Introduction
Rates of comorbid posttraumatic stress disorder and alcohol use disorder (PTSD/AUD) are extremely high (Blanco et al., Reference Blanco, Xu, Brady, Perez-Fuentes, Okuda and Wang2013). PTSD/AUD comorbidity is associated with greater symptom severity and functional impairment than either disorder alone (Norman, Haller, Hamblen, Southwick, & Pietrzak, Reference Norman, Haller, Hamblen, Southwick and Pietrzak2018). Although several studies show that gold-standard trauma-focused psychotherapies are efficacious interventions for some patients with comorbid PTSD/AUD (Norman et al., Reference Norman, Trim, Haller, Davis, Myers, Colvonen and Mayes2019; Roberts, Roberts, Jones, & Bisson, Reference Roberts, Roberts, Jones and Bisson2015; Ruglass et al., Reference Ruglass, Lopez-Castro, Papini, Killeen, Back and Hien2017), they are associated with high-dropout rates and a significant number of individuals maintaining elevated symptoms after treatment (Krystal et al., Reference Krystal, Davis, Neylan, Raskind, Schnurr, Stein and Huang2017; Steenkamp, Reference Steenkamp2016). One potential reason for modest outcomes is that PTSD and AUD are highly heterogeneous – suggesting that they are not distinct disorders but are comprised of sets of neurobiological mechanisms that have both unique and shared pathophysiology. Under this framework, there is a need to construct neurobiologically-grounded subtypes focused on dysfunctional brain networks that capture the heterogeneity (Feczko et al., Reference Feczko, Miranda-Dominguez, Marr, Graham, Nigg and Fair2019; Huys, Maia, & Frank, Reference Huys, Maia and Frank2016; Yamada et al., Reference Yamada, Hashimoto, Yahata, Ichikawa, Yoshihara, Okamoto and Kawato2017). Subtyping patients on shared brain network features instead of clinical symptoms could inform the mechanistic understanding of PTSD/AUD pathophysiology and provide clinically-useful biomarkers that are associated with treatment outcomes (Milham, Craddock, & Klein, Reference Milham, Craddock and Klein2017; Woo, Chang, Lindquist, & Wager, Reference Woo, Chang, Lindquist and Wager2017).
Mapping brain network communication has been instrumental in characterizing the pathophysiology of PTSD and AUD in addition to understanding how network connectivity patterns relate to treatment success (Sheynin et al., Reference Sheynin, Duval, King, Angstadt, Phan, Simon and Liberzon2020; Zhutovsky et al., Reference Zhutovsky, Thomas, Olff, van Rooij, Kennis, van Wingen and Geuze2019). A common method to measure brain network communication is through the use of functional connectivity (Abi-Dargham & Horga, Reference Abi-Dargham and Horga2016). Such work has revealed that PTSD and AUD are commonly observed to involve dysfunctional neural connectivity within and between several regions implicated in affective reactivity, cognition, and emotion regulation (Akiki, Averill, & Abdallah, Reference Akiki, Averill and Abdallah2017; Koch et al., Reference Koch, van Zuiden, Nawijn, Frijling, Veltman and Olff2016; Sheynin & Liberzon, Reference Sheynin and Liberzon2017). For example, increased pregenual anterior cingulate cortex (pgACC) connectivity with the amygdala is associated with higher PTSD symptoms after completing a trauma-focused psychotherapy (Cisler et al., Reference Cisler, Sigel, Kramer, Smitherman, Vanderzee, Pemberton and Kilts2015) and increased connectivity of the insula with the posterior midcingulate cortex (pMCC) is observed in PTSD patients who no longer met the criteria for PTSD post-treatment (Simmons, Norman, Spadoni, & Strigo, Reference Simmons, Norman, Spadoni and Strigo2013). In AUD, decreased connectivity between regions of the prefrontal cortex (PFC) and the subcortical network consisting of reward regions (e.g. ventral striatum) is associated with relapse (Camchong, Stenger, & Fein, Reference Camchong, Stenger and Fein2013) and increased connectivity between the PFC, striatum, and the insula is associated with residential treatment program completion (Kohno, Dennis, McCready, & Hoffman, Reference Kohno, Dennis, McCready and Hoffman2017).
A limitation of these investigations is that they do not consider the clinical and neural heterogeneity within these disorders. To date, the majority of neuroimaging studies exclude individuals with commonly comorbid conditions, limiting the generalizability and translation of their findings (Blanco et al., Reference Blanco, Wall, Lindquist, Rodríguez-Fernández, Franco, Wang and Olfson2016). Instead, data-driven approaches that characterize highly representative patients on functional connectivity patterns show promise in identifying neurobiological subtypes within broader heterogeneous diagnostic groups and in predicting treatment response (Drysdale et al., Reference Drysdale, Grosenick, Downar, Dunlop, Mansouri, Meng and Liston2017; Etkin et al., Reference Etkin, Maron-Katz, Wu, Fonzo, Huemer, Vértes and O'Hara2019; Maron-Katz et al., Reference Maron-Katz, Zhang, Narayan, Wu, Toll, Naparstek and Etkin2019).
