1. Introduction
Laparoscopic Surgery is a type of surgery where contrary to the conventional open surgery the surgeon makes tiny incisions that are only big enough to insert special surgical instruments and a laparoscope within a trocar [Reference Queirós, Vilaça, Rodrigues, Neves, Teixeira and Correia-Pinto1]. These types of surgeries have grown rapidly and become more preferred by patients because of their numerous advantages [Reference Agha and Muir2]. They have a great impact on reducing the patient’s pain, recovery time, cost as well as the scarring in the patient. However, when it comes to the surgical team, laparoscopic surgeries impose more challenges compared to conventional surgeries. These challenges include restricted area of operation, lack of depth perception due to the two-dimensional visual feedback, reduction in sensory feedback during surgery, and difficulty of instrument manipulation due to the fulcrum effect (the instrument tip moves to the opposite direction of the surgeon’s hand due to pivot point) and limited number of degrees of freedom (DOFs) [Reference Agha and Muir2, Reference Sánchez-Margallo, Sánchez-Margallo, Usón and Sanchez-Margallo3]. In addition, the surgeon would lose orientation of the 3D view because of laparoscope shake if the laparoscope operator were unskilled or became tired after long operating hours [Reference Das, Shirwalkar and Kar4–Reference Saing, Sotthivirat, Vilasrussamee and Suthakorn6].
Accordingly, many researchers have shed light on implementing technologies to reduce laparoscopic surgeries’ challenges. Surgical robots have been substantially exploited in the last few decades, with the first medical robot used in 1985 [Reference Beasley7]. Furthermore, due to the numerous advantages and challenges of laparoscopic surgeries, laparoscopic robots have became one of the most active areas for research and development of surgical robots. Robotic systems have increased the surgical dexterity in various ways [Reference Lanfranco, Castellanos, Desai and Meyers8]; increasing DOFs of the instruments greatly enhances the surgeon’s ability to manipulate instruments and thus the tissues, compensating the surgeon’s tremor on the end-effector motion by suitable design of robot hardware and software filters, in addition to scaling the surgeon’s relatively large movements of the control grips into micro-motions inside the patient.
Surveys of laparoscopic robots are scarce [Reference Kalan, Chauhan, Coelho, Orvieto, Camacho, Palmer and Patel9, Reference Kawashima, Kanno and Tadano10]. Most of them, however, concentrate on certain systems such as laparoscope positioners [Reference Bihlmaier11–Reference Taniguchi, Nishikawa, Sekimoto, Kobayashi, Kazuhara, Ichihara, Kurashita, Takiguchi, Doki, Mori and Miyazaki14], tele-operated master–slave systems [Reference Sood, Arya, Maple, Grange and Haq15–Reference Koukourikis and Rha18], or hand-held devices [Reference Payne and Yang19, Reference Sánchez-Margallo, Sánchez-Margallo and Szold20]. The aim of this paper is to review various kinds of robots used in laparoscopic surgeries simultaneously considering different points of view with comparisons of the numerous requirements and control techniques. To achieve this aim, the following section presents several kinds of robots that are used in laparoscopic surgeries, classifying them based on application, configuration, number of DOFs, and control scheme. Then, a generalized block diagram for all robotic systems involved in the field of laparoscopy is presented. A section dedicated for the application of these robotic systems presents their experimental and clinical evaluation. Finally, a discussion is followed by the conclusion of the paper, which suggests future prospects regarding surgical robots in laparoscopic surgeries.
This paper provides a literature review of the state of art of laparoscopic robots. Contrary to the existing review papers that classify laparoscopic robots according to their application only or focus on a single category, this paper classifies the proposed systems into main categories according to their application and provides further classifications of the systems based on their configuration, DOFs, and control scheme, in addition to presenting a generalized block diagram that summarizes and compares the control scheme of each category.
2. Overview of robots in laparoscopic surgeries
2.1. Literature review methods
To provide a comprehensive review of robotics in laparoscopic surgery, general searches using online databases like Google scholar, IEEE, ResearchGate, PubMed Central, and ScienceDirect were performed. Keywords such as “Robotic assisted surgery”, “Laparoscopic robots”, “Robotic laparoscopy” were used for a general search. Then data were gathered separately for each robotic application using keywords such as “Laparoscope positioner”, “Voice controlled”, “Head movements control”, “Instrument tracking”, “Tele-operated surgery”, “Robotic training”, “record/playback”, “Robotized laparoscopic tool”, “handheld laparoscopic devices”. Clinical and experimental results of each proposed system were gathered using keywords entry such as “Clinical”, “Experimental”, “Use”, “Evaluation” along with the system’s name. Moreover, the flowchart presented in Fig. 1 depicts inclusion and exclusion criteria for the references included in the literature review.

Figure 1. Flowchart of the literature review methods showing inclusion and exclusion criteria.
Laparoscopic robots may be classified according to their applications, configurations, number of DOFs as well as the employed control technique. The following subsections depict these classifications.
2.2. Classification based on the application of the system
Based on the application of the robotic system applied in the laparoscopic surgeries, four main categories can be outlined, as shown in Fig. 2. The figure depicts the main categories as being handheld laparoscopic devices, laparoscope positioning systems, master–slave teleoperated systems with dedicated consoles (MSTDC), and robotic training systems. The details of these systems are presented in the following subsections.

Figure 2. Classification of robotic systems used in laparoscopic surgeries based on their application.
2.2.1. Handheld laparoscopic devices
Conventional laparoscopic instruments such as forceps, needle drivers, and scissors which do not include robotic actuators may be considered very primitive. They however can be classified as the basic type of handheld laparoscopic devices. They include a simple mechanism which is responsible for transferring the motion to the end effector by simple grasping motion of the surgeon’s hand.
Moreover, handheld motorized devices [Reference Payne and Yang19–Reference Sieber, Fellmann-Fischer and Mueller28] have been developed as an approach for relatively cheap, lightweight robotic systems with a short setup time and a small physical footprint, where the master and slave are integrated in one device. These systems are held and manipulated by surgeons just like how conventional instruments are handled [Reference Payne and Yang19]. However, they provide surgeons with more ergonomic handles, intuitive control of motorized movements of the instrument, and increased number of DOFs. They help surgeons with tasks that are considered challenging with manual manipulation and enhance their surgical dexterity.
A robotized needle holder Jaimy was developed by Endocontrol Medical (Grenoble, France), and its effect on ergonomics and skills has been studied in box trainers [Reference Bensignor, Morel, Reversat, Fuks and Gayet21, Reference Siri, Crochet, Charavil, Netter, Resseguier and Agostini29]. Another commercialized motorized needle holder is the fully steam sterilizable Robot DEX by Dextérité Surgical (Annecy, France) [Reference Sánchez-Margallo and Sánchez-Margallo27]. A study evaluating its performance in a pelvitrainer showed that it allows precise stitching, reduces tearing, and improves the quality of cuts [Reference Madec, Dariane and Cornu30].
Kymerax by Terumo company (Tokyo, Japan) is a handheld laparoscopic system with interchangeable instruments (scissors, dissector, needle, and L-hook), driven by robotic technology [Reference Sánchez-Margallo, Sánchez-Margallo and Szold20, Reference Iacoponi, Terán, De Santiago and Zapardiel23, Reference Sieber, Fellmann-Fischer and Mueller28]. It was clinically used in laparoscopic hysterectomy for uterine sarcoma, reporting more accurate movements and operative time that is comparable to conventional laparoscopy (80 minutes using Kymerax and 75 ± 21 minutes for conventional laparoscopy) and less than that of robotic surgery [Reference Iacoponi, Terán, De Santiago and Zapardiel23]. However, it was taken off the market in 2014 due to difficulties in sterilization of the motorized instruments [Reference Rassweiler and Teber31].
Table I summarizes key features and experimental results of the three commercialized handheld devices (Kymerax, Jaimy, and RobotDex), and a comparison between handheld motorized devices and conventional laparoscopic instruments is summarized in Table II.
Table I. Key features and performance evaluation of commercialized handheld laparoscopic robots.

Table II. Comparison between handheld laparoscopic robots and conventional laparoscopic instrument in terms of structure and ergonomics.

