Introduction
Cognition is commonly measured using time-intensive paper and pencil batteries such as the Repeatable Battery for the Assessment of Neuropsychological Status (RBANS) or the Delis-Kaplan Executive Functions System (DKEFS). However, these legacy measures can be difficult to apply in epidemiological studies. Epidemiological studies that include adults with cognitive impairment based on the behavioral phenotype, rather than underlying etiology, require assessments that can be administered efficiently and interpreted across clinical conditions. The National Institutes of Health Toolbox Cognition Battery (NIH Toolbox-CB) aims to balance brevity with precision for accurate measurement of cognitive function (Weintraub et al., Reference Weintraub, Dikmen, Heaton, Tulsky, Zelazo, Bauer, Carlozzi, Slotkin, Blitz, Wallner-Allen, Fox, Beaumont, Mungas, Nowinski, Richler, Deocampo, Anderson, Manly, Borosh, Havlik, Conway, Edwards, Freund, King, Moy, Witt and Gershon2013).
The NIH Toolbox-CB is a performance-based measure that assesses attention, memory, language, and executive functions. Items are administered using an electronic tablet and completed within 30 min. NIH Toolbox-CB items demonstrated convergent validity with legacy neuropsychological measures of attention, memory, language, and executive functions across the lifespan in healthy, racially and ethnically diverse samples (Carlozzi et al., Reference Carlozzi, Beaumont, Tulsky and Gershon2015; Dikmen et al., Reference Dikmen, Bauer, Weintraub, Mungas, Slotkin, Beaumont, Gershon, Temkin and Heaton2014; Gershon et al., Reference Gershon, Cook, Mungas, Manly, Slotkin, Beaumont and Weintraub2014; Mungas et al., Reference Mungas, Heaton, Tulsky, Zelazo, Slotkin, Blitz, Lai and Gershon2014; Tulsky et al., Reference Tulsky, Carlozzi, Chiaravalloti, Beaumont, Kisala, Mungas, Conway and Gershon2014; Zelazo et al., Reference Zelazo, Anderson, Richler, Wallner-Allen, Beaumont, Conway, Gershon and Weintraub2014). However, validation in healthy samples assumes variance that cannot be assumed to translate to specific clinical populations (Delis et al., Reference Delis, Jacobson, Bondi, Hamilton and Salmon2003). Precision of the NIH Toolbox-CB among cognitively impaired samples with diverse etiologies remains unclear. Prior studies that employed the NIH Toolbox-CB among samples with neurological conditions described the ability to distinguish among samples based on expected impairments (e.g., distinguish among stroke, traumatic brain injury, spinal cord injury) (Carlozzi et al., Reference Carlozzi, Goodnight, Casaletto, Goldsmith, Heaton, Wong, Baum, Gershon and Tulsky2017a). Within homogenous populations, the NIH Toolbox-CB classified severity of impairments categorically (Carlozzi et al., Reference Carlozzi, Tulsky, Wolf, Goodnight, Heaton, Casaletto, Wong, Baum, Gershon and Heinemann2017b; Hackett et al., Reference Hackett, Krikorian, Giovannetti, Melendez-Cabrero, Rahman, Caesar, Chen, Hristov, Seifan, Mosconi and Isaacson2018). However, the association between continuous scores on NIH Toolbox-CB and scores on legacy measures of cognition among samples at elevated risk for cognitive impairment remain unclear. The present analysis is a step toward this.