Methods to improve the identification of the complex and directional neural patterns underlying psychiatric heterogeneity are needed (Reid et al., Reference Reid, Headley, Mill, Sanchez-Romero, Uddin, Marinazzo and Cole2019). Effective connectivity-derived biotypes have been shown to be superior in differentiating PTSD patients from healthy controls and better predict symptom severity than static connectivity measures (Jin et al., Reference Jin, Jia, Lanka, Rangaprakash, Li, Liu and Deshpande2017; Li et al., Reference Li, Zhu, Jiang, Jin, Zhang, Guo and Liu2014). One novel data-driven effective connectivity strategy called group iterative multiple model estimation with subgrouping (S-GIMME) has been shown to be particularly successful in deriving reliable and parsimonious directed connectivity and in separating clinically heterogeneous populations into distinct subtypes, even in samples as low as 25 (Beltz & Gates, Reference Beltz and Gates2017; Gates, Lane, Varangis, Giovanello, & Guskiewicz, Reference Gates, Lane, Varangis, Giovanello and Guskiewicz2017). A recent review of network modeling approaches shows that GIMME is receiving a growing interest as an analytical tool to understand the causal relationships between brain networks (Robinaugh, Hoekstra, Toner, & Borsboom, Reference Robinaugh, Hoekstra, Toner and Borsboom2020). S-GIMME applied to rs-fMRI has been fruitful in identifying subtypes in cognitive task ability (McCormick & Telzer, Reference McCormick and Telzer2018), accurately distinguishing depressed patients from healthy individuals (Price et al., Reference Price, Lane, Gates, Kraynak, Horner, Thase and Siegle2017b), and revealing major depressive disorder, attention-deficit/hyperactivity disorder, and transdiagnostic internalizing psychiatric disorder and personality subtypes (Chahal et al., Reference Chahal, Weissman, Hallquist, Robins, Hastings and Guyer2020; Lazarus, Sened, & Rafaeli, Reference Lazarus, Sened and Rafaeli2020; Price, Gates, Kraynak, Thase, & Siegle, Reference Price, Gates, Kraynak, Thase and Siegle2017a; Price et al., Reference Price, Beltz, Woody, Cummings, Gilchrist and Siegle2020). However, no studies to date have leveraged this data-driven approach to identify neural subtypes of comorbid PTSD/AUD individuals or to treatment response (Gilpin & Weiner, Reference Gilpin and Weiner2017).
To this end, we applied S-GIMME to rs-fMRI in a sample of 53 psychotherapy treatment-seeking Veterans diagnosed with comorbid PTSD and AUD participating in a randomized clinical trial examining treatment effectiveness of trauma-focused and non-trauma-focused therapies (Norman et al., Reference Norman, Trim, Haller, Davis, Myers, Colvonen and Mayes2019). Connectivity-based subtyping was conducted using seven regions of interest (ROIs) that were selected from a recent meta-analysis showing brain regions, including the cingulate cortex, insula, and the PFC, that distinguished PTSD and AUD patients from healthy controls (Klaming et al., Reference Klaming, Harlé, Infante, Bomyea, Kim and Spadoni2019). The aim of our investigation was to extend these findings by examining whether pre-treatment effective connectivity of these PTSD and AUD-relevant brain regions can be used to identify subgroups of patients and to test whether effective connectivity subgroups predict psychotherapeutic treatment improvement in Veterans.
Materials and methods
Participants
A total of 59 subjects (mean age = 40.23, s.d. = 10.73; range = 25–63; 47 men) from a parent psychotherapy treatment trial (NCT01601067) (Norman et al., Reference Norman, Trim, Haller, Davis, Myers, Colvonen and Mayes2019) comparing trauma-focused [integrated-prolonged exposure (I-PE)] to non-trauma-focused [integrated-coping skills (I-CS)] psychotherapy for comorbid PTSD/AUD agreed to complete neuroimaging prior to being assigned to a treatment arm (see Table 1 for sample characteristics). I-PE and I-CS therapy arms were delivered in 90 min individual sessions (12–16 sessions for both therapy arms; Norman et al., Reference Norman, Trim, Haller, Davis, Myers, Colvonen and Mayes2019). Subjects completed an average of 10.32 (s.d. = 4.33) therapy sessions [I-PEmean = 9.10, I-PESD = 4.35; I-CSmean = 11.79, I-CSSD = 3.89; t(50.67) = 2.37, p = 0.02]. Five subjects did not complete scanning due to technical and scheduling issues and one subject was removed due to do excessive motion artifact (>0.3 mm mean FD) measured via frame-wise displacement, leaving a total of 53 pretreatment resting-state scans. Thirty-eight subjects were retained at post-treatment. Subjects provided informed consent and were monetarily compensated for their participation. Study procedures were approved by the institutional review board of the Veteran's Administration San Diego Healthcare System.
Table 1. Descriptive statistics and sample characteristics
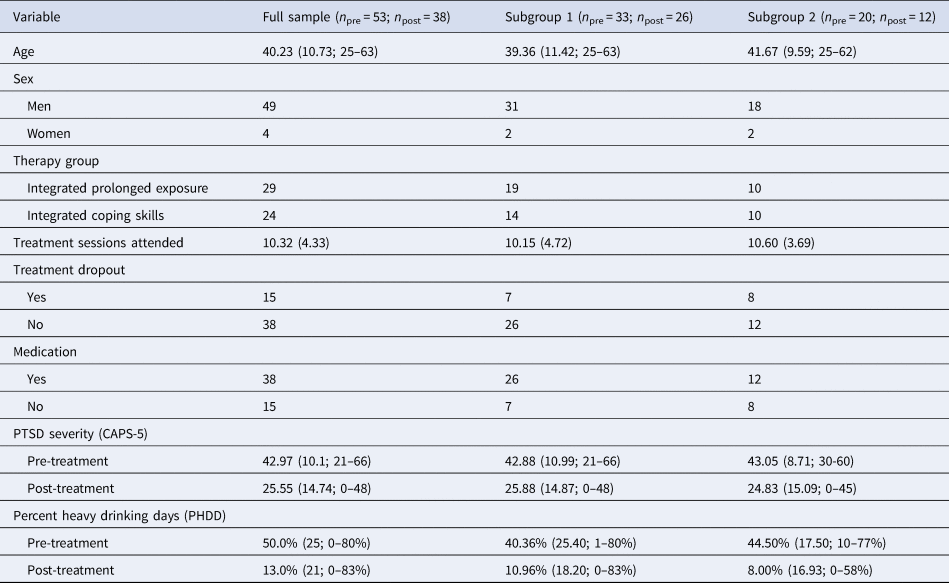
Note. Data reported are the raw descriptive statistics. No connectivity subgroup differences were found on descriptive measures. n pre, number of subjects at pre-treatment; n post, number of subjects at post-treatment; CAPS-5, Clinician Administered PTSD Scale for DSM-5.