Furthermore, new prototypes of motorized laparoscopic instruments of KARL Storz SE & Co., (Tuttlingen, Germany) have been tested to compare their performance with conventional laparoscopic instruments [Reference Uysal, Gasch, Behnisch, Nickel, Müller-Stich, Hohenfellner and Teber32]. The study showed that using the conventional instruments was faster, with fewer exercise failures in needle guidance and its usability was preferred; however, participants valued the additional DOFs [Reference Uysal, Gasch, Behnisch, Nickel, Müller-Stich, Hohenfellner and Teber32].
Wuhan University and Harbin Institute of Technology in China developed a laparoscopic robotized needle holder consisting of a wired handle and a flexible tip mimicking da Vinci’s articulated arm design to retain its 7 DOFs feature [Reference Feng, Yang, Zhang, Li, Chen, Yan, Du and Wang33]. Its performance was evaluated using a force-sensing test platform, and the study showed that the use of the robotized needle holder reduced the force generated during operation; however, its learning curve was longer than that of conventional instruments [Reference Feng, Yan, Li, Zhang, Chen, Du and Yang34].
Moreover, Miyazaki et al. [Reference Miyazaki, Hirose, Ishikawa, Kanno and Kawashima26] proposed a master–slave integrated handheld robot, where the master controller (joystick) is integrated in the end of the pneumatically driven forceps (the slave). The proposed system focused on suturing using a curved needle, and the experimental results showed a favorable reduced contact force against organ models compared to conventional suturing.
Earlier studies proposed a prototype model of a master-slave combined system and confirmed its validity as a handheld robotic forceps [Reference Jinno, Matsuhira, Sunaoshi, Hato, Miyagawa, Morikawa, Furukawa, Ozawa, Kitajima and Nakazawa24, Reference Matsuhira, Jinno, Miyagawa, Sunaoshi, Hato, Morikawa, Furukawa, Ozawa, Kitajima and Nakazawa25]. The slave forceps are cable-driven by DC servo motors mounted near the master hand grip, and the master grip is integrated at the end of the forceps’ shaft [Reference Jinno, Matsuhira, Sunaoshi, Hato, Miyagawa, Morikawa, Furukawa, Ozawa, Kitajima and Nakazawa24]. Focacci et al. [Reference Focacci, Piccigallo, Tonet, Megali, Pietrabissa and Dario22] developed lightweight robotic forceps, by separating actuators from the main body using a passive holder, and preliminary tests emphasized its use in difficult suturing procedures.
Moreover, handheld devices can use virtual fixtures to limit the movement of the laparoscopic instrument during surgery and prevent damaging the surrounding tissues in high-risk areas. Virtual fixtures are computer-generated constraints that limit the robot’s motion into restricted pre-defined regions and/or constrain it to move along a desired path [Reference Li, Kapoor and Taylor35, Reference Selvaggio, Fontanelli, Ficuciello, Villani and Siciliano36]. A recent study proposed a human-robot interface that provides safe operation constraining the movement of a robotic forceps using virtual remote center of motion (RCM) [Reference Colan, Nakanishi, Aoyama and Hasegawa37].
2.2.2. Laparoscope positioning systems
There are two types of rigid laparoscopes, forward-viewing laparoscope and oblique-viewing laparoscope [Reference Yamaguchi, Nakamoto, Sato, Nakajima, Konishi, Hashizume, Nishii, Sugano, Yoshikawa and Yonenobu38]. In oblique-viewing laparoscopes, the viewing direction has a tilt from the laparoscope cylinder axis. This allows for easily changing the viewing direction by rotating the laparoscope around its axis, providing a larger field of view. However, oblique-viewing laparoscopes require an additional DOF and a more difficult scope calibration process [Reference Yamaguchi, Nakamoto, Sato, Nakajima, Konishi, Hashizume, Nishii, Sugano, Yoshikawa and Yonenobu38–Reference Wu, Jaramaz and Narasimhan42].
Positioning the laparoscope using motorized robotic systems can be approached with several techniques [Reference Pandya, Reisner, King, Lucas, Composto, Klein and Ellis13]. Some of these techniques require manual control of the robot. This may be effectuated using hand/foot pedals directly operated by the surgeon or assistant [Reference Kraft, Jäger, Kraft, Leibl and Bittner43–Reference Vaida, Pisla, Plitea, Gherman, Gyurka, Stancel, Hesselbach, Raatz, Vlad and Graur45].
Other techniques require direct commands from the surgeon such as vocal, or head movements commands. Other methods are reactive, where sensors track data such as the surgeon’s eye gaze [Reference Li, Zhang, Xue, Kim and Zhang5] or the instrument position, and the laparoscope is moved directly according to sensor readings. Proactive strategies use higher level control, where a cognitive robot has preliminary knowledge of the surgical procedures and is able to adjust the laparoscope’s view according to the prediction of the next step in the surgery.
Voice control
The AESOP robot developed by Computer Motion, is considered one of the first robots to enter the surgical arena and the first voice controlled to be approved by the Food and Drug Administration (FDA) [Reference Mendivil, Holloway and Boggess46]. AESOP1000 was developed in 1993 based on shared control principle. It was put in clinical use in 1994 [Reference Kraft, Jäger, Kraft, Leibl and Bittner43] and has undergone several modifications. AESOP is a SCARA type robotic arm [Reference Jaspers, Breedveld, Herder and Grimbergen12] that could be controlled manually or remotely with hand or foot control. Its newer version AESOP2000 was developed in 1996 [Reference Nerli, Reddy, Chowdhary and Gundeti, ed.)47] and is controlled using voice commands, which proved its excellence over older versions, as the voice control eliminated the need for human laparoscope operator [Reference Chatzilias, Kamarianakis, Golemati and Christodoulou48–Reference Weghorst, Sieburg and Morgan50]. The learning curve of the AESOP was evaluated and has shown that the use of the AESOP robot was not as fast as hand control, but the skill to use it was learned as quickly [Reference Jacobs, Shayani and Sackier51].
VIKY from Endocontrol Medical (Grenoble, France), another laparoscopic holder that is available for commercial use, is considered an evolutionary system of the AESOP as it is also FDA approved and has been used in several laparoscopic surgeries [Reference Takahashi and Abedin-Nasab, ed.)52, Reference Voros, Haber, Menudet, Long and Cinquin53]. It is a compact, lightweight, fully sterilizable robot. VIKY is either controlled by foot pedal or voice commands and is small enough to be placed directly on the operating room table without interfering with other held instruments.
Another laparoscope holder was developed in 2008 in Romania, the PARAllel robot for Minimally Invasive Surgery (PARAMIS). It was designed to be a laparoscope holder controlled with a keyboard, joy stick, haptic, or voice command control [Reference Pisla, Plitea, Vaida, Hesselbach, Raatz, Vlad, Graur, Gyurka, Gherman and Suciu44, Reference Vaida, Pisla, Plitea, Gherman, Gyurka, Stancel, Hesselbach, Raatz, Vlad and Graur45]. However, the voice control mode was preferred by surgeons as it allows them to operate with both hands. The PARAMIS is a simple parallel robot whose geometric and kinematic models are described by Plitea et al. [Reference Plitea, Hesselbach, Vaida, Raatz, Pisla, Budde, Vlad, Burisch and Senner54]. Its open architecture control system which consists of three levels; user interface, programmable logic controller (PLC), and the robot level is presented in ref. [Reference Vaida, Pisla, Plitea, Gherman, Gyurka, Stancel, Hesselbach, Raatz, Vlad and Graur45]. It allows fast introduction of new commands without any physical modification [Reference Vaida, Pisla, Plitea, Gherman, Gyurka, Stancel, Hesselbach, Raatz, Vlad and Graur45].
Head movements control
One of the oldest laparoscope holders controlled by head movements is the Endosista (Armstrong Projects Ltd.) [Reference Jaspers, Breedveld, Herder and Grimbergen12, Reference Ornstein55, Reference Finlay56]. It was developed as a result of modifications of the prototype unit LapaRobot that was designed and built in 1992 [Reference Dowler and Holland57, Reference Finlay and Ornstein58]. The prototype version had only 3 DOFs corresponding to pan, tilt, and zoom motions. However, the newer version Endosista has a larger operating envelope and an extra DOF for achieving the swivel motion that is rotation about its own axis. This additional DOF is required for oblique-viewing laparoscopes [Reference Finlay and Ornstein58]. Endosista was tested on a phantom, then after ethical committee approval and informed consent of patients, it was used clinically and proved its applicability [Reference Ornstein55].
Endosista’s commercial version was then introduced in 1998 [Reference Bihlmaier11], the EndoAssist laparoscopic holder from Armstrong Healthcare Limited [Reference Gilbert59]. It is activated by foot pedal and controlled by the surgeon’s head movements where the movements will only take place when the foot pedal is pressed to avoid any unwanted movements of the laparoscope. Head movements of the surgeon are detected by an infrared headset.
Freehand from Freehand Ltd. was considered a successor to the EndoAssist. It followed its operation method, where it was also controlled by the surgeon’s head movements detected by an infrared headset and activated only when a foot pedal was pressed [Reference Rane, Eddy, Kommu, Anderson and Rimington60, Reference Stolzenburg, Franz, Kallidonis, Minh, Dietel, Hicks, Nicolaus, Al-Aown and Liatsikos61]. Its small, light-weight, and simple design, that includes three independent axes, offered simplified control, and has made it advantageous in laparoscopic surgeries [Reference Finlay62, Reference Sapalidis, Michalopoulos, Mantalovas and Kesisoglou63].
Emaro, the world’s first pneumatically driven laparoscope holder robot with smooth operation [Reference Yoshida, Maruyama, Takahashi, Matsukuma and Kohnoe64] was launched as a result of a venture originating in universities [Reference Kawashima, Kanno and Tadano10, Reference Tadano and Kawashima65]. The robotic arm is a parallel manipulator with pneumatic driving mechanism that has made Emaro’s movements smooth and gentle, in addition to keeping the design small and simple. Instead of using external sensors that require a clear uninterrupted space between the transmitter and the receiver, two gyroscopes are attached to the surgeon’s head and body. The tracking accuracy of Emaro robot was first confirmed experimentally by Tadano and Kawashima [Reference Tadano and Kawashima65], proving that it had sufficient dynamic characteristics, allowing it to quickly follow the surgeon’s head movements.
Instruments tracking
Visual tracking of the surgical instruments is a more intelligent solution to maneuver the laparoscope compared to the voice and head controlled that require direct commands. The laparoscope is automatically displaced to follow areas of interest, marked by the surgical instruments. Surgical instruments are tracked by identifying significant features in them like color, shape, texture, and movement. To enhance the object tracking process, some studies attached distinguishing colored markers to the instruments [Reference Zhang and Payandeh66, Reference Zhao67]. Kim et al. [Reference Kim, Lee, Ko, Kwon and Lee68, Reference Lee, Kim and Ko69] designed an instrument tracking laparoscope holder “KaLAR” with a bending mechanism enabling it to adjust viewing angles within a compact design. It can track the instrument ‘s tip identified by colored markers, adjust these views according to voice commands, and switch between the two control modes by simple “Track” and “Voice” commands. Its original version was designed for laparoscopic cholecystectomy. In order to apply it to general laparoscopy, a modified version was developed with a passive base, 2 DOF manipulator that is the lower part of
$MC^{2}E$
robot [Reference Zemiti, Morel, Ortmaier and Bonnet70] and the same bending laparoscope [Reference Saing, Sotthivirat, Vilasrussamee and Suthakorn6, Reference Shin, Ko and Kwon71].
However, sterilization of the colored marker would be a problem in clinical use [Reference Khoiy, Mirbagheri and Farahmand72]. Therefore, several studies proposed marker-free tracking frameworks, which could detect and locate the surgical instrument based solely on its features [Reference Climent and Mares73–Reference Voros, Long and Cinquin77].
