No CrossRef data available.
Article contents
Denoising Large In Situ TEM Image Datasets: A Convolutional Neural Network-based Approach
Published online by Cambridge University Press: 30 July 2020
Abstract
An abstract is not available for this content so a preview has been provided. As you have access to this content, a full PDF is available via the ‘Save PDF’ action button.
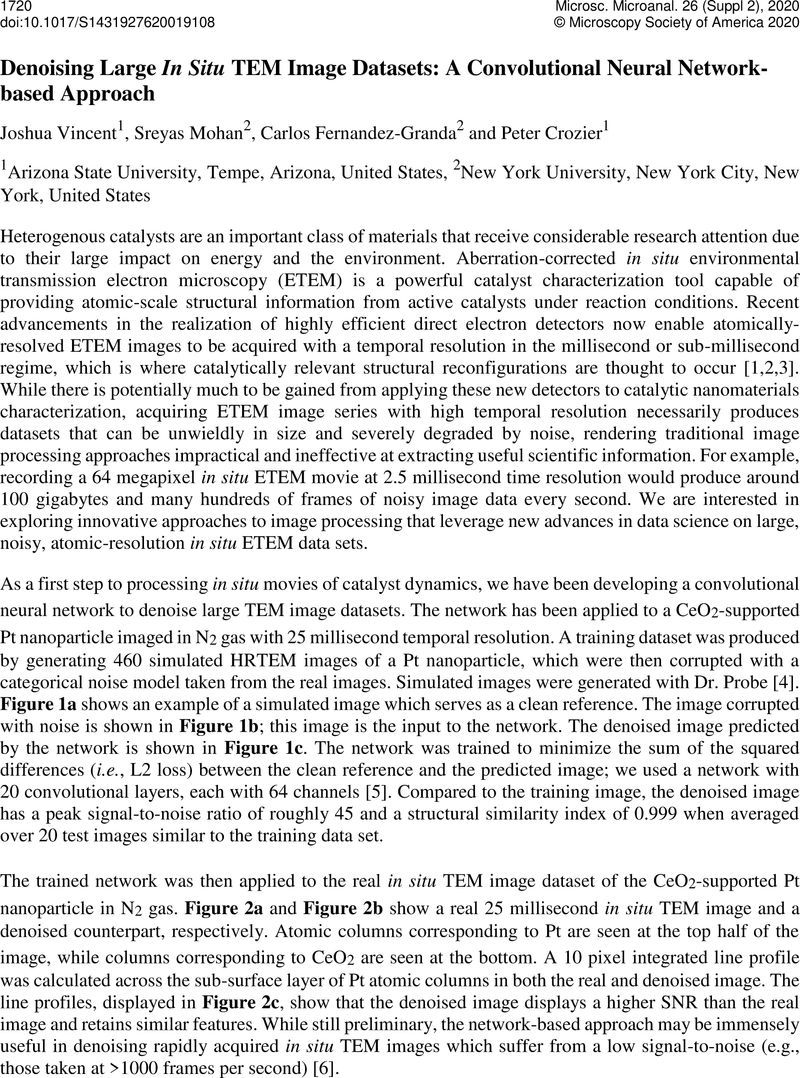
- Type
- Advances in Modeling, Simulation, and Artificial Intelligence in Microscopy and Microanalysis for Physical and Biological Systems
- Information
- Copyright
- Copyright © Microscopy Society of America 2020
References
Crozier, P. A., et al. ., Microscopy and Microanalysis, 2019. 25(S2): p. 1464-1465.10.1017/S1431927619008055CrossRefGoogle Scholar
Faruqi, A. and McMullan, G., Nucl. Instrum. Methods Phys. Res, 2018. 878: p. 180-190.10.1016/j.nima.2017.07.037CrossRefGoogle Scholar
Barthel, J., Ultramicroscopy, 2018. 193, p. 1-11.10.1016/j.ultramic.2018.06.003CrossRefGoogle Scholar
Mohan, S., Kadkhodaie, Z., Simocelli, E. P., and Fernandez-Granda, C., 2019. Robust and Interpretable Blind Image Denoising via Bias-Free Convolutional Neural Networks, preprint available at https://arxiv.org/abs/1906.05478Google Scholar
We gratefully acknowledge support of NSF grants CBET-1604971 and OAC-1940263, and the facilities at ASU's John M. Cowley Center for High Resolution Electron Microscopy.Google Scholar