Clinical measures
Clinician Administered PTSD Scale for DSM-5 (CAPS-5). The CAPS-5 (score range 0–80, with 0 indicating no PTSD symptoms and 80 indicating extreme ratings across all symptoms), a 30-item structured interview considered to be the criterion standard for PTSD, was the primary measure of PTSD symptoms and diagnosis. The CAPS-5 diagnosis has been shown to demonstrate strong interrater reliability (κ = 0.78), and severity scores had strong internal consistency (α = 0.88) (Weathers et al., Reference Weathers, Bovin, Lee, Sloan, Schnurr, Kaloupek and Marx2018).
Percentage of heavy drinking days (PHDD). Frequency and quantity of alcohol use were assessed using the Timeline Follow-Back, a calendar-assisted structured clinical interview that displays good psychometric properties (Sobell & Sobell, Reference Sobell, Sobell, Litten and Allen1992). The PHDD was calculated by dividing the number of days in which five or more drinks for men or five or more drinks for women were consumed by the total number of days in the reference period (i.e. 90 days at baseline and time from baseline assessment for post-treatment assessment).
fMRI acquisition and preprocessing
MRI acquisition and preprocessing are detailed in the Supplementary Materials.
Regions of interest
Five to 15 ROIs are recommended when using S-GIMME for identifying directed connectivity paths and sample subtyping (https://gimme.web.unc.edu/63-2/gimme-basics/). Therefore, we chose to take a hypothesis-driven approach for ROI selection based upon a recent meta-analysis from our group that identified seven key brain regions important for distinguishing between PTSD, AUD, their overlap, and healthy control populations (Klaming et al., Reference Klaming, Harlé, Infante, Bomyea, Kim and Spadoni2019). Our aim was to extend this work by determining whether effective connectivity of these regions can also identify subgroups of comorbid PTSD/AUD patients and whether these subgroups are clinically relevant. To this end, we extracted mean BOLD time-series from seven spherical ROIs with a 10 mm radius. ROIs were placed in three regions of the rostral cingulate cortex (see Shackman et al., Reference Shackman, Salomons, Slagter, Fox, Winter and Davidson2011 for detailed anatomical and functional description of the cingulate cortex): one in the pMCC (x = 0, y = −4, z = 34), one in the pgACC (x = 2, y = 42, z = 20), and one in the subgenual cingulate cortex (sgACC; x = 0, y = 6, z = −6). One ROI was placed in the left middle frontal gyrus (MFG; x = −46, y = 10, z = 38). One was placed in the inferior parietal cortex (IPC) near the supramarginal gyrus (x = 52, y = −46, z = 44). One in the left insula (x = −38, y = −4, z = 6) and one in the right putamen (x = 34, y = −4, z = 0). The extracted time-series from these seven ROIs were then entered into the connectivity clustering analysis described below.
Data-driven subgroup clustering via effective connectivity
S-GIMME in R (package: gimme) was used to identify whether clinically relevant subgroups of subjects can be derived based upon pretreatment resting-state effective connectivity patterns (Gates et al., Reference Gates, Lane, Varangis, Giovanello and Guskiewicz2017) (online Supplementary Fig. S1). S-GIMME is a unified structural equation modeling strategy that reliably recovers both the presence and direction of connectivity paths within heterogeneous individuals (Beltz & Gates, Reference Beltz and Gates2017; Gates, Molenaar, Iyer, Nigg, & Fair, Reference Gates, Molenaar, Iyer, Nigg and Fair2014). S-GIMME can be used to identify common pathways across all subjects but importantly can classify subjects within subgroups based upon unique connectivity profiles and estimate subject-level connectivity (Gates et al., Reference Gates, Lane, Varangis, Giovanello and Guskiewicz2017).
First, S-GIMME enters all the ROIs in a single step to mitigate the problem of variable order during the model-building procedure. Next, compared to a null model that assumes no connections exist, S-GIMME searches across individuals and adds lagged (lag = 1 TR) and contemporaneous directed paths that are common for the majority of subjects (group-level). S-GIMME uses modification indices to count individuals whose connectivity paths exist across individuals and only includes the path if it improves model fit for the majority of the subjects (75%) and must survive a conservative Bonferroni correction of 0.05/number of subjects (N = 53 in the current analysis). Paths not meeting these criteria are pruned. This process is iteratively repeated for each connectivity path and stops adding paths when no new path would improve the model-fit indices in the majority of the sample. If any remaining connection no longer improves model fit during this iterative process, then that path will be removed from the model. This pruning step can occur at the individual, sub-group, or group-level model estimation process (Beltz & Gates, Reference Beltz and Gates2017). This approach is robust to outliers and sign differences of parameter estimates (Gates et al., Reference Gates, Lane, Varangis, Giovanello and Guskiewicz2017). Next, S-GIMME uses Walktrap unsupervised community detection (Gates, Henry, Steinley, & Fair, Reference Gates, Henry, Steinley and Fair2016; Orman, Labatut, & Cherifi, Reference Orman, Labatut and Cherifi2012) on the similarity matrix derived from the individual-level estimates of the group-level connectivity paths to identify an optimal number of subgroups who have similar connectivity profiles. Once subgroups are identified, S-GIMME searches for unique connectivity paths for each data-driven subgroup. Finally, S-GIMME identifies individual-level connections using the group- and subgroup-derived temporal patterns as priors (not reported here).