Learning-based systems have been beneficial to the marker-free instrument tracking process. Detection/segmentation of surgical instruments based on Machine Learning (ML) methods relied highly on hand-crafted features of the instrument [Reference Zhang and Gao78]. Where, feature descriptors such as Histogram of oriented Gradients (HoG) [Reference Wesierski, Wojdyga and Jezierska79], region Covariance [Reference Reiter, Allen and Zhao80], and Scale Invariant Feature Transform (SIFT) [Reference Allan, Thompson, Clarkson, Ourselin, Hawkes, Kelly and Stoyanov81, Reference Du, Allan, Dore, Ourselin, Hawkes, Kelly and Stoyanov82] were used to distinguish surgical instruments from the background. However, due to complications in the surgical environment such as smoke, shadows/reflections, body fluid, and the dynamic nature of background tissues [Reference Islam, Atputharuban, Ramesh and Ren83], these hand-crafted features were not robust in detection/segmentation of surgical instruments. Deep learning (DL) on the other hand can automatically extract robust image figures using well-trained convolutional neural networks (CNNs) and has shown potential in detecting/segmenting marker-free surgical instruments [Reference Zhang and Gao78, Reference Islam, Atputharuban, Ramesh and Ren83–Reference Chen, Zhao and Cheng86]. There are mostly two types of studies for surgical instruments tracking using DL [Reference Islam, Atputharuban, Ramesh and Ren83], tracking by detection using bounding box [Reference Zhao, Voros, Weng, Chang and Li84, Reference Chen, Zhao and Cheng86, Reference Zhao, Cai, Chang and Cheng87], and tracking by segmentation [Reference Zhang and Gao78, Reference Islam, Atputharuban, Ramesh and Ren83, Reference Garcia-Peraza-Herrera, Gruijthuijsen, Borghesan, Reynaerts, Deprest, Ourselin, Vercauteren and Poorten88, Reference Garcia-Peraza-Herrera, Li, Fidon, Gruijthuijsen, Devreker, Attilakos, Deprest, Poorten, Stoyanov and Vercauteren89]. In the bounding detection method, the surgical instrument is depicted by some special image features and its position is represented by a rectangle bounding box [Reference Zhao, Voros, Weng, Chang and Li84]. However, affected by the angle of imaging, there would be a large locating error if the end effector has occupied a small fraction of the rectangular bounding box [Reference Zhang and Gao78]. In tracking by segmentation, the instruments can be annotated into binary (where every pixel in an image is labeled as background or instrument), parts (background, shaft, wrist, or end-effector), and categories (according to the instrument type) [Reference Islam, Atputharuban, Ramesh and Ren83]. ToolNet [Reference Garcia-Peraza-Herrera, Li, Fidon, Gruijthuijsen, Devreker, Attilakos, Deprest, Poorten, Stoyanov and Vercauteren89], a holistically nested real-time instrument segmentation approach of robotic surgical instrument focused only on binary segmentation and has shown robustness and generalization ability for robotic instrument segmentation. Shvets et al. [Reference Shvets, Rakhlin, Kalinin and Iglovikov90] segmented the instruments into binary, parts, and categories and the model has obtained better accuracy compared with other segmentation models [Reference Islam, Atputharuban, Ramesh and Ren83, Reference Shvets, Rakhlin, Kalinin and Iglovikov90]. Moreover, Islam et al. [Reference Islam, Atputharuban, Ramesh and Ren83] proposed a CNN model with adversarial scheme for real-time surgical instruments segmentation from high-resolution videos. They also segmented the instruments into binary, parts, and categories. The model surpassed performance of previous work on the MICCAI robotic instrument segmentation challenge 2017 [91] in each category of segmentation. Furthermore, Zhang and Gao [Reference Zhang and Gao78] proposed a marker-free surgical instrument tracking framework based on object extraction via DL. The segmentation model was trained to extract the end-effector and the shaft portions of the surgical instrument in real time, and the surgical instrument joint was defined as the tracking point instead of the instrument’s tip. The framework achieved accurate and fast tracking of the surgical instrument in laparoscopic videos. A novel system presented in ref. [Reference Garcia-Peraza-Herrera, Gruijthuijsen, Borghesan, Reynaerts, Deprest, Ourselin, Vercauteren and Poorten88] allows the surgeon to perform bimanual coordination and navigation task while a robotic arm autonomously positions the laparoscope. Using a novel tooltip detection method and a new visual servoing approach for a generalized laparoscope model with support for RCM and laparoscope bending. The tooltip localization method is based on a hybrid mixture of DL and classical computer vision that ensures smooth and accurate motion of the laparoscope.
In addition to visually tracking the instruments, Eslamian et al. [Reference Eslamian, Reisner, King and Pandya92] proposed an instrument-tracking laparoscope algorithm implemented on da Vinci robot platform, where joint values of the instruments’ manipulators are captured and used to set the desired joint values for the laparoscope manipulator that will enable it to point toward the centroid of the instruments.
Cognitive laparoscope positioners
Furthermore, laparoscope positioning systems that can learn/predict the desired field of view, instead of just following the surgical instruments in the field of view can offer real autonomy. In [Reference Weede, Mönnich, Müller and Wörn93], traditional tracking methods are modified with prediction of the surgical instrument’s motion using Markov chains based on the collected information from former interventions. KaLAR can also distinguish the procedure in progress and recognize the optimal view corresponding to each procedure, after having preliminary knowledge of the surgical procedure loaded from the database [Reference Ko and Kwon94]. A cognitive laparoscope guidance system that learns the human assistant’s surgical knowledge about suitable laparoscope position using a supervised ML algorithm is presented in ref. [Reference Bihlmaier and Worn95]. The system utilizes a learned knowledge-based control mechanism, where spatial surgical knowledge is extracted and learned from recorded interventions. Moreover, [Reference Ji, Krishnan, Patel, Fer and Goldberg96, Reference Rivas-Blanco, Perez-del-Pulgar, López-Casado, Bauzano and Muñoz97] explored using learning from demonstration (LFD) to guide the laparoscope during surgery. LFD allows the robot to observe how a human performs a task then it autonomously imitates the human behavior to complete the same task. In ref. [Reference Rivas-Blanco, Perez-del-Pulgar, López-Casado, Bauzano and Muñoz97], the system stores the laparoscope position and its relation to the position of surgical instruments, teaching the system how the laparoscope moves depending on the instrument’s position. Ji et al. [Reference Ji, Krishnan, Patel, Fer and Goldberg96] focused on learning viewpoint selection problem based on anatomical features, not the instrument’s position.
Key features of famous laparoscope positioning robots are summarized in Table III along with representative figures.
Table III. Comparison of laparoscope positioning robots. The table shows key features of the systems including degrees of freedom, weight, control method, FDA approval, and fixture type in the operating room.
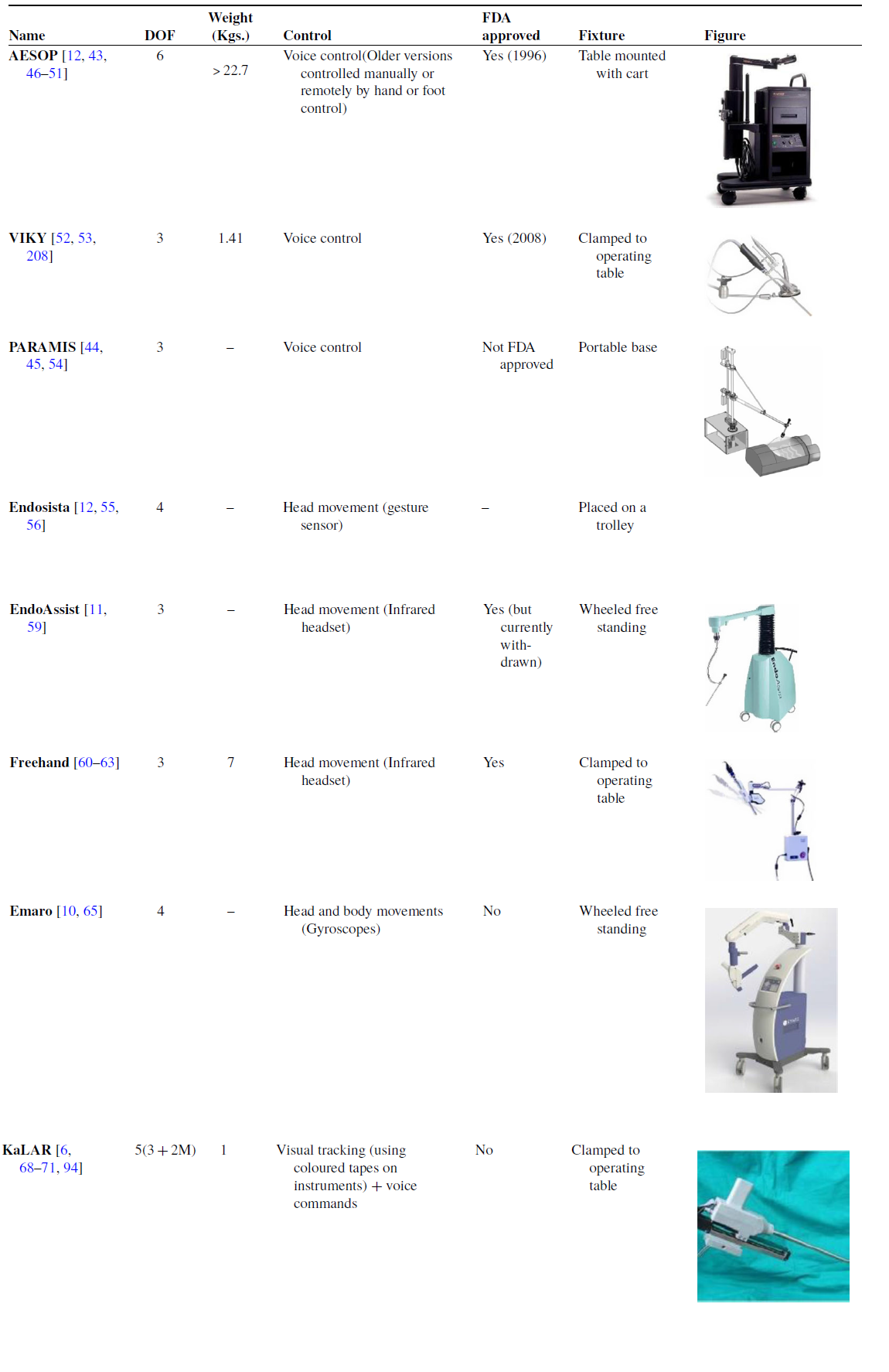
2.2.3. Master–slave teleoperated systems with dedicated consoles
Master–slave tele-operated systems with Dedicated Consoles (MSTDC) have become very common in laparoscopic surgeries [Reference Kalan, Chauhan, Coelho, Orvieto, Camacho, Palmer and Patel9, Reference Berkelman and Ma98–Reference Suzuki, Aoki, Kobayashi, Tsuji, Konishi, Hashizume, Sakuma, Arai and Arai, eds.)105]. They offer significant advantages, including eliminating the surgeon’s hand tremor and reducing his/her fatigue in dealing with restricted area of operation, due to limited DOFs provided by the trocar. The surgeon controls the laparoscope and the surgical instruments using a master device at a separate dedicated console while monitoring the surgical view. A slave robot at the patient side maps the master’s movements and manipulates the laparoscope and the instruments following the surgeon’s lead [Reference Ma and Berkelman101, Reference Pan, Qu, Ai, Fu and Liu106]. The concept of master–slave tele-manipulation system was introduced in the early 1990’s, where it was urged by the goal to perform surgical procedures on patients from remote places, like battlefields or outer space [Reference Ruurda, Van Vroonhoven and Broeders107]. Several systems are now available with different configurations regarding the surgeon console (including hand controllers and vision display), patient console, and endoscope control.
The first two MSTDCs to be commercialized were Zeus by Computer Motion, introduced in 1998 [Reference Kalan, Chauhan, Coelho, Orvieto, Camacho, Palmer and Patel9, Reference Marescaux and Rubino102, Reference Eto and Naito108], and the da Vinci surgical system by Intuitive Surgical. Zeus was initially developed for cardiac operations [Reference Boyd, Kiaii, Kodera, Rayman, Abu-Khudair, Fazel, Dobkowski, Ganapathy, Jablonsky and Novick109–Reference Reichenspurner, Damiano, Mack, Boehm, Gulbins, Detter, Meiser, Ellgass and Reichart113]. However, several clinical trials have been reported using it in abdominal surgery [Reference Goh, Lomanto and So114–Reference Zhou, Yu, Li, Bao, Liu, Zhang, Ren, Wei, Zheng and Huang118], gynecology [Reference Falcone, Goldberg, Garcia-Ruiz, Margossian and Stevens119, Reference Falcone, Goldberg, Margossian and Stevens120], and urology [Reference Guillonneau, CappÈLe, Martinez, Navarra and Vallancien121, Reference Guillonneau, Jayet, Tewari and Vallancien122]. The da Vinci was the first to gain FDA approval in 2000 followed by Zeus in 2001 [Reference Kalan, Chauhan, Coelho, Orvieto, Camacho, Palmer and Patel9, Reference Rao104, Reference Yates, Vaessen and Roupret123, Reference Palep124]. After Intuitive Surgical had bought out Computer Motion and discontinued the sale of Zeus system [Reference Longmore, Naik and Gargiulo17], the da Vinci system monopolized the market of MSTDC, with different versions for multi-port laparoscopic surgeries including da Vinci SI, da Vinci XI, and da Vinci X [Reference Rao104]. The da Vinci family has become well adapted for cardiac, urologic, gynecologic, pediatric, and general surgeries [Reference Koh, Jang, Park, Ham, Han, Rha and Choi125].
With the expiry of some key patents of Intuitive Surgical in 2019, competitors were allowed to adopt their technologies, introduce new robotic platforms, and apply for approval for clinical use [Reference Cisu, Crocerossa, Carbonara, Porpiglia and Autorino16, Reference Rassweiler, Autorino, Klein, Mottrie, Goezen, Stolzenburg, Rha, Schurr, Kaouk and Patel126]. The Senhance system from Transenterix Surgical Inc. initially named “ALF_X” became FDA approved in 2017 [Reference Rao104, Reference Gueli, Perrone, Cianci, Rossitto, Monterossi, Bernardini and Scambia127, Reference Hutchins, Manson, Lerebours, Farjat, Cox, Mann and Zani128], making it the only market cleared competitor with Intuitive Surgical in the USA [Reference Cisu, Crocerossa, Carbonara, Porpiglia and Autorino16]. It has several unique features compared to da Vinci including haptic feedback, gaze control of the endoscope, and independent robotic arms capable of holding reusable laparoscopic instruments. Moreover, Transenterix recently received 510 (k) clearance for its machine vision system which will automatically move the laparoscope according to instrument movements and recognition of learned landmarks in the field of view [Reference Cisu, Crocerossa, Carbonara, Porpiglia and Autorino16, 129].
The REVO-I system was developed in 2015 and has received Ministry of Food and Drug Safety (MFDS) approval. Its configuration is similar to that of da Vinci including single patient cart with 4 arms, 3D stereoscopic display, and similar hand controls at the surgeon’s console [Reference Rao104, Reference Brodie and Vasdev130].
Versius system that was unveiled by CMR Surgical in 2018 is not yet FDA approved but has received Therapeutic Goods Administration (TGA) approval for use in Australia [Reference Cisu, Crocerossa, Carbonara, Porpiglia and Autorino16]. The system’s slave arms are human-like robotic arms, enabling them to hold the laparoscopic instrument more like how a surgeon would hold it, thanks to the multiple wrist
$\mathrm{V}-\text{wrist}^{\mathrm{TM}}$
technology (as named by CMR surgical). The robotic arms are of relatively small size compared to other systems, with a footprint of only 38 cm x 38 cm each, offering an easy setup [Reference Nakadate, Hashizume and Sanchez-Margallo, ed.) (IntechOpen103].
Avatera system by Avateramedical (Jena, Germany) has been in development since 2011 and has received Conformité Européenne (CE) certification in 2019 [Reference Cisu, Crocerossa, Carbonara, Porpiglia and Autorino16]. It shares a similar configuration to the da Vinci multi-port family [Reference Franz, Rassweiler, Liatsikos, Kyriazis, Bach, Siemer, Yanev and Stolzenburg131]; however, it uses single-use disposable instruments [Reference Koukourikis and Rha18]. Mirosurge system was developed by the DLR Institute of Robotics and Mechatronics in Germany with the MIRO robotic arm as its main component [Reference Alabdulaali and Rha132]. The system is considered versatile as it fulfils the requirements of a broad range of surgical applications and enables the implementaion of different control modes [Reference Hagn, Konietschke, Tobergte, Nickl, Jörg, Kübler, Passig, Gröger, Fröhlich and Seibold133]. Since all joint units of MIRO integrate both position and torque sensors, it can be used in impedance-controlled mode. Therefore, a soft (“hands on”) robotics feature is integrated into the system’s workflow, allowing sensitive movements of the robot affected by the surgeon. In addition, the end-effector’s orientation remains constant due to programmed high stiffness, enabling the surgeon to easily insert the surgical instrument into the patient by touching and moving the robotic arm [Reference Konietschke, Hagn, Nickl, Jorg, Tobergte, Passig, Seibold, Le-Tien, Kubler and Groger134]. Medtronic (Dublin, Ireland) has been developing Hugo system since it acquired Mirosurge system in 2013 [Reference Cisu, Crocerossa, Carbonara, Porpiglia and Autorino16, Reference Gözen and Rassweiler135]. Hugo was unveiled in 2019 [Reference Hu and Shoag136] and is expected to receive CE in 2021 [Reference Gözen and Rassweiler135]. It is unique for its modular, wheeled design, and cost-effectiveness [Reference Cisu, Crocerossa, Carbonara, Porpiglia and Autorino16]. Medicaroid Cooperation (Kobe, Japan) was established in 2013 as a result of collaboration between Kawasaki a Japanese Industrial Robotics Company and Sysmex a Medical Manufacturer [Reference Cisu, Crocerossa, Carbonara, Porpiglia and Autorino16]. It developed Hinotori system that has recently (August 2020) received regulatory approval from the Japanese Ministry of Health, Labor, and Welfare [Reference Koukourikis and Rha18]. In 2013, Central South University in collaboration with Tianjin University developed Micro hand S system [Reference Yi, Wang, Li, Jiang, Son, Su and Zhu137, Reference Yi, Wang, Li, Jiang, Son, Su, Zhu and Wang138]. The system’s slave manipulator is based on the folding principle of the laminated double parallelogram mechanism allowing it to have a compact design and wide range of motions [Reference Li, Zhu, Juan and Yi139, Reference Trastulli, Coratti, Guarino, Piagnerelli, Annecchiarico, Coratti, Di Marino, Ricci, Desiderio and Cirocchi140].
Moreover, several systems have been developed for single port laparoscopic syrgery, such as the da Vinci SP [Reference Kaouk and Bertolo141–Reference Shin, Yoo, Lee, Lee, Jeong and Moon143], the SPORT surgical system [Reference Barret144–Reference Peters, Armijo, Krause, Choudhury and Oleynikov147], and SPRINT system [Reference Piccigallo, Scarfogliero, Quaglia, Petroni, Valdastri, Menciassi and Dario148–Reference Scarfogliero, Quaglia, Piccigallo, Tognarelli, Valdastri, Menciassi and Dario151]. They all utilize a single robotic arm, with the instruments having additional articulated joints for enhanced dexterity within a single incision [Reference Longmore, Naik and Gargiulo17].
Table IV summarizes comparison points of MSTDCs along with representative figures in Table V.
Table IV. Comparison of Master–Slave Teleoperated surgical systems with Dedicated Consoles (MSTDCs), showing differences in their structures, type of approval received, and the manufacturer of each system.
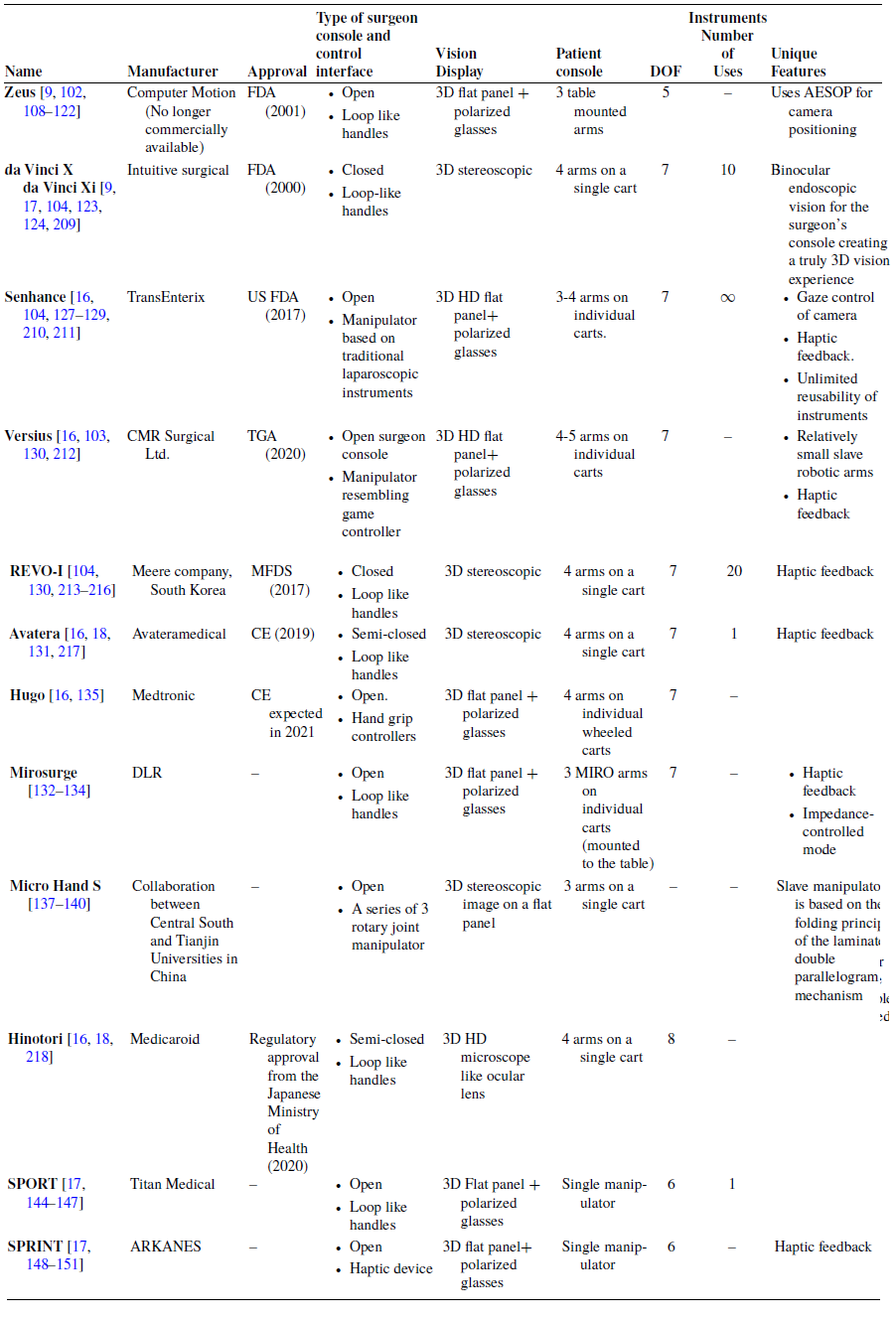
Table V. Representative figures of MSTDCs.

