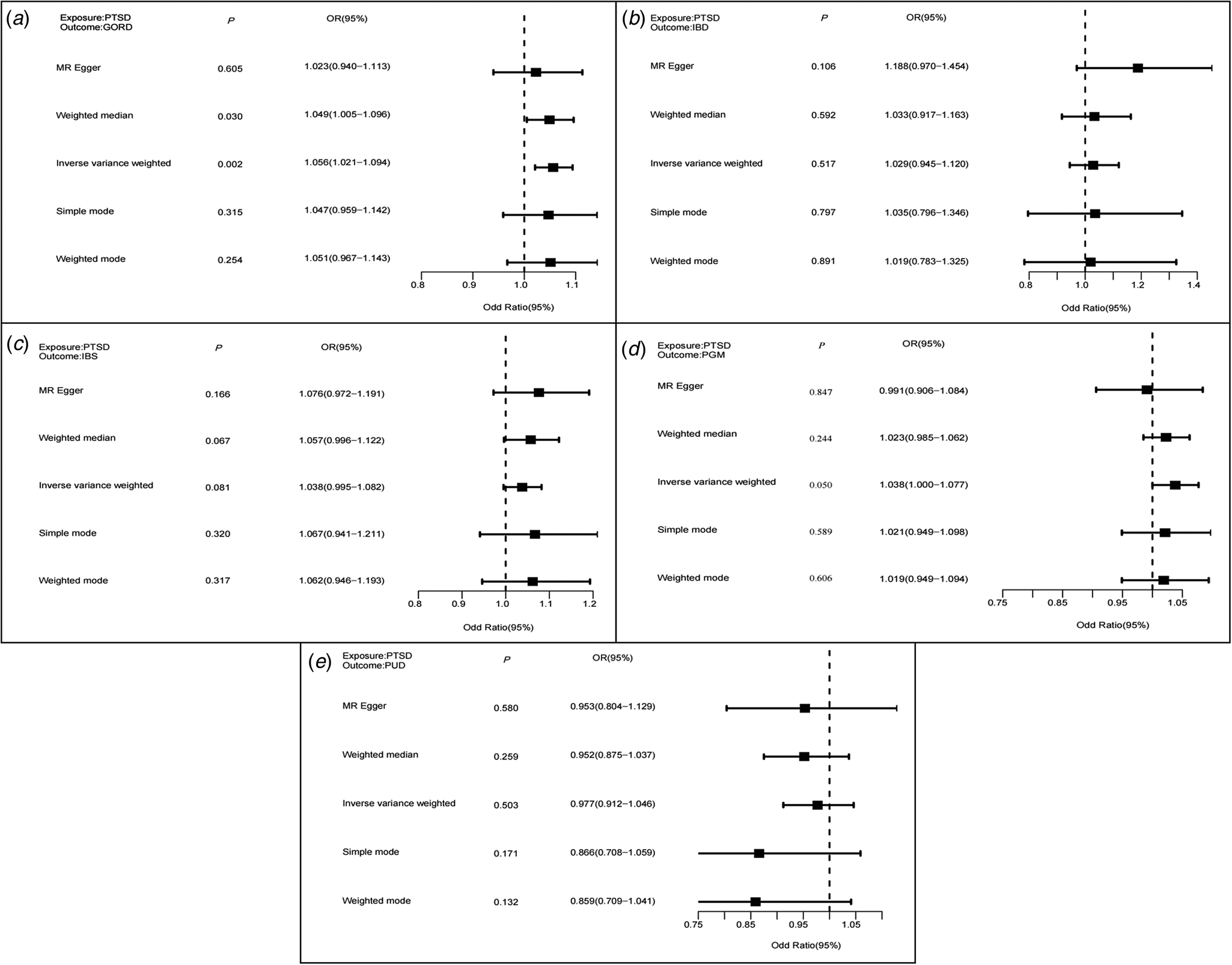
Figure 3. MR analyses for causal effects of PTSD on GIT disorders. Boxes represent the point estimates of causal effects, and error bars represent 95% confidence intervals. Inverse-variance-weighted approach was adopted as the primary analysis. PTSD, post-traumatic stress disorder; PUD, peptic ulcer disease; PGM, PUD and/or GORD and/or medications; GORD, gastroesophageal reflux disease; IBS, irritable bowel syndrome; IBD, inflammatory bowel disease; GIT, gastrointestinal tract.