S-GIMME stops adding new paths/connections once the model achieves good fit on at least two out of the following four model fit indices (Brown, Reference Brown2006): confirmatory fit index (CFI) ⩾0.95; non-normed fit index ⩾0.95; standardized root mean square residual (SRMR) ⩽0.05; root mean square error of approximation ⩽0.05 (Gates & Molenaar, Reference Gates and Molenaar2012). Stopping the S-GIMME path search based on model fit instead of stopping based on individual path significance ensures that extraneous connectivity paths are not added, a critical step to minimize complex models and model overfitting. A simulation study showed that these model fits can be achieved with low sample sizes (e.g. N = 25) (Gates et al., Reference Gates, Lane, Varangis, Giovanello and Guskiewicz2017). After identification of subgroups derived from GIMME, further characterization can be completed by comparing subgroups on clinical and treatment measures. Because the contemporaneous and lagged pathways were identical and highly correlated in our analysis (R 2s > 0.79), we only visualize the contemporaneous pathways for simplicity in the main report (Price, et al., Reference Price, Gates, Kraynak, Thase and Siegle2017a, Reference Price, Lane, Gates, Kraynak, Horner, Thase and Siegle2017b). Full connectivity maps are presented in online Supplementary Fig. S1b and S1d.
Subgroup characterization analysis
To examine connectivity subgroup differences in treatment outcomes, a linear mixed-effects model (lmer in the lme4 R package) was conducted on CAPS-5 scores. Connectivity subgroup, treatment group (I-PE and I-CS), and time (pre-treatment and post-treatment) were entered as fixed effects and the intercept was specified as random to account for repeated measurements per subject. We used the Satterthwaite's method to calculate the Type III sum of squares. An identical model was used to examine subgroup effects on PHDD. Post-hoc independent-samples t tests were corrected for multiple comparisons using the Benjamini and Hochberg (Reference Benjamini and Hochberg1995) false discovery rate (FDR).
Exploratory subgroup analysis
We conducted a series of exploratory analyses to further phenotype the connectivity-derived subgroups. We examined brain volume differences and additional clinical and behavioral measures. The aim of these analyses is exploratory and for generating future research questions (see Supplementary Materials).
Results
Subgroup connectivity profiles
Final model fit indices indicated excellent fit across individuals, CFI = 0.96 and SRMR = 0.03. S-GIMME identified two subgroups with common and unique network effective connectivity patterns. First, across all subjects, S-GIMME identified a series of seven contemporaneous directed connectivity pathways (Fig. 1; Table 2). These connectivity paths consisted of directed paths from the left insula to the right putamen, MFG, and the IPC, directed paths from the putamen to the pgACC and the sgACC, a path from the MFG to the pgACC, and one path from the pgACC to the pMCC. Although these paths are common across subgroups, subgroup differences in strength in these networks emerged in two out of the seven networks. Specifically, subgroup 2 had significantly lower insula to IPC connectivity (t = 3.32, p = 0.003, p FDR = 0.011) and greater pgACC to pMCC connectivity compared to subgroup 1 (t = −4.18, p = 0.0002, p FDR = 0.001; Fig. 2). Next, examination of subgroup connectivity revealed that subgroup 2 was further characterized by a pgACC to IPC path, while subgroup 1 had IPC to MFG and putamen to pMCC connectivity paths (Fig. 1b).

Fig. 1. S-GIMME identified common and unique effective connectivity. (a) S-GIMME group-level effective connectivity paths. Paths represent connections common to most subjects (red arrows). Green border on arrow represents path significantly greater for subgroup 1. Yellow border on arrow represents path significantly greater for subgroup 2. (b) Subgroup 1 was further characterized by two effective connectivity paths: putamen to pMCC, and IPC to MFG (green arrows). (c) Subgroup 2 was characterized by one additional effective connectivity path: pgACC to IPC (yellow arrows). Auto-regressive and lagged paths are not shown.
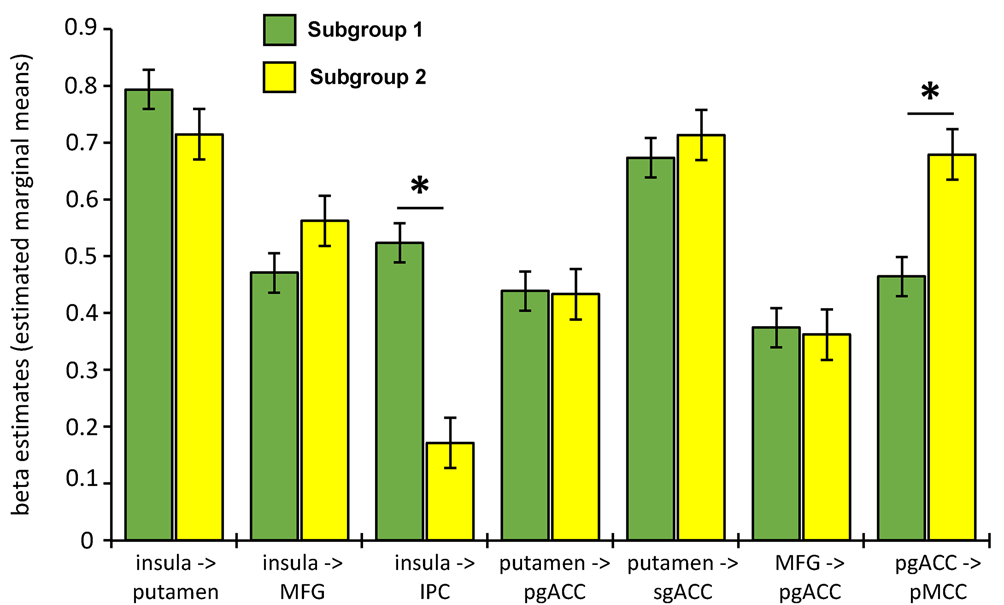
Fig. 2. Connectivity subgroup differences in group-level paths. Subgroup 1 had a greater insula to IPC path compared to subgroup 2. Subgroup 2 had a greater pgACC to pMCC path compared to subgroup 1. *ps < 0.004 (uncorrected) and psFDR < 0.02. See online version for color.