2.2.4. Robotic training systems
Traditionally, surgical training was carried out by observational learning, and refining the surgical skills by practice during live operations under experts’ supervision. However, this process is considered time consuming and risky. An alternative to this risk of training under real-life situation is the use of simulated surgical environments, such as physical box trainers [Reference Derossis, Bothwell, Sigman and Fried152], virtual reality [Reference Alaker, Wynn and Arulampalam153–Reference Yiannakopoulou, Nikiteas, Perrea and Tsigris156], and augmented reality simulators [Reference Abboudi, Khan, Aboumarzouk, Guru, Challacombe, Dasgupta and Ahmed157, Reference Varras, Nikiteas, Varra, Varra, Georgiou and Loukas158]. However, none of these solutions mimic the conventional hand-over-hand guidance that surgeons use to teach their trainees.
Robotic training systems can actively teach trainees the skilled motion of master surgeons and evaluate the trainee’s performance by comparing his/her motion with that of the master surgeon [Reference Chui, Chng, Yang, Wen, Huang, Liu, Su and Chang159]. The expert surgeon’s manipulation of the surgical instrument is either recorded and played back to the surgical trainee, or using a master–slave system, the expert surgeon remotely instructs surgical trainees by manipulating the master robot that is imitated by the slave robot holding the trainee’s laparoscopic instrument [Reference Chui, Chng, Yang, Wen, Huang, Liu, Su and Chang159–Reference Prince, Kang, Simonelli, Lee, Gerber, Lim, Chu, Dutson and Tsao163].
Chui et al. [Reference Chui, Chng, Yang, Wen, Huang, Liu, Su and Chang159] proposed a robotic box trainer with a 5 DOF manipulator that can record, play back movements carried out by the master surgeon, guide the trainee, and evaluate his/her performance. In record mode, the master surgeon performs surgery while his/her manipulation of the instrument is tracked (using cameras to acquire the 3D coordinate location of the instrument) and recorded. In replay mode, the robot is in full control, driving the surgical instrument by mimicking the recorded trajectory and the trainee is holding onto the instrument’s handle to learn the master’s skilled motion. In the guiding mode, the robot guides the trainee to follow the recorded trajectory by preventing his/her motion form varying excessively from that of the master’s using force feedback. In the evaluation mode, the trainee performs the surgical tasks while the motion of the surgical instrument is being recorded and compared with that of the surgeon.
Other systems record the manipulator’s joints’ angles during the record stage. Garudeswaran et al. [Reference Garudeswaran, Cho, Ohu and Panahi161] proposed a 6 DOF robotic arm, with its servomotors acting as potentiometers during the recording stage when they are not energized. The expert surgeon manipulates the surgical instrument, moving the robotic arm. The joints’ angles are recorded, stored, and then played back to the servomotors in the training stage. A system of two surgical manipulators with 5 DOF each, providing a similar range of motion as in conventional laparoscopic instruments, is proposed by Lee et al. [Reference Lee, Yang, Yang, Chui, Liu, Huang, Su and Chang162]. With one manipulator being controlled by the surgeon, recording joints’ angles measured by encoders, and the other follows its recorded movements to guide the trainee. Abdelaal et al. [Reference Abdelaal, Sakr, Avinash, Mohammed, Bajwa, Sahni, Hor, Fels and Salcudean160] proposed a record/playback system that utilizes the tele-operated da Vinci surgical system as the training platform. The proposed system records joint angles of both the master tool manipulators (MTMs) and the patient side manipulators (PSMs) during the performance of a tele-operated laparoscopic surgery by an expert surgeon. These motions are then played back to either the MTMs for robotic laparoscopic surgery training or the PSMs for conventional laparoscopic surgery training.
A more recent work by Prince et al. [Reference Prince, Kang, Simonelli, Lee, Gerber, Lim, Chu, Dutson and Tsao163] proposed the LapaRobot, a laparoscopic surgical system for tele-mentoring training, composed of an expert station and a trainee station. The joints’ angles in the expert station are measured by optical encoders and sent as joint commands to the trainee station. The expert and trainee stations can also be used as standalone record/playback devices without tele-operation.
Features of developed robotic training systems are summarized in Table VI.
Table VI. Summary of robotic training systems, including key features and experimental results.