Adults with chronic stroke and those with sickle cell disease (SCD) are examples of two populations whose behavioral phenotype may include cognitive impairments. Stroke affects 80 million adults globally, and among these, 32–43% experience persistent impairments in executive functions, memory, attention, processing speed, or language (Sexton et al., Reference Sexton, McLoughlin, Williams, Merriman, Donnelly, Rohde, Hickey, Wren and Bennett2019). These impairments are attributed to lesions in the brain caused by acute adult-onset vascular ischemia or hemorrhage (Zhao et al., Reference Zhao, Biesbroek, Shi, Liu, Kuijf, Chu, Abrigo, Lee, Leung, Lau, Biessels, Mok and Wong2018). SCD is an inherited blood disorder that causes diffuse organ damage, including extensive central nervous system pathology. People with SCD sustain silent cardiovascular events beginning in childhood. These events, including transient ischemic attacks, ischemic strokes, seizures, and high intracranial pressure, are not consistently detected clinically, and result in subtle cognitive impairments (Edwards et al., Reference Edwards, Raynor, Feliu, McDougald, Johnson, Schmechel, Wood, Bennett, Saurona, Bonner, Wellington, DeCastro, Whitworth, Abrams, Logue, Edwards, Martinez and Whitfied2007). Further, silent cardiovascular events and chronic hypoxia negatively influence cognitive development resulting in impairments in attention, memory, processing speed, and executive functions that persist in adulthood (Jorgensen et al., Reference Jorgensen, Metti, Butters, Mettenburg, Rosano and Novelli2017; Kirkham & Datta, Reference Kirkham and Datta2006). Among adults with SCD, 36% score 1 standard deviation and 5% score 2 standard deviations below the population mean in the Processing Speed Index of the Wechsler Adult Intelligence Scale III (Vichinsky et al., Reference Vichinsky, Neumayr, Gold, Weiner, Rule, Truran, Kasten, Eggleston, Kesler, McMahon, Orringer, Harrington, Kalinyak, De Castro, Kutlar, Rutherford, Johnson, Bessman, Jordan and Armstrong2010). Furthermore, approximately 50% of adults with SCD have silent cerebral infarcts which are associated with cognitive impairments (Kassim et al., Reference Kassim, Pruthi, Day, Rodegheier, Gindville, Brodsky, DeBaun and Jordan2016). Abnormalities in the basal ganglia, thalamus, and white matter integrity have been associated with neurocognitive impairments among adults with SCD (Mackin et al., Reference Mackin, Insel, Truran, Vichinsky, Neumayr, Armstrong, Gold, Kesler, Brewer and Weiner2014; Vichinsky et al., Reference Vichinsky, Neumayr, Gold, Weiner, Rule, Truran, Kasten, Eggleston, Kesler, McMahon, Orringer, Harrington, Kalinyak, De Castro, Kutlar, Rutherford, Johnson, Bessman, Jordan and Armstrong2010). While the underlying etiology of neurocognitive impairment varies between stroke and SCD, both groups experience poor outcomes in daily living associated with cognitive impairments (Mole & Demeyere, Reference Mole and Demeyere2018; Sanger et al., Reference Sanger, Jordan, Pruthi, Day, Covert, Merriweather, Rodeghier, DeBaun and Kassim2016).
People with stroke and SCD represent clinical populations that are at risk for cognitive impairment. Neurocognitive assessment can be particularly challenging in these populations due to the presence of fatigue and pain (Chakravorty & Williams, Reference Chakravorty and Williams2015; Duncan et al., Reference Duncan, Wu and Mead2012). Simultaneously, neurocognitive assessment is important in these populations who are at high risk for cognitive impairments. The NIH Toolbox-CB may be particularly advantageous because of the brevity of the test, combined with the opportunity to obtain a full cognitive profile. It is currently unclear if continuous scores on the NIH Toolbox-CB behave similarly across distinct samples with cognitive impairment. Thus, the aim of this secondary analysis was to explore associations between continuous scores on the NIH Toolbox-CB and legacy measures of cognition in two clinical populations with neurocognitive impairments of varied underlying etiology. We expected to observe significant associations between corresponding measures to the extent that the different subtests tap the same underlying cognitive process. That is, that subtests which measure similar underlying processes would have strong associations.
Methods
This is an exploratory secondary analysis of data from two studies. These studies contained two samples: people with stroke (cerebrovascular accident, CVA) and people with SCD. Data from each sample was analyzed separately.
Participants
Participants provided written informed consent. Study procedures were approved by The University of Pittsburgh Institutional Review Board and conducted in compliance with the Helsinki Declaration.
Participants with stroke
Community-dwelling people with chronic stroke (CVA) were participants in an intervention study that promoted engagement in daily activities to reduce sedentary behavior (ClinicalTrials.gov NCT03305731). Data were collected from December 2017 to December 2018. People who were: (1) greater than 6 months post-stroke; (2) ambulatory in the community; (3) reported greater than 6 hr of sedentary time daily (related to the parent study); and (4) resided within 50 miles of our research institution, were included. People who: (1) were currently participating in rehabilitation therapies (occupational, physical, or speech therapy); or who had: (2) current major depressive disorder, psychiatric disorder, or substance abuse disorder (Patient Health Questionnaire-9, PRIME-MD/Mini Neuropsychiatric Interview, (Kroenke et al., Reference Kroenke, Spitzer and Williams2001; Spitzer et al., Reference Spitzer, Williams, Kroenke, Linzer, Verloin deGruy, Hahn, Brody and Johnson1994); (3) cancer, in current treatment; or (4) diagnosis of neurodegenerative disorder, were excluded.