Table 2. Path estimates and Z-scores
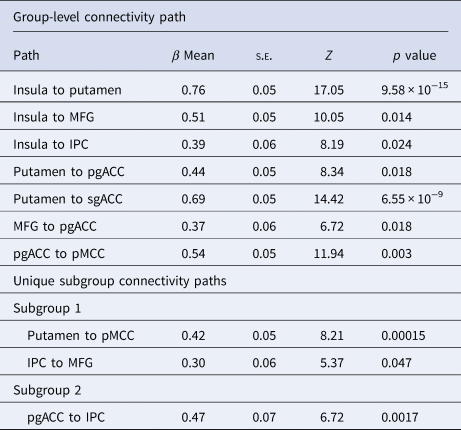
Note. β Means, s.e.s, Z, and p values are computed as an average across subjects.
Clinical characterization of subgroups
We found a significant subgroup × therapy group × time interaction controlling for medication status [F (1,41.2) = 4.58, p = 0.038]. Within the I-CS therapy group, subgroup did not significantly predict PTSD symptom outcomes (subgroup main effect, p = 0.82, subgroup × time interaction, p = 0.63). In contrast, for patients undergoing I-PE, subgroup was associated with PTSD symptoms (subgroup × time interaction, p = 0.041). Post-hoc t tests revealed that before beginning I-PE, there were no subgroup differences (t = −0.17, p = 0.87). As shown in Fig. 3a, patients in subgroup 2 showed a greater reduction in PTSD symptoms post-treatment (t = 2.63, p = 0.022, p FDR = 0.044). Subgroups particularly moderated treatment outcomes on avoidance and hyperarousal symptoms (three-way interaction ps < 0.017; Supplementary Materials).
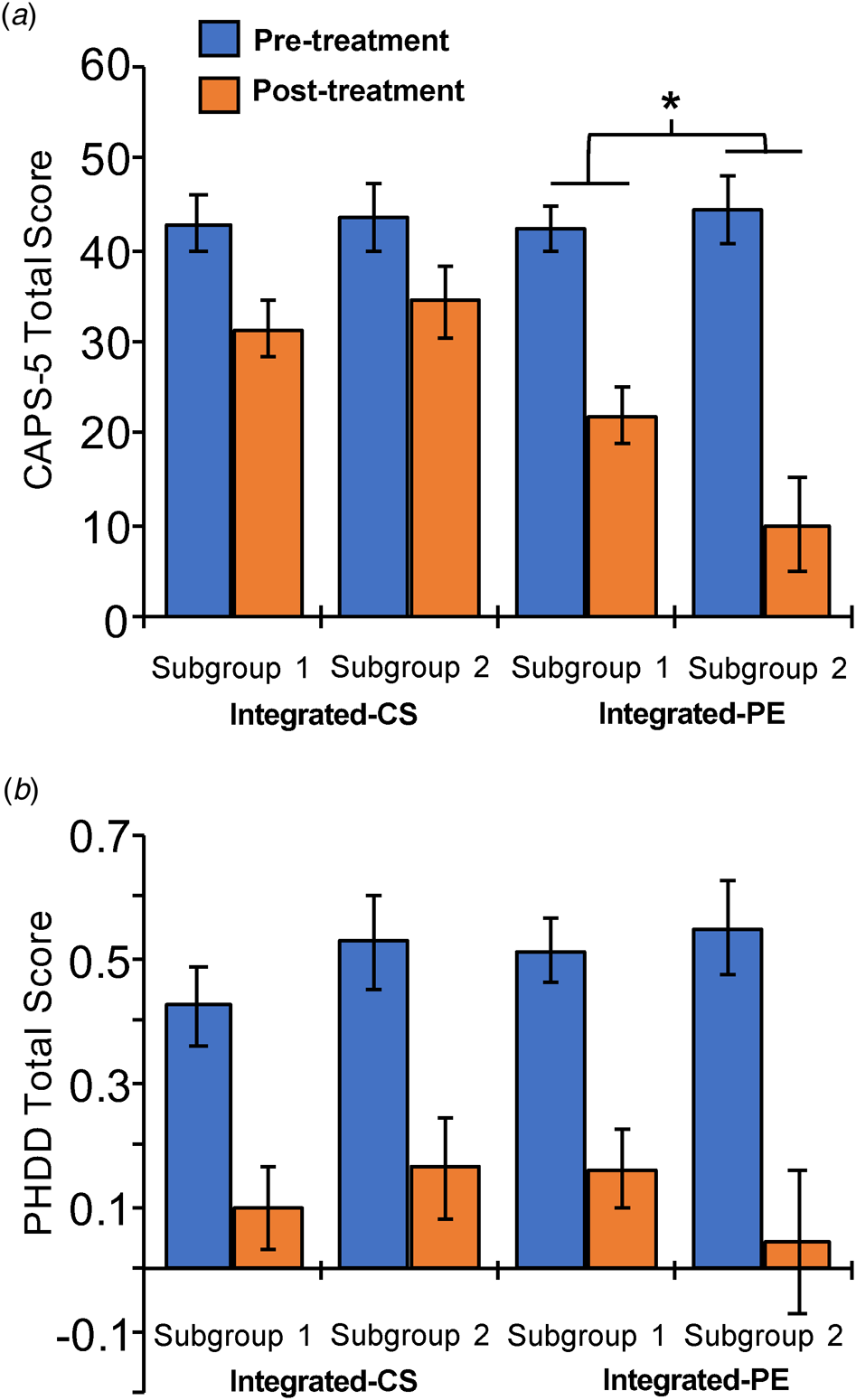
Fig. 3. Connectivity cluster moderated psychotherapy outcome for PTSD symptoms. (a) Clinician Administered PTSD Scale for DSM-5 (CAPS-5) total severity scores (estimated marginal means) for pre- and post-treatment assessments. Connectivity subgroup 2 showed a bigger change in PTSD symptoms than subgroup 1 in the I-PE groups: subgroup × time interaction, *p = 0.041. (b) Percentage of heavy drinking days (PHDD) for pre- and post-treatment assessments (estimated marginal means). Connectivity subgroup did not impact improvement in drinking symptoms assessed using the PHDD. See online version for color.