2.3. Classification based on configuration
Generally, laparoscopic manipulators which exist in clinical use can be classified into two main categories: serial manipulators and parallel manipulators.
2.3.1. Serial manipulators
Surgical serial manipulators depend on wires or rod and gear-based systems to transmit the mechanical movement to the robot joints since no actuators are allowed to be inside the patient body. Most of the commercially available MSTDCs utilize serial manipulators, such as da Vinci, Senhance, REVO-I, Avatera, and Hinotori [Reference Cisu, Crocerossa, Carbonara, Porpiglia and Autorino16, Reference Koukourikis and Rha18, Reference Rao104, Reference Gueli, Perrone, Cianci, Rossitto, Monterossi, Bernardini and Scambia127, Reference Guthart and Salisbury164, Reference Khandalavala, Shimon, Flores, Armijo and Oleynikov165]. In addition to several laparoscope positioners including the AESOP [Reference Kraft, Jäger, Kraft, Leibl and Bittner43], Endosista [Reference Jaspers, Breedveld, Herder and Grimbergen12], Endoassist [Reference Gilbert59], and Freehand [Reference Rane, Eddy, Kommu, Anderson and Rimington60, Reference Stolzenburg, Franz, Kallidonis, Minh, Dietel, Hicks, Nicolaus, Al-Aown and Liatsikos61]. Some handheld devices also have serial configuration [Reference Focacci, Piccigallo, Tonet, Megali, Pietrabissa and Dario22, Reference Feng, Yang, Zhang, Li, Chen, Yan, Du and Wang33]. A number of serial laparoscopic robots have spherical configuration to mechanically constrain the instrument with respect to the incision, but this may come with a tradeoff of inadequate workspace. To solve this problem, a redundant serial spherical multi-robot system was proposed in ref. [Reference Nelson, Laribi and Zeghloul166]. Moreover, snake-like wire-driven devices are proposed, where a snake-like device with multi-backbone has been introduced by Simaan et al. [Reference Simaan, Taylor and Flint167], with three super elastic wires responsible for performing pull and push modes.
However, wire-driven laparoscopic manipulators have many downsides. The wires might rupture during the operation, or be slacked, requiring pre-tensioning mechanism [Reference Khalifa, Fanni, Mohamed and Miyashita168]. In addition, the sterilization process may also be troublesome. Therefore, researchers have started to propose parallel manipulators that offer further advantages over the serial manipulators.
2.3.2. Parallel manipulators
Parallel manipulators are considered closed-chain mechanisms that have a movable platform connected with the fixed base platform through several independent kinematic chains. This configuration has offered multiple advantages over the serial configuration, including higher structural stiffness and payload capacity, accurate positioning with high speed, and easy sterilization process.
Several designs of laparoscopic parallel manipulators have been proposed in the last decade, including laparoscope positioners such as the voice-controlled parallel manipulator PARAMIS [Reference Pisla, Plitea, Vaida, Hesselbach, Raatz, Vlad, Graur, Gyurka, Gherman and Suciu44, Reference Vaida, Pisla, Plitea, Gherman, Gyurka, Stancel, Hesselbach, Raatz, Vlad and Graur45, Reference Plitea, Hesselbach, Vaida, Raatz, Pisla, Budde, Vlad, Burisch and Senner54], and the pneumatically driven laparoscope positioner Emaro [Reference Tadano and Kawashima65]. Parallel configuration has also been introduced in robotic forceps that can be used in handheld devices or teleoperated systems. A robotic forceps with complex rigid link mechanism has been developed by Arata et al., allowing bending in two directions and grasping. But the bending angle is limited to ± 70°. Yamashita et al. [Reference Yamashita, Iimura, Aoki, Suzuki, Nakazawa, Kobayashi, Hashizume, Sakuma and Dohi170] introduced another laparoscopic forceps manipulator with 2-DOFs bending mechanism by multi-slider linkage mechanisms and 1-DOF wire-driven grasping mechanism; however, troubles about precision and power consumption have been reported. Ishii et al. [Reference Ishii, Kobayashi, Kamei and Nishitani171] developed new dual screw drive (DSD) forceps, but the manufacturing accuracy needs to be improved. A novel actuator driven handheld laparoscopic instrument is introduced by Rose et al. [Reference Röse, Wohlleber, Kassner, Schlaak and Werthschützky172] having a parallel kinematic instrument tip to overcome the workspace restrictions of classic laparoscopic instruments. However, the largest bending angle of the manipulator is not equal in every direction because of the distinctive design of its limbs. A parallel hybrid surgical robot PARASURG-9 M is introduced by Pisla et al. [Reference Pisla, Gherman, Vaida, Suciu and Plitea173], consisting of a surgical robotic arm, PARASURG whose kinematics and singularity analysis are studied in refs. [Reference Pisla, Plitea, Gherman, Vaida, Pisla and Suciu174, Reference Pisla, Gherman, Vaida and Plitea175] and an active robotized surgical instrument PARASIM. A significant drawback is its limited range of the orientation angles. Elastostatic model of a novel hybrid laparoscopic robot has been introduced [Reference Tanev, Cammarata, Marano and Sinatra176], having two identical spherical-prismatic-universal (SPU) limbs and a 3 revolute (RRR) limb to enable spherical movements of the end effector. The robot possesses four active joints, 3 for the parallel mechanism and 1 for the translational movement along the axis of the surgical instrument. However, its maximum bending angle was ± 60° in any direction. A 4-DOF laparoscopic parallel manipulator has been designed and implemented [Reference Ibrahim, Ramadan, Fanni, Kobayashi, Abo-Ismail and Fujie177], with two prismatic-universal-universal (PUU) and two prismatic-universal-spherical (PUS) limbs, capable of reaching ± 90° in all directions. However, a thorough singularity analysis is required to discover why the movable platform could not resist forces in some configurations. Moreover, the kinematics of the general 3-prismatic-revolute-spherical (PRS) parallel mechanism that owns 2R1T DOFs is investigated by Li and Xu [Reference Li and Xu178]. Table VII shows kinematic structures of developed parallel manipulators along with their limitations. Novel laparoscopic parallel manipulator with 3-PUU architecture that also owns 2R1T DOFs is presented by Khalifa et al. [Reference Khalifa, Fanni, Mohamed and Miyashita168]. It can achieve a bending angle of more than ± 90° in any direction. Unlike the 3-PRS mechanism, this manipulator does not need precise, troublesome spherical joints. Therefore, from a practical point of view, 3-PUU manipulator owns better orientation capability and is also cheaper and easier to produce [Reference Khalifa, Fanni, Mohamed and Miyashita168].
Table VII. shows kinematic structures and degrees of freedom of different surgical parallel manipulators along with their reported drawbacks.
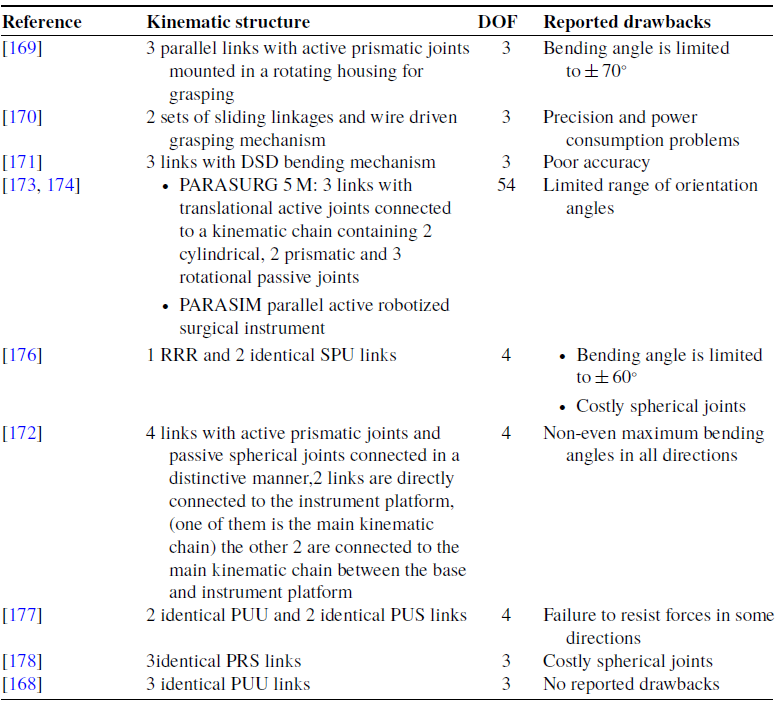
2.4. DOFs of laparoscopic robots
A minimum number of 3 DOFs is required to maneuver the laparoscope providing insertion/withdrawal, pitch, and yaw movements. Moreover, additional roll motion may be required to correct the top and bottom of image for forward-viewing laparoscope and to observe the back of the organs for oblique-viewing laparoscope [Reference Taniguchi, Nishikawa, Sekimoto, Kobayashi, Kazuhara, Ichihara, Kurashita, Takiguchi, Doki, Mori and Miyazaki14]. Furthermore, conventional laparoscopic instruments require 4 DOFs and an additional DOF if there is a grasping action [Reference Fu, Pan, Li and Wang179]. Developed laparoscope positioning robots offer a wide range of DOFs starting from 3 up to 6 DOFs [Reference Saing, Sotthivirat, Vilasrussamee and Suthakorn6, Reference Kawashima, Kanno and Tadano10, Reference Kraft, Jäger, Kraft, Leibl and Bittner43, Reference Voros, Haber, Menudet, Long and Cinquin53, Reference Plitea, Hesselbach, Vaida, Raatz, Pisla, Budde, Vlad, Burisch and Senner54, Reference Shin, Ko and Kwon71]. Additionally, a robotic system developed by ref. [Reference Petani, Scheikl and Mathis-Ullrich180] for positioning oblique-viewing laparoscopes has 8 DOFs including the Franka Emika Panda manipulator (7 DOFs) and a rigid 30 degree oblique-viewing laparoscope with mono vision (1 DOF).
Industrial master–slave systems provide the surgeon with either 5,6,7, or 8 DOFs [Reference Kalan, Chauhan, Coelho, Orvieto, Camacho, Palmer and Patel9, Reference Cisu, Crocerossa, Carbonara, Porpiglia and Autorino16, Reference Eto and Naito108, Reference Brodie and Vasdev130]. Handheld devices also offer the surgeon enhanced dexterity with 5 and 7 DOFs [Reference Sánchez-Margallo, Sánchez-Margallo and Szold20, Reference Bensignor, Morel, Reversat, Fuks and Gayet21]. Published works of robotic training systems proposed a variety of 5 to 7 DOFs robotic trainers [Reference Chui, Chng, Yang, Wen, Huang, Liu, Su and Chang159–Reference Lee, Yang, Yang, Chui, Liu, Huang, Su and Chang162]. Figure 3 classifies laparoscopic robots according to their DOFs.