Participants with SCD
Participants with SCD were recruited from the University of Pittsburgh Medical Center (UPMC) Adult Sickle Cell Program outpatient clinic. Data were collected from October 2016 to December 2018 as part of a longitudinal study of neuroradiological biomarkers of cognitive function in SCD (ClinicalTrials.gov NCT02946905). All patients with HbSS, HbSC and HbS/β-thalassemia – the three most prevalent genotypes of SCD – older than 18, and able to provide informed consent were informed about the study by staff members during their routine clinic visit and offered entry into the study if they were in steady-state SCD (Ballas, Reference Ballas2012). Eligibility criteria also included: (1) English-speaking; and (2) currently receiving routine follow-up care at the UPMC Adult Sickle Cell Program. Exclusion criteria included: (1) pregnancy as determined by a positive urine human chorionic gonadotropin test at the time of informed consent and (2) acute medical problem including acute vaso-occlusive crisis.
Measures
Assessments were conducted by independent raters trained to criterion and supervised by a senior neuropsychologist (MB). Assessments were administered during one or two testing sessions. If two testing sessions were required, they were scheduled within the same week. The CVA sample completed assessments in their homes in a quiet testing area free of distractions. The SCD sample completed assessments in the research clinic. All participants from both samples received monetary compensation for completion of assessments (unrelated to effort; all participants in the same parent study received the same amount).
NIH toolbox-CB
The NIH Toolbox-CB is a performance-based assessment of cognitive functions conducted using a mobile application (NIH Toolbox v.1.21, Glinberg & Associates Inc, Madison, WI) on an iPad Air 2 (Apple, Cupertino, CA, USA) device which has a 9.7” display. Algorithms within the mobile application compute age-corrected standard scores based on population mean (100) and standard deviation (15), which were used in the present analysis. The NIH Toolbox-CB was validated across the lifespan (ages 3–85) among a healthy population and demonstrated good test-retest reliability in adults aged 20–85 (ICC > 0.72) on all subtests (Weintraub et al., Reference Weintraub, Dikmen, Heaton, Tulsky, Zelazo, Bauer, Carlozzi, Slotkin, Blitz, Wallner-Allen, Fox, Beaumont, Mungas, Nowinski, Richler, Deocampo, Anderson, Manly, Borosh, Havlik, Conway, Edwards, Freund, King, Moy, Witt and Gershon2013). This assessment battery contains seven subtests and was designed to take no more than 30 min. The subtests include: (1) Flanker Inhibitory Control and Attention; (2) Pattern Comparison Processing Speed; (3) List Sorting Working Memory; (4) Picture Sequence Memory; (5) Oral Reading Recognition; (6) Picture Vocabulary; and (7) Dimensional Change Card Sort. Administration procedures defined by the test developers were followed (including practice trials) and alternate test forms were used.
Flanker inhibitory control and attention
A row of stimuli (arrows) are presented at the center of the tablet screen. Participants indicate the direction of the center arrow by tapping the corresponding response button on the screen. Sometimes the center arrow matches the others, and sometimes it does not. Twenty test items are completed. The score for this subtest is based on speed.
Pattern comparison processing speed
Two stimuli are presented on the screen that may be the same or different. Participants are instructed to use their dominant hand to tap yes if the stimuli are the same and no if the stimuli are not the same. Participants are instructed to go as quickly as they can. The test continues until either 130 test items were completed or 85 s have passed. The score for this subtest is based on speed.
List sorting working memory
During condition 1, a series of stimuli that belong to the same category (either food or animals) are presented sequentially on the tablet screen. Participants are instructed to verbally indicate the stimuli that they observed on the screen from smallest to largest. During condition 2, a series of stimuli from two categories (food and animals) are presented. Participants are instructed to verbally indicate the stimuli that they observed on the screen beginning with food (from smallest to largest) and then animals (from smallest to largest). The assessor scores each response as correct (1) or incorrect (0).
Picture sequence memory
Images and verbal statements of events that might occur at a single event (such as Going to the Park) are presented sequentially and assigned the corresponding position around the edge of the tablet screen. Images are then scrambled in the center of the tablet screen and participants must replicate the sequence by dragging images to their appropriate position around the edge of the screen. Two test trials are completed, first with 15 items and second with 18 items.
Oral reading recognition
This is a computer adapted test in which participants are presented with words on the tablet that they must read out loud. The assessor scores correct (1) and incorrect (0) responses based on the NIH-CB pronunciation guide.
Picture vocabulary
This is a variable length computer adapted test in which a word is read aloud and four images are presented on the screen. Participants must select the image that corresponds with the word.