In light of the therapy group difference in the number of sessions attended (therapy group: t = 2.37, p = 0.02), and the qualitative difference in the number of sessions attended between connectivity subgroup (subgroup: t = −0.39, p = 0.70) and the qualitative difference in therapy drop-out status between connectivity subgroups and therapy groups (subgroup: χ2 = 1.34, p = 0.25; therapy group: χ2 = 1.97, p = 0.16), we added number of attended sessions and drop-out status as covariates into the model. The subgroup × therapy group × time interaction remained significant (p = 0.047).
To determine whether any of the seven paths that showed subgroup differences influenced treatment outcomes, we computed a multiple linear regression. We entered the seven directed connectivity path β values as the predictors of CAPS total score post-treatment with CAPS pretreatment, therapy group, connectivity cluster subgroup, and medication status as covariates. Results indicate that only the putamen to sgACC path significantly predicted CAPS post-treatment scores after controlling for CAPS at pre-treatment [β = 33.81, t = 2.43, p = 0.022 (uncorrected)].
Subgroup was unrelated to drinking behavior as measured with the PHDD (Fs < 1.09, ps > 0.30; Fig. 3b). See Supplementary Materials for the results associated with Therapy Group Type on the clinical symptoms (see also Norman et al., Reference Norman, Trim, Haller, Davis, Myers, Colvonen and Mayes2019). Connectivity subgroups identified did not significantly differ on age, sex, ethnicity, race, medication status, treatment group assignment (I-CS v. I-PE), number of treatment sessions attended, or treatment drop-out (ps > 0.25). None of the seven connectivity paths predicted drinking behavior (ps > 0.27). There were no significant differences between subgroups on MRI movement-related variables: TRs censor, DVARS, or FD (Fs < 1.21, ps > 0.23).
Exploratory results
We did find subgroup differences in volumes of a handful of regions, including the PFC and medial PFC (ps < 0.07; uncorrected). There were no differences between subgroups on diagnostic comorbidities (ps > 0.46; online Supplementary Tables S1 and S2).
Discussion
The aim of the current investigation was to identify neural connectivity subtypes of comorbid PTSD/AUD patients and to test whether the data-driven subtypes are associated with psychotherapy outcomes. We observed that the PTSD/AUD connectivity subtype 2 was associated with PTSD symptom improvement from I-PE. Furthermore, this connectivity subgroup was characterized by lower insula to IPC, higher pgACC to pMCC connectivity, and was further characterized by a pgACC to IPC connectivity path compared to subgroup 1. Both subgroups showed similar improvement from I-CS psychotherapy. Finally, we did not observe that connectivity subgroup moderated treatment outcomes for alcohol use. Our results provide novel evidence that data-driven approaches to characterize PTSD/AUD based on underlying neural connectivity patterns can detect clinically relevant subtypes.
There were five connectivity patterns that differentiated subgroup 2 from subgroup 1. Two primary regions played a particularly important role in subgroup differences. These regions included an ROI in the (1) IPC, and (2) two ROIs in the cingulate cortex: the pgACC and the pMCC. Our findings are consistent with previous work showing that PTSD and AUD are associated with aberrant activation and connectivity for each of these regions (Akiki et al., Reference Akiki, Averill and Abdallah2017; Gilpin & Weiner, Reference Gilpin and Weiner2017) and activity in these regions are associated with clinical outcomes (Malejko, Abler, Plener, & Straub, Reference Malejko, Abler, Plener and Straub2017; van Rooij, Kennis, Vink, & Geuze, Reference van Rooij, Kennis, Vink and Geuze2016; Zakiniaeiz, Scheinost, Seo, Sinha, & Constable, Reference Zakiniaeiz, Scheinost, Seo, Sinha and Constable2016). The subgroup connectivity profile differences among these regions may partially explain why subgroup 2 benefited more from I-PE than I-CS.
First, compared to subgroup 1, subgroup 2 had lower insula to IPC connectivity. This is consistent with data showing that compared to healthy controls, PTSD is associated with decreased IPC and insula connectivity (Zhang et al., Reference Zhang, Xie, Chen, Li, Guo and Chen2016). The IPC is highly connected anatomically and functionally across the brain (Wang et al., Reference Wang, Zhang, Rong, Wei, Zheng, Fox and Jiang2016; Zhang & Li, Reference Zhang and Li2014) and is a critical region implicated in attention, cognitive control, and emotion regulation (Etkin, Buchel, & Gross, Reference Etkin, Buchel and Gross2015). The insula is an integral hub in the salience network (Menon and Uddin, Reference Menon,V.2010), plays a prominent role in integrating cognitive and affectively salient information (Craig, Reference Craig2009; Gu et al., Reference Gu, Liu, Van Dam, Hof and Fan2013), and is important for interoceptive awareness (Critchley et al., Reference Critchley, Wiens, Rotshtein, Ohman and Dolan2004). Here, we show that the subgroup that benefited the most from I-PE had lower insula to IPC connectivity compared to the other subgroup, suggesting that this group may be better able to disengage attention and cognitive control from affective and interoceptive information that can interfere with goal-related behavior (Kuckertz et al., Reference Kuckertz, Amir, Boffa, Warren, Rindt, Norman and McLay2014).