Figure 3. Shows degrees of freedom of conventional laparoscopic robots.
2.5. Classification based on the control technique employed in the system
Further to the above subdivision, Fig. 4 classifies laparoscopic robots according to their type of control technique used as being open or closed loop. All systems have visual feedback to the surgeon. However, systems are considered to have closed loop control if the robot’s path is continuously monitored and adjusted according to feedback of the desired position. Therefore, gaze/instrument tracking, cognitive laparoscope positioning robots, MSTDCs, robotic training systems, and handheld systems with virtual fixtures exhibit closed-loop control. Moreover, open loop systems may use monitoring systems to evaluate their performance by measuring parameters such as instrument’s position and/or contact force in test platforms [Reference Bensignor, Morel, Reversat, Fuks and Gayet21, Reference Miyazaki, Hirose, Ishikawa, Kanno and Kawashima26, Reference Feng, Yang, Zhang, Li, Chen, Yan, Du and Wang33].

Figure 4. Classification of robots in laparoscopic surgery according to control type.
3. Generalized control block diagram of robots in laparoscopic surgeries
The color-coded generalized block diagram, shown in Fig. 5, demonstrates the general control scheme of robots used in laparoscopic surgeries following the subdivisions of the previous section.
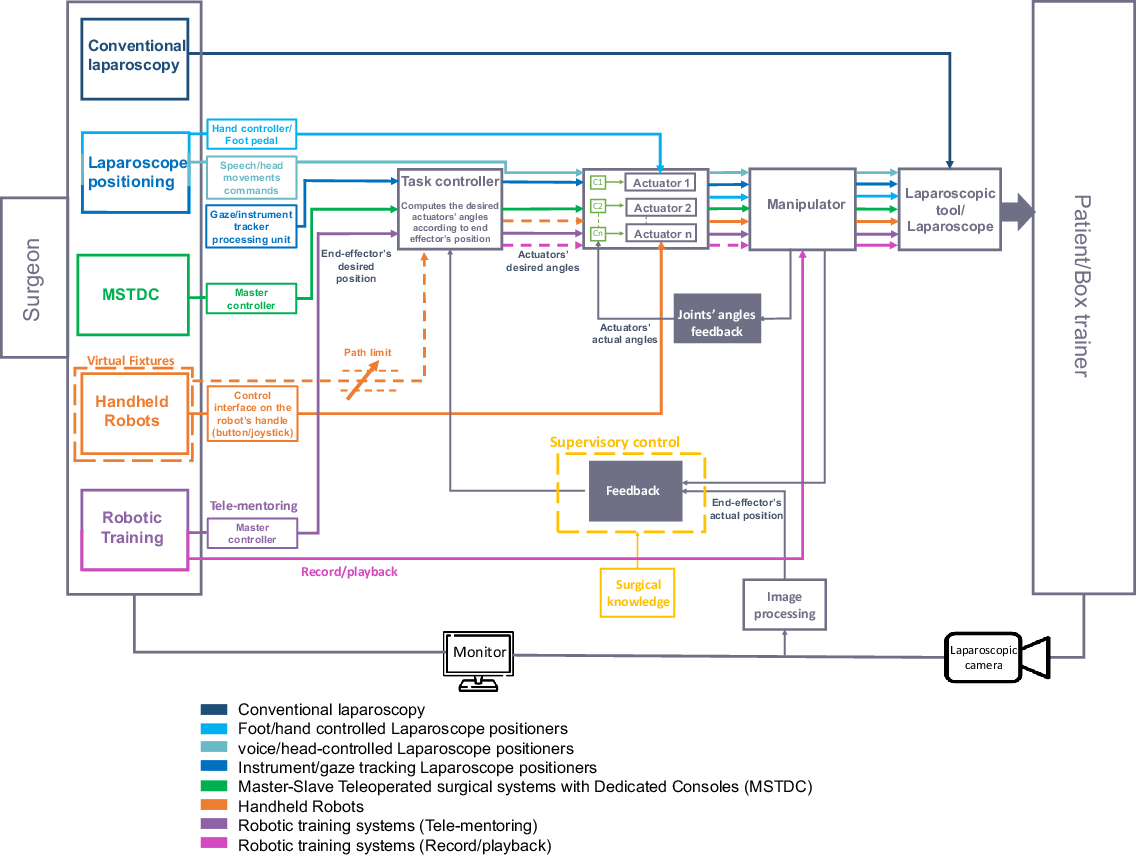
Figure 5. Generalized block diagram of robotic systems in laparoscopic surgery.
It can be easily seen from the block diagram that in conventional manual laparoscopy, the surgeon directly controls conventional laparoscopic instruments. Handheld robots allow the surgeon to directly control the actuators using control interface on the robot’s handle (button, joystick), enabling the robot to manipulate the articulated instrument’s tip in an open loop manner [Reference Payne and Yang19, Reference Sánchez-Margallo, Sánchez-Margallo and Szold20]. However, handheld robots with virtual fixtures provide closed loop control by limiting the instrument’s motion into restricted pre-defined region/path [Reference Colan, Nakanishi, Aoyama and Hasegawa37]. In manually (hand/foot) controlled laparoscope positioning robots, direct commands are sent to the manipulator’s actuators to position the laparoscope accordingly. While in the voice, head movements control, the actuators’ controllers (c1, c2… cn) send the required control signals to the actuators. These control signals are modified according to the joints’ angles feedback, i.e. closed loop motor control [Reference Vaida, Pisla, Plitea, Gherman, Gyurka, Stancel, Hesselbach, Raatz, Vlad and Graur45, Reference Tadano and Kawashima65]. However, there is no feedback that compares the actual end effector’s position with the desired position, that is why the whole system is considered an open loop system, although the actuators exhibit closed-loop control. In the eye gaze/instrument tracking, the end effector’s desired position is obtained from the image processing unit and is fed to the task controller, which in turn sends commands of the actuator’s required angles to the actuators’ controllers [Reference Li, Zhang, Xue, Kim and Zhang5, Reference Pandya, Reisner, King, Lucas, Composto, Klein and Ellis13, Reference Tzagkas, Gketsis, Stavrakakis, Zervakis and Hatzilias181]. These commands are modified according to feedback of the actual end effector’s position (in addition to the joints’ angles feedback). Furthermore, supervisory control can be achieved by providing laparoscopic robots with preliminary surgical knowledge such as the sequence of procedures, instruments used in each stage, and the preferred laparoscope view at each one. The robotic arm can estimate the current stage using surgical knowledge and information of surgical environment and suggests the proper laparoscope view corresponding to the estimated surgical stage [Reference Kim, Ko, Lee and Kwon182–Reference Kwon, Ko and Kim184].
In MSTDCs, the ergonomic handles (master controller) controlled by the surgeon provide the desired instrument position that is mapped to the slave’s workspace and sent to the task controller. The controller sends the required control effort to the slave’s actuators’ controllers which are modified according to feedback of the actual end effector’s position (in addition to the joints’ angles feedback) [Reference Ma and Berkelman101, Reference Pan, Qu, Ai, Fu and Liu106]. Moreover, bi-lateral control can be achieved if haptic feedback signal is sent from the slave to the master control unit [Reference Sarmah and Gulhane185, Reference van den Bedem, Hendrix, Rosielle, Steinbuch and Nijmeijer186].
For robotic training systems, the tele-mentoring mode operates in a similar manner to that of the MSTDC [Reference Prince, Kang, Simonelli, Lee, Gerber, Lim, Chu, Dutson and Tsao163]. The record/play training mode allows the surgeon to directly move the manipulator while manipulating the laparoscopic instrument. The instrument’s trajectory/video feedback/ manipulator’s joints angles are recorded and stored then the task controller sends the required control effort to the actuators’ controllers in the playback stage [Reference Abdelaal, Sakr, Avinash, Mohammed, Bajwa, Sahni, Hor, Fels and Salcudean160, Reference Garudeswaran, Cho, Ohu and Panahi161].
Further to the generalized control block diagram of laparoscopic robots, the following subsection discusses more detailed control strategies of selected laparoscopic robots in previous studies.
3.1. Technical control strategies of laparoscopic robots in selected previous studies
Paramis voice-controlled laparoscope positioning robot is composed of three system levels [Reference Vaida, Pisla, Plitea, Gherman, Gyurka, Stancel, Hesselbach, Raatz, Vlad and Graur45]: user interface (shown in green in Fig. 6), PLC (shown in blue), and the robot (shown in yellow). In the user interface level, the surgeon interacts with the robotic system through vocal command inputs that are processed using the voice commands module and translated into a given command for the controller level. In the PLC level, the actuation driver performs the connection between the motion elements and software application. The mathematical model provides the kinematic model of the robot to the path definition module that uses it to calculate the position variation for each actuator for a given command. Then, motion parameters are calculated in the positioning parameters calculation module based on the manipulator’s kinematics and its current position. The communication module ensures communication between the computer, PLC, and robot actuators based on CANOpen protocol. Finally, the robot level represents the mechanical structure and the actuators. The control module in the robot level calculates the position of the robot as a function of the parameters imposed by the voice commands and current position of each actuator obtained from position transducer.

Figure 6. Control block scheme of the actuation system for Paramis robot [Reference Vaida, Pisla, Plitea, Gherman, Gyurka, Stancel, Hesselbach, Raatz, Vlad and Graur45].
The 4-DOF head movements-controlled robot Emaro [Reference Tadano and Kawashima65] is controlled by first measuring the angular velocity (wh) and translational velocity (vh) using gyroscopes mounted on the surgeon’s head and body, respectively, as shown in Fig. 7. The reference angular velocity (wref) of the robotic arm is obtained by multiplying the angular velocity by the matrix that indicates the angular position of each rotational joint of the robot (R). Then the angular velocity of each rotational joint is obtained using the Jacobian matrix. Velocity of the axis responsible for the zoom motion is defined as the anteroposterior direction of vh. After obtaining velocities of all four joints of the robot, they are integrated to provide the reference position vector qref and the reference torque (Tref) is calculated using PD controller along with feed-forward compensation using inverse dynamics calculated from the reference position.

Figure 7. Control block diagram of Emaro robot [Reference Tadano and Kawashima65].
The control and interface architecture of the instrument tracking laparoscope holder KaLAR is presented in Fig. 8. The real-time surgical image is acquired from CCD camera and using the Meteor-II board from the Matrox Co. The image data is sent to the supervisory control system (that can distinguish the procedure in progress and recognize the optimal view corresponding to each procedure, after having preliminary knowledge of the surgical procedure loaded from the database) and to the surgeon/operator. This data is used to generate the desired path of the manipulator that is sent to the manipulator’s controller [Reference Shin, Ko and Kwon71, Reference Ko and Kwon94].

Figure 8. Control and interface architecture of KaLAR robot [Reference Shin, Ko and Kwon71].
A recent work proposed and simulated the application of Model Predictive Control (MPC) with fuzzy approximation for active RCM constraint (caused by the incision point) on the industrial serial 7-DOF robot KUKA LWR + used as a laparoscopic robot [Reference Su, Zhang, She, Zhang, Fan, Zhang, Liu, Ferrigno and De Momi187]. The control objective is to make the robot follow a desired trajectory while controlling the position and orientation of the surgical tool simultaneously. First, 2D trajectory tracking and RCM constraint for the surgical tool are considered. Modeling the surgical tool as a virtual dynamic system with virtual velocity along the two axes and virtual angular velocity around its end and transferring the trajectory tracking and RCM constraint problem into the control problem of the virtual system. Then MPC is applied to design the controller of the virtual dynamic system. Second, the 3D workspace of the robot is simplified, by projecting it into xy-plane and xz-plane, respectively, so the 2D MPC solution can be used to solve these two 2D problems. By solving the angle and angular velocity of each joint of the robot using inverse kinematics [Reference Hassan, El-Habrouk and Deghedie188], the robot can be controlled to get to the actual pose and realize the trajectory tracking and RCM constraint. Finally, the fuzzy approximation is introduced to compensate for the external disturbances introduced by the motion of organs during breathing. The control framework is shown in Fig. 9 [Reference Su, Zhang, She, Zhang, Fan, Zhang, Liu, Ferrigno and De Momi187].

Figure 9. Control framework of Model Predictive Control with fuzzy approximation for active RCM constraint [Reference Su, Zhang, She, Zhang, Fan, Zhang, Liu, Ferrigno and De Momi187].
A cognitive control architecture is proposed in ref. [Reference De Rossi, Minelli, Roin, Falezza, Sozzi, Ferraguti, Setti, Bonfè, Secchi and Muradore189] that integrates the appropriate surgical task determinism using supervisory controller, motion safety using velocity-constrained MPC, and adaptability to human task execution timings provided by action segmentation using multi-modal neural network. The system’s overall block diagram is shown in Fig. 10. The employed robotic system consists of the daVinci surgical system (tele-operated by the surgeon) along with the SARAS robotic arm (autonomous). The surgeon tele-operates the daVinci system and the AI module processes the cartesian poses of both the daVinci system and the SARAS arm using knowledge of the training data. Implementing the neural network EdSkResNet to integrate multi-modal learning over the data available during robotic laparoscopic surgery, action segmentation is achieved. After the action segmentation module estimates the current action and the confidence level, the supervisory controller determines the next task to be performed by the robot (the next goal position and confidence level). Finally, the MPC receives the current goal and confidence level required to control the SARAS arm, where it is intended to maximize the performance and the safety of the arm incorporating both a collision avoidance formulation and velocity modulation based on the uncertainty of the action segmentation module.