Dimensional change card sort
A stimulus is presented at the center of the screen, and two response options are provided on the screen. The participant is instructed to tap the response option which matches one of two dimensions (shape or color). Practice trials for each dimension are completed, and then thirty test items that include both dimensions are completed. The score for this subtest is based on speed.
Legacy neurocognitive measures
The Repeatable Battery for the Assessment of Neuropsychological Status (RBANS), Delis-Kaplan Executive Functions System (DKEFS), and Wide Range Achievement Test-4 (WRAT4) are well validated and widely applied measures of cognitive functions (e.g., Delis et al., Reference Delis, Kaplan and Kramer2001; Karr et al., Reference Karr, Hofer, Iverson and Garcia-Barrera2019; McFarland, Reference McFarland2020; Randolph et al., Reference Randolph, Tierney, Mohr and Chase1998; Wilkinson & Robertson, Reference Wilkinson and Robertson2006). The RBANS consists of 12 subtests that assess cognitive functioning across 5 domains: attention, language, immediate memory, delayed memory, and visuospatial/constructional. Subtests are described in detail by Randolph et al. (Reference Randolph, Tierney, Mohr and Chase1998). Age corrected scaled scores were computed for each subtest. In addition, scores from each subtest were used to derive the total index score (Randolph et al., Reference Randolph, Tierney, Mohr and Chase1998). Age-corrected scaled scores from DKEFS Color-Word Interference (Condition 3: Inhibition, Condition 4: Inhibition/Switching), Test of Trail Making (Condition 4: Switching), and Verbal Fluency (Condition 3: Category Switching) were used to assess executive functions. The WRAT4-Reading Subtest raw score was used to compute an age-corrected standard score (Wilkinson & Robertson, Reference Wilkinson and Robertson2006). Based on the designs of the parent studies, the WRAT4 was only administered to the SCD sample.
Statistical analyses
Four types of analyses were used to describe association between scores on the NIH Toolbox-CB and corresponding legacy measures: (1) linear correlations; (2) Bland-Altman analysis; (3) Lin’s Concordance Correlation Coefficient; and (4) dichotomous agreement using percent agreement and Cohen’s Kappa. Prior to conducting these analyses, corresponding subtests were identified by a senior neuropsychologist (MB) and guided by prior validity studies (Table 1, Carlozzi et al., Reference Carlozzi, Beaumont, Tulsky and Gershon2015; Dikmen et al., Reference Dikmen, Bauer, Weintraub, Mungas, Slotkin, Beaumont, Gershon, Temkin and Heaton2014; Gershon et al., Reference Gershon, Cook, Mungas, Manly, Slotkin, Beaumont and Weintraub2014; Mungas et al., Reference Mungas, Heaton, Tulsky, Zelazo, Slotkin, Blitz, Lai and Gershon2014; Tulsky et al., Reference Tulsky, Carlozzi, Chiaravalloti, Beaumont, Kisala, Mungas, Conway and Gershon2014; Zelazo et al., Reference Zelazo, Anderson, Richler, Wallner-Allen, Beaumont, Conway, Gershon and Weintraub2014). Analyses were conducted using SPSS Statistics for Windows, version 27.0 (SPSS Inc, Chicago, Ill., USA) and Microsoft Excel. Across all subtests, age-corrected scores were converted to Z-scores using the population mean and standard deviation for each measure (M[SD] for NIH Toolbox-CB and WRAT4-Reading = 100[15]; DKEFS = 10[3]; RBANS used age-specific population mean and standard deviation). Because the NIH Toolbox-CB Total Cognition Composite Score and RBANS Total Index Scores are both scaled using population M(SD) = 100(15), Z-scores were not computed for total scores. Data were analyzed by sample. Distributions were examined for each subtest and normality was assessed using Shapiro-Wilk (α = .05). Linear correlations between continuous scores on NIH Toolbox subtests and corresponding legacy subtests were assessed using Pearson’s r (or Spearman’s rho, if nonnormally distributed). Correlations were interpreted as weak (.1 to .3), moderate (.4 to .6), strong (.7 to .9), and perfect (1.0) (Akoglu, Reference Akoglu2018). Then association between continuous scores on the NIH Toolbox-CB and corresponding legacy subtests were assessed using Bland-Altmann analyses (Giavarina, Reference Giavarina2015). First, new variables were created for each comparison that described: (1) difference between Z-scores; and (2) mean Z-scores. A one-samples t-test was conducted to determine if the mean difference between Z-scores differed from 0 (α = .05). We report the magnitude and direction of difference from 0. Plots of the mean Z-scores versus difference between Z-scores and simple linear regression models were examined to determine if there were differences in magnitude and direction of agreement across sample means. Limits of agreement were computed. Next Lin’s CCC (r) was computed for each comparison to describe the degree of precision and accuracy of NIH Toolbox-CB subtests relative to corresponding subtests on legacy measures (Barnhart et al., Reference Barnhart, Haber and Lin2007; Lawrence & Lin, Reference Lawrence and Lin1989). The CCC was interpreted using the same classifications as for the linear correlations described above, following Altman’s recommendation to interpret the CCC similar to Pearson’s r (Altman, Reference Altman1991). Point estimates of the CCC within 95% confidence intervals were plotted to enable visual comparison among samples. Lastly, composite scores on the NIH Toolbox and RBANS were dichotomized to consider scores ≤1.5 SD as impaired, and all others not impaired. This is a widely used cut point for impairment on neuropsychological measures that represents scores at or below approximately the 10th percentile (Ciafone et al., Reference Ciafone, Little, Thomas and Gallagher2020). Percent agreement and Cohen’s Kappa were computed. Agreement based on Cohen’s Kappa was interpreted as slight (.0 to .2), fair (.2 to .4), moderate (.4 to .6), substantial (.6 to .8), and almost perfect (greater than .8) (Watson & Petrie, Reference Watson and Petrie2010).