Second, we found that subgroup 1 was further characterized by an IPC to MFG connectivity path. Experimental evidence indicates that higher levels of anxiety are associated with increased parietal and prefrontal activation that biases attention and working memory to potential threat (Balderston et al., Reference Balderston, Hale, Hsiung, Torrisi, Holroyd, Carver and Grillon2017; Stout, Shackman, Pedersen, Miskovich, & Larson, Reference Stout, Shackman, Pedersen, Miskovich and Larson2017). Our observation that subgroup 1 had unique IPC to MFG connectivity and did not improve as much from I-PE as subgroup 2 may suggest that subgroup 1 may be characterized by maladaptive utilization of working memory – a cognitive process important for facilitating extinction learning (Stout et al., Reference Stout, Acheson, Moore, Gur, Baker, Geyer and Risbrough2018). These results suggest that patients similar to subgroup 1 that have overactive pretreatment frontoparietal connectivity may see increased benefit from prolonged exposure therapy when an adjunctive treatment that targets the frontoparietal network is added (i.e. working memory training or repetitive transcranial magnetic stimulation) (Bomyea, Stein, & Lang, Reference Bomyea, Stein and Lang2015; Osuch et al., Reference Osuch, Benson, Luckenbaugh, Geraci, Post and McCann2009).
Third, subgroup 2 was characterized by a pgACC to IPC path. The pgACC is one of two primary regions in the anterior portion of the rostral cingulate cortex (Shackman et al., Reference Shackman, Salomons, Slagter, Fox, Winter and Davidson2011; Vogt, Nimchinsky, Vogt, & Hof, Reference Vogt, Nimchinsky, Vogt and Hof1995). The pgACC is well-positioned anatomically and functionally to exert influence on the flexible deployment of cognitive and affective control (Rolls, Reference Rolls2019; Shackman et al., Reference Shackman, Salomons, Slagter, Fox, Winter and Davidson2011; Tang et al., Reference Tang, Jbabdi, Zhu, Cottaar, Grisot, Lehman and Haber2019), is a critical node in the default mode network and internal cognitive processing (Raichle, Reference Raichle2015; Smallwood, Brown, Baird, & Schooler, Reference Smallwood, Brown, Baird and Schooler2012), and may be an important hub for dysfunction across a broad-spectrum of psychiatric disorders (Goodkind et al., Reference Goodkind, Eickhoff, Oathes, Jiang, Chang, Jones-Hagata and Etkin2015). Importantly, individuals with PTSD show dysfunctional pgACC connectivity (Kennis, Rademaker, van Rooij, Kahn, & Geuze, Reference Kennis, Rademaker, van Rooij, Kahn and Geuze2015) and decreased activation during affective interference tasks (Clausen et al., Reference Clausen, Francisco, Thelen, Bruce, Martin, McDowd and Aupperle2017). Clinically, increased IPC and pgACC connectivity is associated with improvements from cognitive behavioral therapy in depressed patients (Sambataro et al., Reference Sambataro, Doerig, Hänggi, Wolf, Brakowski, Holtforth and Spinelli2018). Furthermore, the pgACC resting-state connectivity results observed in the current report extend the structural neuroimaging findings showing the clinical importance of this region in prolonged exposure therapy for PTSD. For example, pretreatment pgACC cortical thickness and volume is associated with improved PTSD symptoms following prolonged exposure (Bryant et al., Reference Bryant, Felmingham, Whitford, Kemp, Hughes, Peduto and Williams2008). Collectively, our results in combination with the extant literature indicate that pgACC connectivity and structure play an integral role in psychological intervention improvement. Such improvement may be through the role of the pgACC in cognitive or emotion regulation of internally-based cognitions, such as rumination and traumatic memories (Palomero-Gallagher et al., Reference Palomero-Gallagher, Hoffstaedter, Mohlberg, Eickhoff, Amunts and Zilles2019).
The fourth and fifth subgroup connectivity difference involved the pMCC. The midcingulate cortex (MCC) is located on the dorsal portion of the rostral cingulate cortex and is divided into anterior and posterior (pMCC) subdivisions (Vogt et al., Reference Vogt, Nimchinsky, Vogt and Hof1995). A meta-analysis revealed that the pMCC and pgACC are consistently activated during negative affective states and pain (Shackman et al., Reference Shackman, Salomons, Slagter, Fox, Winter and Davidson2011). Furthermore, low pMCC connectivity with the amygdala prospectively predicts increased PTSD severity (Belleau et al., Reference Belleau, Ehret, Hanson, Brasel, Larson and DeRoon-Cassini2020), and PTSD patients show increased putamen activity when experiencing thermal pain, aversive images, and when experiencing an electric shock as an unconditioned stimulus during a fear learning task (Elman et al., Reference Elman, Upadhyay, Langleben, Albanese, Becerra and Borsook2018; Geuze et al., Reference Geuze, Westenberg, Jochims, de Kloet, Bohus, Vermetten and Schmahl2007; Linnman, Zeffiro, Pitman, & Milad, Reference Linnman, Zeffiro, Pitman and Milad2011). Here, we observed that subgroup 1 had weaker pgACC to pMCC and had a putamen to pMCC connectivity path, providing evidence for a circuit centered in the pMCC implicated in increased negative affect and pain that when less connected may also impair emotional processing – a necessary component of prolonged exposure therapy (Foa, Hembree, Rothbaum, & Rauch, Reference Foa, Hembree, Rothbaum and Rauch2019).