Figure 10. Control framework of a multi-modal learning system to control surgical assistant robots via action segmentation [Reference De Rossi, Minelli, Roin, Falezza, Sozzi, Ferraguti, Setti, Bonfè, Secchi and Muradore189].
In ref. [Reference Du, Liang, Yan, Sun and Chen190] a physical human-robot interaction (pHRI) control scheme of a haptic 9-DOF master manipulator used in laparoscopic robots is presented for enhancing the precision and comfort of operations. A sensor-less force control scheme based on torque pattern is proposed to estimate the complete dynamic information without explicit acceleration calculation. To further improve the performance of the proposed system, a compensator based on time-delay neural network (TDNN) is introduced. First, the inverse dynamics equations of the manipulator are derived, and a joint torque observer based on the generalized momentum is derived from the manipulator’s dynamic model. To reduce the residual error between the output of the observer and the actual data, multilayer perception (MLP) networks have been used, but they are unable to compensate for the inertia moment calculation error which will impact the performance of the pHRI. Since the inertia moment can be obtained without explicit acceleration calculation by using the angular velocity information and its filtered value which is obtained using the current and previous velocity information, TDNN is used to add a delay operator to the MLP allowing the network to have a finite dynamic response to time series data. Finally, comparative experiments were conducted by the simulation to verify that the proposed compensator can fit the nonlinear function of the system. The framework of the proposed pHRI control scheme is presented in ref. [Reference Du, Liang, Yan, Sun and Chen190].
A recent study discussed perception, control, and manipulation of robotic laparoscopic tools with emphasis given to the pivot trajectories and RCM constrained motion planning [Reference K191] using the 7-DOF KUKA manipulator and the 3-fingered Barrett hand gripper attached to it for grasping purposes. The robotic arm is controlled to be able to detect, grasp, and manipulate a laparoscopic surgical tool. To achieve this goal, the robot should be capable of visually detecting the scene and the laparoscopic tools then calculating the relative position and orientation of the center of mass of each tool, calculating the contact points on the tool, on which the fingers of the gripper will be placed to make a firm grasp, calculating the path from the tool’s table to the surgical site table, and finally calculating the required trajectory to be executed when the tool is inserted into the trocar. Several control system schemes were studied: RCM tracking, visual servoing, and firm grasping algorithm and force control. In the RCM tracking method, the RCM error is calculated and used as a feedback signal in a control system that makes sure that the laparoscopic tool is always aligned with the fulcrum point and satisfies the RCM constraint. Determining the line of the long axis of the laparoscopic tool and the position and orientation of the fulcrum reference frame, the alignment error is calculated as the distance of the line from the fulcrum point. Using the calculated RCM error and the estimated position of the origin of the fulcrum reference frame, an adaptive motion control system is implemented to correct the trajectory and to avoid RCM misalignment. The RCM tracking control system is shown in Fig. 11 [Reference K191]. Two visual servoing control system schemes were implemented: position-based servoing and image-based servoing. In the position-based servoing, depth information is extracted from motion, stereo vision, or 3D sensors, from which the desired position is calculated and used as the reference input in the closed-loop control system shown in Fig. 12. In the image-based servoing, the robot is directly controlled using features extracted from the images and positions on the image plane. The robot is driven so that the video frame is changed from an initial view to a final desired view. The image-based visual servoing closed loop control is shown in Fig. 13. Where the image controller is a PD controller that outputs commands to control the robot in task space (and the internal robot controller drives each joint to the desired angle). The computer vision feature extraction calculates the vector from the detected tool’s center of mass to the center of image frame which is used to calculate the error for the controller. The firm grasping algorithm and force control combine position measurements with force measurements to ensure that the gripper firmly grasps the surgical tool. The position of the finger of the Barrett hand is known using the forward kinematics and the forces are measured using tactile sensors. According to the block diagram shown in Fig. 14, the force error calculated using a reference force and the measured force produces a position error via the first PD controller. This position error is added to the current measured position and will make the gripper’s finger move closer to (if the error is positive) or away (if the error is negative) from the tool. This loop is repeated until satisfactory contact is reached, and the desired force is exerted on the tool.

Figure 11. Control framework based on RCM tracking [Reference K191].

Figure 12. Control framework using position based servoing [Reference K191].

Figure 13. Control framework using image based servoing [Reference K191].

Figure 14. Firm grasping algorithm and force control block diagram [Reference K191].
A control strategy for RCM and direct teaching is proposed in ref. [Reference Kim, Zhang and Jin192] that enables the surgical robot to mimic the movements of a nurse’s wrist. The assistant robot has an end-effector attached to a 6-joint cooperative robot that holds the laparoscope. To maintain a non-mechanical remote center, force/torque applied by the surgeon is measured using a force/torque sensor attached between the robot and the end-effector and is then converted into a control command. Control objective is to maintain a solid remote center even while the end-effector’s posture is operated with direct teaching. The control block diagram of the system is shown in Fig. 15. The position control inputs are the predefined remote center position and the end-effector’s posture. Where the end-effector’s posture is calculated from values generated by the force/torque sensor due to direct teaching through a motion generation algorithm based on impedance control [Reference Kim, Zhang and Jin192]. The position control is designed by differential kinematics with PID control and back calculation.

Figure 15. Block diagram of RCM control and direct teaching with motion generation algorithm [Reference Kim, Zhang and Jin192].
Moreover, modeling and control of a laparoscopic parallel manipulator with three limbs having identical prismatic-universal-universal (3-PUU) architecture are discussed in a recent study [Reference Khattab193, Reference Zidane, Khattab, El-Habrouk and Rezeka194]. The manipulator has 3 DOFs corresponding to rotation about x-axis (Ex), y-axis (Ey), and translation along the z-axis (Pz). To avoid the computational complexity of solving the manipulator’s kinematics in real-time application, two feed-forward artificial neural networks (ANNs) are used as forward and inverse kinematics estimators [Reference Zidane, Khattab, El-Habrouk and Rezeka194, Reference Khattab, Zidane, El-Habrouk and Rezeka195]. The dynamic model of the 3-PUU manipulator is built using MATLAB Simscape environment, and several control schemes are investigated. Starting with motor control, PID and PID plus feed-forward using the model’s inverse dynamics control schemes were studied. Simulation results were compared to the PID and adaptive fuzzy logic control schemes implemented in a previous study by Khalifa et al. [Reference Khalifa, Fanni, Mohamed and Miyashita168]. Figure 16 depicts the comparison and shows that the PID plus feed-forward control scheme provides the best motor performance between the other control schemes [Reference Khattab193, Reference Zidane, Khattab, El-Habrouk and Rezeka194]. Compared to the adaptive fuzzy logic controller [Reference Khalifa, Fanni, Mohamed and Miyashita168], the PID plus feed-forward control provided better motor performance with 78.26% less maximum tracking error, in addition to being easier to implement online than the adaptive fuzzy logic controller. Closed-loop trajectory control based on Cartesian space feedback of the manipulators’ position and orientation and inverse kinematics ANN is then studied. Figure 17 shows the complete schematic control block diagram of the 3-PUU manipulator with closed-loop trajectory control. In this control system [Reference Khattab193, Reference Zidane, Khattab, El-Habrouk and Rezeka194], the position and orientation error is fed-back to the inverse kinematics ANN which acts as a controller. The ANN’s output represents the error in the prismatic displacements d1, d2, and d3 resulting from any change in the manipulator’s actual model. This error is then added to the desired prismatic displacement to compensate for the actual position and orientation error. The closed-loop control scheme can enhance the system’s performance, eliminating the error resulting from any change in the manipulator’s actual model due to manufacturing or assembly defects. Simulation results of a defected manipulator model show that the closed-loop control scheme improves the manipulator’s trajectory tracking capability compared to motor control, reducing the z-axis position error by 89.23% and the orientation error by 86.76% and 82.83% about x-axis and y-axis directions, respectively [Reference Khattab193, Reference Zidane, Khattab, El-Habrouk and Rezeka194].
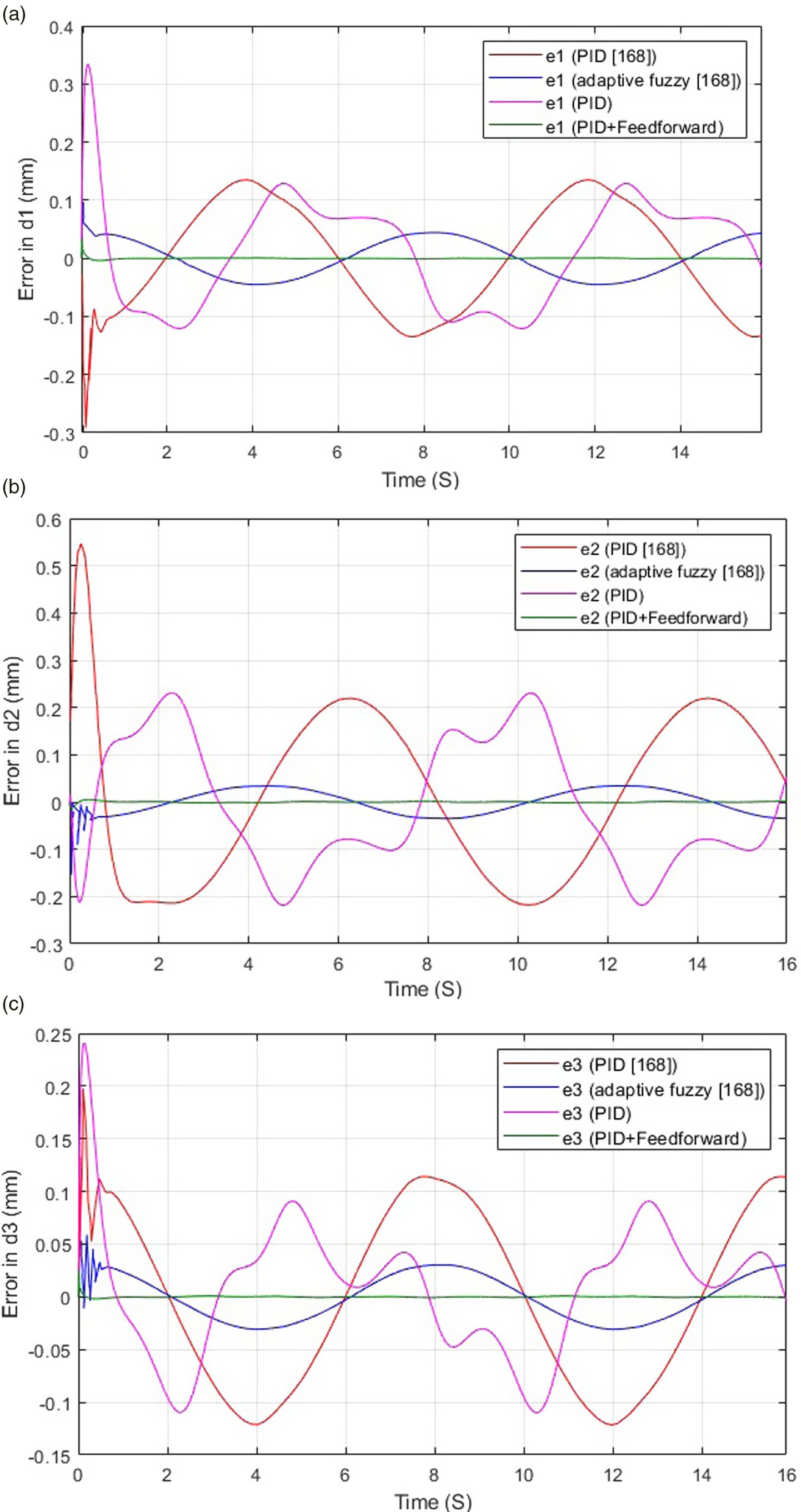
Figure 16. Comparison between the error in motors’ sine response using different control schemes for the 3-PUU laparoscopic manipulator [Reference Khattab193, Reference Zidane, Khattab, El-Habrouk and Rezeka194]. (A) Error in d1 (B) Error in d2 (C) Error in d3.