Table 1. NIH Toolbox-CB and corresponding legacy measures

Results
Participants
Participant characteristics are displayed in Table 2. Analyses were completed by group.
Table 2. Participant characteristics

* Note. n = 25; exact years of education unknown for n = 1 with less than high school education.
Stroke
The stroke group (n = 26) averaged 68.85 (11.22) years of age and 46.2% were male. The majority of the group was white (76.9%), and had an average of 14.76 (2.93) years of education. Participants had sustained ischemic (84.6%) or hemorrhagic (15.4%) strokes affecting the right hemisphere (42.3%), on average 27.23 (13.76) months prior to enrollment in the research study.
SCD
The SCD group (n = 64) averaged 36.20 (11.92) years of age and 51.6% were male. The majority of this group was black (96.2%) and had an average of 13.52 (1.98) years of education. Participants in this group carried the Hb-SS (46.9%), Hb S/beta thalassemia (17.2%), and Hb-C (35.9%) genotype.
Primary outcomes
Sample mean scores (Table 3) on the NIH Toolbox-CB fell within 1 standard deviation above or below the population mean (85 to 115) on all subtests and composite scores in the CVA sample except for Flanker Inhibitory Control and Attention, M(SD) = 84.8(11.0) and Pattern Comparison Processing Speed, M(SD) = 78.9(26.6), and on all subtests and composite scores in the SCD sample except for Flanker Inhibitory Control and Attention, M(SD) = 78.9(14.7) and Fluid Cognition Composite Score, M(SD) = 82.5(17.6). On legacy measures, sample mean scores fell within 1 standard deviation above or below the population mean (7 to 13) on all subtests in the CVA sample except for RBANS Figure Copy, M(SD) = 6.8(5.8), and on all subtests in the SCD sample except for RBANS Coding, M(SD) = 5.9(4.1) and DKEFS Test of Trail Making Condition 4, M(SD) = 5.7(3.9).
Table 3. Distribution of age-corrected scores on cognitive measures

NIH Toolbox-CB subtest and composite, population mean(SD) = 100(15).
RBANS subtest, population mean(SD) = 10(3).
RBANS total index, population mean(SD) = 100(15).
DKEFS subtest, population mean(SD) = 10(3).
WRAT4 subtest, population mean(SD) = 100(15).
Note. Table S1 in the supplementary file contains NIH Toolbox-CB T-scores for this same sample, which are fully corrected for age, education, sex, and race/ethnicity.