Discussion
With large-scale GWAS summary data and multiple statistical approaches, we present a comprehensive assessment of shared genetic architecture between PTSD and GIT disorders. We identified significant genetic correlations between all five GIT disorders with PTSD in global correlation analysis, and we did not find a local correlation at statistical significance, suggesting that current analyses are underpowered to detect correlation in specific genomic regions between PTSD and GIT disorders. Significant genetic correlation reflects not only a common genetic etiology (biological pleiotropy), but also suggests a potential causal effect (vertical pleiotropy). In agreement, we observed causal associations of four GIT disorders (IBD, IBS, PGM, and GORD) with PTSD using IVW and MR-PRESSO, and we found the estimates remain directionally consistent in MR–Egger and weighted median approaches.
In the cross-trait meta-analysis of PTSD, we found a genome-wide significant locus rs13107325 for both PGM and GORD. This independent SNP is mapped to the gene SLC39A8, encoding a metal cation transporter Zrt-/Irt-related protein (ZIP8) in all vertebrates. Missense mutation in SLC39A8 leads to reduced zinc transport, which in turn perturbs electrophysiological recordings of neurons, resulting in a significant reduction in spontaneous excitatory postsynaptic currents and reduced surface expression of glutamate receptors (Kalappa, Anderson, Goldberg, Lippard, & Tzounopoulos, Reference Kalappa, Anderson, Goldberg, Lippard and Tzounopoulos2015; Tseng et al., Reference Tseng, Reinhart, Lanz, Weber, Pang, Le and Buhl2021). A recent review recognizes SLC39A8 exhibits significant pleiotropic effects associated with clinical diseases in virtually every organ, tissue, and cell type (Nebert & Liu, Reference Nebert and Liu2019). Among the central nervous system diseases, SLC39A8 is associated with Parkinson's disease and schizophrenia (Pickrell et al., Reference Pickrell, Berisa, Liu, Segurel, Tung and Hinds2016; Schizophrenia Working Group of the Psychiatric Genomes Consortium, 2014), and the latter is possibly related to putamen gray matter volume mediated by SLC39A8 variants and expression (Luo et al., Reference Luo, Chen, Wang, Desrivières, Quinlan and Jia2019). In a phenome-wide approach, functional SLC39A8 variants are correlated with brain-related traits including delirium and dementia (McCoy, Pellegrini, & Perlis, Reference McCoy, Pellegrini and Perlis2019). In the immune system, reduced SLC39A8 expression decreases blood–brain barrier integrity, increases interleukin-6/interleukin-1β and nuclear factor kappa B expression following stimulation of tumor necrosis factor α, suggesting that ZIP8 dysfunction may exacerbate immune and inflammatory signaling (Kebir et al., Reference Kebir, Kreymborg, Ifergan, Dodelet-Devillers, Cayrol, Bernard and Prat2007; Liu et al., Reference Liu, Bao, Gálvez-Peralta, Pyle, Rudawsky, Pavlovicz and Knoell2013; Melia et al., Reference Melia, Lin, Xavier, Thompson, Fu, Wan and Donowitz2019). In a gastrointestinal system, a SLC39A8 variant is associated with Crohn disease and altered colonic mucosal microbiota (Li et al., Reference Li, Achkar, Haritunians, Jacobs, Hui, D'Amato and Duerr2016).
Among the seven loci identified in our meta-analysis of PTSD and PGM, rs6973700 is mapped to mitotic spindle-assembly checkpoint gene MAD1L1, which correlates with psychiatric susceptibility (Liu et al., Reference Liu, Xie, Fu, Yao, Han, Zhan and Zhu2021). For the meta-analysis of PTSD and IBS, we found that an independent SNP rs4937872, mapped to the neural cell adhesion molecule (NCAM1) gene, is associated with multiple psychiatric disorders (Shiwaku et al., Reference Shiwaku, Katayama, Kondo, Nakano, Tanaka, Yoshioka and Takahashi2022; Wendt et al., Reference Wendt, Pathak, Deak, De Angelis, Koller, Cabrera-Mendoza and Polimanti2022). Among genes identified in our gene-level analysis, BTN3A2 encodes a member of the immunoglobulin superfamily in the juxta-telomeric region of the major histocompatibility class 1 locus (Wu et al., Reference Wu, Bi, Zeng, Ma, Sun, Li and Yao2019). BTN3A2 is significantly up-regulated in induced pluripotent stem cells derived neurons from schizophrenia patients and gastric tumors (Wu et al., Reference Wu, Bi, Zeng, Ma, Sun, Li and Yao2019; Zhu et al., Reference Zhu, Yan, Ren, Huang, Zhu, Gu and Jin2017). Thus, these genes might serve as potential candidates for both PTSD and GIT disorders.