Figure 17. Schematic of the 3-PUU manipulator with closed-loop trajectory control [Reference Khattab193, Reference Zidane, Khattab, El-Habrouk and Rezeka194].
Figures 18 and 19 provide classifications of the discussed control systems according to their control objective and control input (Fig. 18) and type of controller used (Fig. 19).

Figure 18. Classification of control objectives and control inputs of the discussed control systems.

Figure 19. Classification of types of controllers used in the discussed control systems.
4. Clinical/Training applications of robotics in laparoscopic surgeries
Several experimental and clinical trials of laparoscope positioning robots have been performed, showing common results such as stable and accurate laparoscope movements, steady and less smudgy images, feasibility, safety, and no additional complications compared to conventional laparoscopy as shown in
Table VIII Detailed studies were conducted to gather this information. One study included 105 procedures of 21 different types that were performed by 43 consultant surgeons between 2013 and 2016 using Freehand robotic camera assistant. Overall surgeons’ satisfaction was 4.29 out of 5 for setup, 4.12 for ergonomics, 4.39 for usability, and 4.34 for the overall experience and detailed statistical data for each procedure is presented in ref. [Reference Ali, Lam and Coonar196]. In addition, several experiments were carried out to compare different laparoscope holders [Reference Sood, Arya, Maple, Grange and Haq15, Reference Gumbs, Crovari, Vidal, Henri and Gayet197–Reference Yavuz, Ystgaard, Skogvoll and Mårvik200]. Reported experimental/clinical trials of different MSTDCs (Table IX) show common results such as feasibility and safety, enhanced ergonomic posture of the surgeon, and enhanced surgical dexterity. In a recent study in Klaipeda University Hospital, 100 procedures were observed using Senhance robotic platform in general and colorectal surgery, gynecology, and urology. All surgeons felt confident with the system, reporting its feasibility and safety in different types of procedures [Reference Samalavicius, Janusonis, Siaulys, Jasėnas, Deduchovas, Venckus, Ezerskiene, Paskeviciute and Klimaviciute201]. From 2019 to 2020, 40 extraperitoneal radical prostatectomies were performed using Senhance, where it is reported that considerable surgical proficiency is gained after 30 cases and the learning curve is shorter for surgeons with previous laparoscopic experience. Detailed patient data and experimental results are provided in ref. [Reference Kastelan, Hudolin, Kulis, Penezic, Gidaro, Bakula, Zekulic and Knezevic202]. Moreover, randomized controlled trials that compared robot-assisted abdominopelvic surgery with laparoscopy, open surgery, or both were conducted. Data were gathered from 50 studies including 4894 patients, where 39 studies reported the incidence of complications requiring further surgical interventions, and 4 of which (10%) showed fewer complications with robot-assisted surgery [Reference Dhanani, Olavarria, Bernardi, Lyons, Holihan, Loor, Haynes and Liang203]. Although the majority of studies showed no difference in intraoperative complications, conversion rates, and long-term outcomes, surgeons reported that robotic-assisted surgeries provided an ergonomic advantage [Reference Bakalar204]. Furthermore, experimental trials of handheld devices were carried out in box trainers to evaluate their performance and effect on ergonomics [Reference Bensignor, Morel, Reversat, Fuks and Gayet21, Reference Sánchez-Margallo and Sánchez-Margallo27, Reference Sieber, Fellmann-Fischer and Mueller28]. Only RobotDex showed no improvement in technical performance, and Kymerax’s handle was described as non-ergonomic (Table I). Reported experimental evaluation of robotic training systems show better performance and enhanced accuracy compared to conventional training as shown in Table VI.
Table VIII. Summary of experimentation results of laparoscope positioning robots.

Table IX. Summary of experimentation results of MSTDCs.
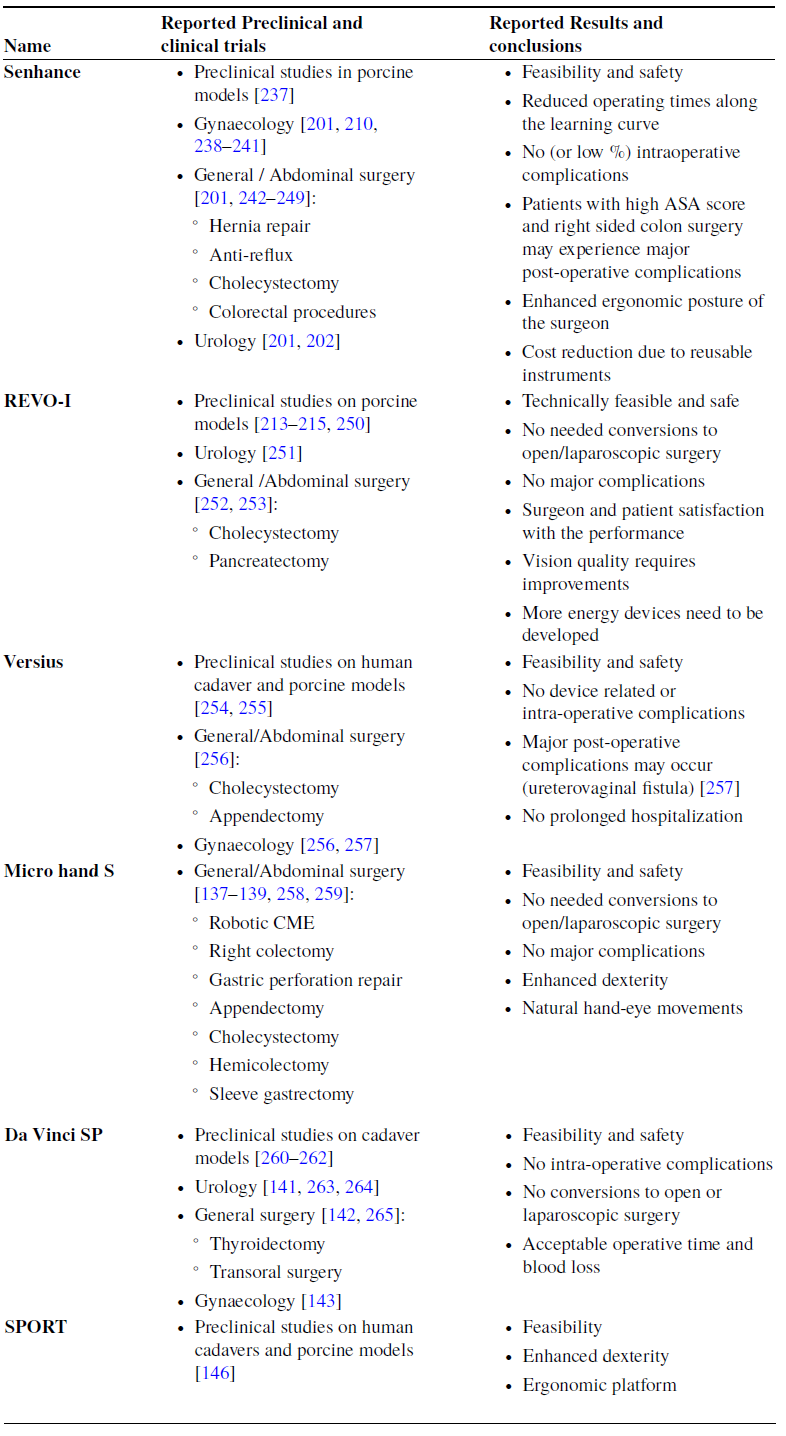
5. Discussion
Several robotic applications have been adopted to reduce the challenges opposed by laparoscopic surgeries. Laparoscope positioning robots are found to provide the surgeon with a steadier and less foggy view, eliminating the unintended moves resulting from the human assistant’s hand tremor. The voice control method offers a simple way of communication. However, voice recognition for each operator and vocal commands must be set before the operation. Head movements control is more intuitive, but the operator might find it more difficult to focus on his/her head movements while pressing the foot pedal. Both voice-controlled Viky, and infrared headset controlled Freehand endoscope positioners are considered leading robots in this category in terms of accuracy, applicability, and weight. They are also still commercially available and FDA approved. Attempts have been made to develop more intuitive solutions, where cognitive laparoscope positioners with preliminary knowledge are emerging to provide real autonomous behavior.
MSTDCs have already been used in millions of laparoscopic surgeries, mostly under the name da Vinci. However, other systems might end this monopoly because of their lower cost and desirable features like haptic feedback and intuitive endoscope control. Nevertheless, handheld robotic devices that integrate both the master and the slave in one handheld device provide a much cheaper and smaller solution. They combine both benefits of conventional and tele-operated laparoscopic surgeries, enhancing surgical dexterity using ergonomic handles with wrist joints at the instrument’s tip and increasing the number of DOFs, without losing the advantages of reduced setup time and haptic feedback of conventional laparoscopy.
Finally, robotic training systems provide an intelligent alternative to the hand-over-hand conventional training and assessment of surgical trainees.
The number of references gathered to conduct this literature review and their distribution over a timeline from 1995 to 2022 is presented in Figure 20. While Fig. 21 shows reference distribution based on the type of application.

Figure 20. Distribution of references included in this literature review over a timeline from 1995 to 2022.

Figure 21. Distribution of references included in this literature review based on the type of application.
6. Conclusion
This paper has reviewed different robotic technologies implemented to overcome the challenges of laparoscopic surgeries. Existing papers classified robotic systems according to their application only, or focused on a single category as laparoscope positioners, tele-operated master–slave systems, or handheld devices. All of the proposed systems have proved to be beneficial in laparoscopic surgeries, and some of them offer real autonomy. The major benefit this paper has over existing papers is that it classifies the proposed systems into main categories according to their application and provides further classifications of the systems based on their configuration, DOFs, and control scheme, in addition to presenting a generalized block diagram. This approach can provide researchers/students with a starting point for the state of art in their research fields. Where the different classifications’ criteria along with the generalized control block diagram provide summary and comparisons of the existing laparoscopic robots, paving the way for future research and selecting the best robotic technique to be used in a certain laparoscopic surgery.
Future directions of surgical robots in laparoscopic surgeries will include a higher level of automation. It is anticipated that robots with the ability to make real-time surgical decisions and execute desired tasks - under the surgeon’s supervision- will be used in the operating rooms in the future [Reference Kawashima, Kanno and Tadano10, Reference Kitaguchi, Takeshita, Hasegawa and Ito205], with the aid of DL and providing robots with preliminary knowledge of the surgical procedures. Repetitive, time consuming, and tedious tasks, like instruments maneuvering, and suturing tasks will be autonomously performed by robots. According to Asensus Surgical CEO [Reference Littlejohns206], the next phase is cognitive robots, “building a digital twin for the surgeon” using ML and recorded data from successful surgeries that will be deployed more to systematic surgical tasks than those that rely heavily on experience and skill. Moreover, future work will also include hardware modifications. Snake-like continuum robots represent an active field of research and development that might replace conventional rigid-link robots.
Financial support
This research received no specific grant from any funding agency, commercial, or not-for-profit sectors.
Competing interests
The authors declare none.
Ethical considerations
No ethical issue with this paper.
Authors’ contributions
Iham F. Zidane and Yasmin Khattab conducted the review and wrote the first draft; Iham F. Zidane, Yasmin Khattab, Sohair Rezeka, and Mohamed El-Habrouk revised the manuscript; and Iham F. Zidane, Sohair Rezeka, and Mohamed El-Habrouk provided advice and supervision.