Association between continuous scores on NIH Toolbox-CB and legacy measures
Results of the correlational analyses, Bland-Altman analyses, and Lin’s Concordance Correlation Coefficients are presented in Table 4. Bland-Altman plots for all comparisons are available in the supplementary materials. Based on linear correlations, strong agreement was observed between NIH Toolbox-CB Fluid Cognition Composite Scores and RBANS Total Index Scores (CVA, r = .90, p < .05; SCD, r = .88, p < .05), and NIH Toolbox-CB Total Cognition Composite Scores and RBANS Total Index Scores (CVA, r = .83, p < .05). Lin’s CCC demonstrated strong concordance between NIH Toolbox-CB Fluid and Total Cognition scores versus RBANS Total Index Scores in the CVA sample (r = .78 to .79, p < .05) and only moderate concordance in the SCD sample (r = .60, p < .05). The limits of agreement were wide in both samples when comparing NIH Toolbox-CB Fluid Cognition and NIH Toolbox-CB Total Cognition scores with the RBANS Total Index Score (Table 4). Among subtests, strong linear correlations were observed between NIH Toolbox-CB ORR and WRAT4 (SCD, r = .81, p < .05) and NIH Toolbox-CB DCCS and DKEFS Trails 4 (CVA, r = .77, p < .05). Lin’s CCC demonstrated strong concordance between NIH Toolbox-CB ORR and WRAT 4 (SCD, r = .82, p < .05), and only moderate concordance between NIH Toolbox-CB DCCS and DKEFS Trails 4 (CVA, r = .63, p < .05). The limits of agreement were wide for both subtests (Table 4). Concordance between NIH Toolbox-CB subtests and corresponding legacy measures is depicted in Figure 1.
Table 4. Association between age-corrected scores on NIH Toolbox-CB and legacy measures

Note. Mean Δ = [NIH Toolbox Z-score] – [Legacy measure Z-score] for all subtests; [NIH Toolbox scaled score] – [Legacy measure scaled score] for total composite and total index scores. Statistical significance indicates whether Mean Δ differs from 0.
FL = Flanker Inhibitory Control and Attention; PCPS = Pattern Comparison Processing Speed; PSM = Picture Sequence Memory; DCCS = Dimensional Change Card Sort; Total Cognition = Total Cognition Composite; Fluid Cognition = Fluid Cognition Composite; CWI 3 = DKEFS Color-Word Interference Condition 3: Inhibition; CWI 4 = DKEFS Color-Word Interference Condition 4: Switching; Coding = RBANS Coding; Figure Recall = RBANS Figure Recall; Story Recall = RBANS Story Recall; List Recall = RBANS List Recall; Trails 4 = DKEFS Test of Trail Making Condition 4: Switching; Fluency 3 = DKEFS Verbal Fluency Condition 3: Category Switching; Total Index = RBANS Total Index; WRAT4 = Wide Range Achievement Test Version 4-Reading.
* p < .05.
** Pearson’s r for all except DCCS versus Trails 4 (CVA and SCD samples), FL versus CWI 3 (CVA sample), PSMT versus Story Recall (CVA and SCD samples), ORR versus WRAT4 (SCD sample). Spearman’s rho was used in these cases due to non-normal distribution.
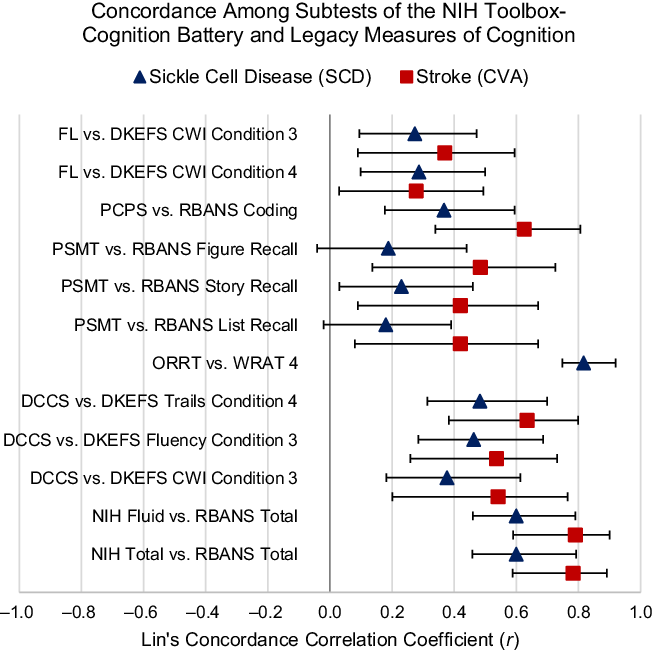
Figure 1. Concordance among subtests of the NIH Toolbox-Cognition Battery and legacy measures of cognition. Forest plot which illustrates the magnitude of Lin’s Concordance Correlation Coefficient (r) in the sickle cell disease (blue triangles) and stroke (red squares) samples within 95% confidence intervals (error bars). Abbreviations: FL = Flanker Inhibitory Attention and Control; PCPS = Picture Comparison Processing Speed; PSMT = Picture Sequence Memory Test; ORRT = Oral Reading Recognition Test; DCCS = Dimensional Change Card Sort; NIH Total = NIH Toolbox Total Cognition Composite Score; DKEFS CWI = Delis-Kaplan Executive Functions Test Color-Word Interference; DKEFS Trails = Delis-Kaplan Executive Functions Test, Test of Trail Making; DKEFS Fluency = Delis-Kaplan Executive Functions Test Verbal Fluency; RBANS = Repeatable Battery for the Assessment of Neuropsychological Status; WRAT 4 = Wide Ranging Achievement Test, Version 4 Reading.