Biomarkers to aid diagnosis and treatment response are few, potentially requiring patients to cycle through multiple failed treatment trials, increasing suffering and resource expenditures. Neuroimaging-based biotyping has had recent success in predicting external measures of functioning and clinical outcomes using standard functional connectivity (Drysdale et al., Reference Drysdale, Grosenick, Downar, Dunlop, Mansouri, Meng and Liston2017; Etkin et al., Reference Etkin, Maron-Katz, Wu, Fonzo, Huemer, Vértes and O'Hara2019) and with effective connectivity measures (Li et al., Reference Li, Zhu, Jiang, Jin, Zhang, Guo and Liu2014; Price et al., Reference Price, Beltz, Woody, Cummings, Gilchrist and Siegle2020). Leveraging data-driven approaches to biotype clinical heterogeneity may better capture the complex symptom presentation of PTSD/AUD and thus be a more robust treatment response biomarker and aid in clinical decision making. Advancements in analytic strategies and methodological tools that allow for the measurement and manipulation (e.g. pharmacological or brain stimulation) of causal network relationships will be critical to enhance the clinical utility of neuroimaging (Reid et al., Reference Reid, Headley, Mill, Sanchez-Romero, Uddin, Marinazzo and Cole2019).
Limitations
There are limitations that will need to be addressed in future studies. First, our sample was restricted to a clinical sample of a mostly male Veteran PTSD/AUD patient population. We also did not include a healthy control group or separate PTSD and AUD groups to determine whether the network connectivity patterns observed here reflect disordered connectivity. Although our findings were specific to PTSD symptoms, it will be necessary to examine if the connectivity-derived subgroups and connectivity paths generalize to women and non-Veteran populations in addition to testing the specificity of effective connectivity-derived profiles in PTSD patients without AUD and in PTSD patients with other comorbidities. Second, our overall sample size is small, limiting our ability to probe how the within-subgroup connectivity paths may relate to clinical and behavioral outcomes and generalizability. However, our sample size is consistent with other recent neuroimaging investigations of psychotherapy outcomes in singly diagnosed individuals (sample size <45) (Bryant et al., Reference Bryant, Erlinger, Felmingham, Malhi, O'Donnell, Williams and Korgaonkar2020; Reggente et al., Reference Reggente, Moody, Morfini, Sheen, Rissman, O'Neill and Feusner2018; Zhutovsky et al., Reference Zhutovsky, Thomas, Olff, van Rooij, Kennis, van Wingen and Geuze2019) and S-GIMME simulation work shows robust model fits and a reduction of model overfitting even at small sample sizes as low as 25 (Gates et al., Reference Gates, Lane, Varangis, Giovanello and Guskiewicz2017). Nonetheless, further work with high-powered samples, multi-site clinical trials, and validation datasets will be required to determine the replicability and validity of the subtypes observed in our study and their treatment implications (Poldrack, Huckins, & Varoquaux, Reference Poldrack, Huckins and Varoquaux2020). Third, a limitation of effective connectivity analysis, and to multivariate analysis more broadly, is that variable selection can bias model fits when estimating directional relationships and the results are dependent on the variables entered into the model (Shi, Westerhuis, Rosén, Landberg, & Brunius, Reference Shi, Westerhuis, Rosén, Landberg and Brunius2019). Here, we used a targeted, hypothesis-driven approach for variable selection. The ROIs that we selected were based on prior research by our group that showed relevant brain regions for PTSD or AUD (Klaming et al., Reference Klaming, Harlé, Infante, Bomyea, Kim and Spadoni2019). A direction for future research will be to broaden the brain regions selected for subtyping to further identify clinically relevant neural circuits involved in benefiting from psychological interventions. Fourth, we did not find evidence of a biotype or connectivity path associated with change in alcohol use. It is unclear if AUD outcomes are separately detectable in the presence of PTSD symptomatology. The high baseline level of heavy alcohol use and low baseline variability in this measure may account for the absence of findings related to alcohol outcomes.
Conclusion
Our study provides novel evidence that the capacity to benefit from psychological interventions in patients with comorbid PTSD/AUD may involve balanced communication between key nodes from networks important for the integration of affect and cognition. Although our results do not provide direct clinical guidance, they do show that patients can be subtyped based on clinically relevant brain network relationships – providing a deeper understanding of the neural mechanisms contributing to symptom improvement that can be tested in future investigations. These results raise the possibility that recruiting clinically complex patient samples and leveraging data-driven network analyses may provide an unprecedented level of insight into the characterization of comorbid PTSD/AUD and identify brain network profiles that are associated with benefiting from standard treatments.
Supplementary material
The supplementary material for this article can be found at https://doi.org/10.1017/S0033291721001513.
Acknowledgements
The views expressed in this article are those of the authors and do not necessarily reflect the position or policy of the Department of Veterans Affairs or the United States government.
Financial support
This work was supported by the VA Center of Excellence for Stress and Mental Health (all authors). DMS is supported by a VA Clinical Science Research and Development Career Development Award (IK2 CX001861). KMH is supported by a VA Clinical Science Research and Development Career Development Award (IK2 CX001584). SBN is supported by a VA Clinical Science Research and Development Merit Grant (I01 RX002412). ANS is supported by a VA Clinical Science Research Merit Award (I01 CX001542). ADS is supported by a VA Clinical Science Research and Development Merit Grant (I01 CX001762). The current work was supported by a VA Clinical Science Research and Development Career Development Award (IK2 CX000864) (ADS), with parent study support from a VA Clinical Science Research Merit Award (I01 CX000756) (SBN).
Conflict of interest
None.
Ethical standards
The authors assert that all procedures contributing to this work comply with the ethical standards of the relevant national and institutional committees on human experimentation and with the Helsinki Declaration of 1975, as revised in 2008.