In the pathway-based analysis for PTSD and GIT disorders (PGM and IBD), we observed a significantly enriched pathway ‘regulation of immune response’, which plays a critical role in the brain–gut axis (Clark & Mach, Reference Clark and Mach2016; Roth, Zadeh, Vekariya, Ge, & Mohamadzadeh, Reference Roth, Zadeh, Vekariya, Ge and Mohamadzadeh2021). Immune dissonance represents a key element both in the pathogenesis of PTSD complications and in tissue repair (Jiang, Reference Jiang2008), while the adaptive immune response plays a major role in the pathogenesis of GIT disorders (Jacenik & Fichna, Reference Jacenik and Fichna2020). Analyzing SNP heritability enrichments for PTSD and GIT disorders, we found that GORD and IBS are mainly enriched in the central nervous and digestive systems, which is consistent with pathway-based analysis involving the brain–gut axis; and IBD is mainly enriched in the immune system, which emphasizes the vital role of immune response in PTSD and GIT disorders.
Limitations
We acknowledge several limitations of our study. First, to avoid bias from population stratification, we restricted all data to European ancestry, undermining generalization in other ethnic groups. Second, despite the large sample sizes of the consortium-based meta-analysis, the number of participants and SNPs differ among studies, limiting the direct comparison between different GIT disorders. Third, data from the UK biobank constitutes approximately 35% of the effective sample size of PTSD GWAS summary statistics, leading to considerable sample overlap in our study. Accordingly, we performed LDSC without a constrained intercept and used ‘power_prune’ function in MR analysis to minimize bias from sample overlap.
In conclusion, our study provides genetic insights into the association of PTSD and GIT disorders, and demonstrates a shared genetic susceptibility. We observed causal effects of GORD, PGM, IBS, and IBD on PTSD, and identified several genomic loci and genes shared by PTSD and GIT disorders, particularly the SLC39A8 gene (or isoforms), allowing translational and research implications for PTSD, GIT disorders and their co-morbidities.
Supplementary material
The supplementary material for this article can be found at https://doi.org/10.1017/S0033291723001423.
Data
All data are available in the main text or in the Supplementary materials.
Acknowledgments
We appreciate the participants and investigators for making the GWAS data available, Dr Xuejiao Song from West China School of Public health, and the support from Food Safety Monitoring and Risk Assessment Key Laboratory of Sichuan Province.
Author contributions
Conceptualization: Siquan Zhou and Jingyuan Xiong; investigation: Siquan Zhou, Hang Luo, Ye Tian, Haoqi Li, and Yaxian Zeng; visualization: Siquan Zhou, Hang Luo, Ye Tian, Haoqi Li, and Yaxian Zeng; funding acquisition: Jingyuan Xiong and Guo Cheng; project administration: Xiaoyu Wang and Shufang Shan; supervision: Jingyuan Xiong and Guo Cheng; writing – original draft: Siquan Zhou; writing – review and editing: Jingyuan Xiong, Guo Cheng, Hang Luo, Ye Tian, Haoqi Li, Yaxian Zeng, Xiaoyu Wang, and Shufang Shan. All authors critically reviewed, revised, and approved the final manuscript for publication.
Financial support
This study was supported by the Department of Science and Technology of Sichuan Province (Grant 2021YJ0156) and the Active Health and Aging Technologic Solutions Major Project of National Key R&D Program (Grant 2020YFC2006300).
Conflict of interest
The authors declare no competing interest.
Ethical standards
The authors assert that all procedures contributing to this work comply with the ethical standards of the relevant national and institutional committees on human experimentation and with the Helsinki Declaration of 1975, as revised in 2008, and the relevant national and institutional guides on the care and use of laboratory animals.
Role of the funder
The funders had no role in the design and conduct of the study; collection, management, analysis, and interpretation of the data; preparation, review, or approval of the manuscript; and decision to submit the manuscript for publication.