Agreement between impairment classification on NIH Toolbox-CB and legacy measures
Impairment classifications determined by NIH Toolbox-CB Composite Scores (Fluid Cognition and Total Cognition) were examined against the RBANS Total Index Score (Table 5). Across all comparisons, percent agreement ranged from 72 to 96%. Substantial agreement between the NIH Toolbox-CB Total Cognition Composite Score and the RBANS Total Index Score was detected in the CVA sample (Kappa = .78, p < .05). All other comparisons demonstrated only fair agreement (Kappa = .34 to .36, p < .05). Percent agreement between impairment classification on NIH Toolbox-CB subtests and corresponding legacy measures is available in the supplementary file (Table S2).
Table 5. Agreement in impairment classifications between RBANS total index and NIH Toolbox composite measures

Note. Impaired = 1.5 SD below population mean. Kappa values interpreted as fair = .21 to .40, moderate = .41 to .60, substantial = .61 to .80.
* p < .05.
Discussion
Examining association between continuous scores on the NIH Toolbox-CB and legacy neuropsychological measures in samples at risk for cognitive impairments is a step toward validation of the NIH Toolbox-CB to characterize cognitive impairments across neurologically impaired populations. Scores on NIH Toolbox-CB had weak to strong associations with corresponding legacy measures in both samples. Wide limits of agreement (>1 SD in either direction from the population mean) were observed across all subtests and composite scores. In addition, proportional bias detected on several subtests and the Fluid Cognition Composite Score in the SCD sample suggest that differences between individuals’ scores on NIH Toolbox-CB and legacy measures may vary depending on the score. Group mean differences between Z-scores on NIH Toolbox-CB subtests and corresponding legacy measures were <1 SD in either direction, suggesting that these subtests may provide adequate estimates of group mean cognitive function among people with CVA and SCD. Further, despite limited overlap of cognitive functions assessed by the NIH Toolbox-CB and the RBANS, the NIH Toolbox-CB Total Cognition Composite Score had moderate to strong associations, and the NIH Toolbox-CB Fluid Cognition Composite Score had strong associations, with the RBANS Total Index Score. This suggests that the NIH Toolbox-CB Total Cognition Composite Score may be a valid measure of overall cognitive function among people with CVA and SCD.
Associations observed between NIH Toolbox-CB subtests and corresponding legacy measures were similar to those reported in prior validation studies of healthy populations on Pattern Comparison Processing Speed (r = .40 to .65 vs. r = .36 to .54, Carlozzi et al., Reference Carlozzi, Beaumont, Tulsky and Gershon2015) and Oral Reading Recognition (r = .81 vs. r = .86) (Gershon et al., Reference Gershon, Cook, Mungas, Manly, Slotkin, Beaumont and Weintraub2014). The present findings support the use of NIH Toolbox-CB ORR among people with SCD. On measures of executive functions, the magnitude of association was smaller in the present study relative to healthy validations samples when comparing the Flanker Inhibitory Control and Attention subtest with Color Word Interference (Condition 3, Inhibition, r = .35 to .48 vs. r = .52) and the Dimensional Change Card Sort with Color Word Interference in the SCD sample (Condition 4, Inhibition/Switching r = .39 vs. r = .55) (Zelazo et al., Reference Zelazo, Anderson, Richler, Wallner-Allen, Beaumont, Conway, Gershon and Weintraub2014). A similar association between the Dimensional Change Card and Color Word Interference was detected in the CVA sample versus prior healthy validation studies (Condition 4, Inhibition/Switching r = .55 vs. r = .55) (Zelazo et al., Reference Zelazo, Anderson, Richler, Wallner-Allen, Beaumont, Conway, Gershon and Weintraub2014). Prior validation studies did not examine the Flanker Inhibitory Control and Attention subset relative to the Color Word Interference: Condition 4 (Inhibition/Switching) subtest, nor did they examine the Dimensional Change Card Sort relative to switching subtests on the Test of Trail Making and Verbal Fluency. The present findings suggest that switching may be related to the NIH Toolbox-CB Flanker Inhibitory Control and Attention, but that these subtests may tap different underlying constructs. Further, only one legacy measure of switching (Test of Trail Making) had a strong association with the Dimensional Change Card Sort in the CVA sample (r = .77). Moderate associations between Dimensional Change Card Sort and additional legacy measures of switching (r = .48 to .62) suggest that these subtests measure related but different cognitive functions among people with CVA and SCD. The Picture Sequence Memory Test was not associated with Figure Recall or List Recall in the SCD sample, and was only moderately associated with Figure Recall and List Recall in the CVA sample, and Story Recall in both samples. Differences in the stimuli themselves (e.g. story, abstract image, words) and the length of time elapsed between presentation of the stimuli and recall on these subtests may contribute to these discrepancies.
An overall cognition score may be computed on both the NIH Toolbox-CB and the RBANS. Only 2 of the 7 NIH Toolbox-CB subtests overlap with cognitive functions assessed by the RBANS (Pattern Comparison Processing Speed, Picture Sequence Memory). Despite these differences, the NIH Toolbox-CB Fluid and Total Cognition Composite Scores and the RBANS Total Index Scores had moderate to strong associations. Further, these associations were stronger than associations on individual subtests and corresponding legacy measures (except for Oral Reading Recognition). This suggests that the NIH Toolbox Fluid and Total Cognition Composite Scores may represent the g-factor proposed by Spearman (Reference Spearman1904) and be useful as a general measure of cognition among people with CVA and SCD.
Limitations
Results of the present analysis should be interpreted as exploratory because of limitations imposed by the purpose of the primary data collection. The CVA sample was smaller than the SCD sample. Although scores in analyses are age-adjusted for all measures, we did not adjust for race, ethnicity, or education. In addition, normative samples with unknown population-based equivalence were used to derive population norms for the DKEFS, RBANS, and WRAT4. We used these population-based M(SD) to compute the standard scores used in the analyses, and remain mindful that this could contribute to bias. Scores on legacy measures of neuropsychological function are biased against Black people, largely reflective of disparities in social systems such as education quality and economic opportunity (Jean et al., Reference Jean, Lindbergh, Mewborn, Robinson, Gogniat and Miller2019). There are varied published methods for adjusting NIH Toolbox-CB scores by race (Casaletto et al., Reference Casaletto, Umlauf, Beaumont, Gershon, Slotkin, Akshoomoff and Heaton2015; Heaton et al., Reference Heaton, Akshoomoff, Tulsky, Mungas, Weintraub, Dikmen, Beaumont, Casaletto, Conway, Slotkin and Gershon2014) and extremely limited representation of Black people in neuropsychological research (Pugh et al., Reference Pugh, Robinson, De Vito, Bernstein and Calamia2021). This is reflected in our predominantly white CVA sample. Future research which specifically recruits people from underrepresented racial and ethnic groups and applies optimal methods to produce fully adjusted scores accounting for race, ethnicity, education, and other social factors is important for building on these exploratory findings.
Future directions
Future studies that build on this work should aim to confirm findings among a broader range of clinical samples who have disorders associated with cognitive impairments. These studies will facilitate precise interpretation of NIH Toolbox-CB and support the use of this tool in broad epidemiological studies inclusive of people at risk for cognitive impairments.
Supplementary Material
To view supplementary material for this article, please visit https://doi.org/10.1017/S1355617722000406
Acknowledgements
We would like to acknowledge Michelle Zmuda and Isaac Delozier for recruiting participants and administering tests, and staff of the Occupational Therapy Cognitive Performance Laboratory for their role in participant recruitment and data collection. We would also like to acknowledge the blinded peer reviewers whose thoughtful feedback strengthened this paper.
Authors’ contribution
Kringle EA: Conceptualization, Investigation, Formal analysis, Writing - Original Draft, Funding acquisition; Novelli E: Conceptualization, Investigation, Writing - Review & Editing, Funding acquisition; Butters MA: Conceptualization, Writing - Review & Editing, Supervision; Skidmore ER: Conceptualization, Investigation, Resources, Writing - Review & Editing, Funding acquisition.
Funding statement
Research reported in this publication was supported by the National Heart Lung and Blood Institute (R01 HL127107-01A1, T32 HL134634, K23 HL159240), the National Center for Advancing Translational Sciences (UL1 TR001857) of the National Institutes of Health, the National Institute on Disability, Independent Living and Rehabilitation Research (90AR5023), the University of Pittsburgh School of Health and Rehabilitation Sciences PhD Student Award, and University of Pittsburgh Occupational Therapy Department Funds. The content is solely the responsibility of the authors and does not necessarily represent the official views of the National Institutes of Health, the National Institute on Disability, Independent Living and Rehabilitation Research, or the University of Pittsburgh.
Conflicts of interest
None.