No CrossRef data available.
Article contents
Automatic Nondestructive Detection of Damages in Thermal Barrier Coatings Using Image Processing and Machine Learning
Published online by Cambridge University Press: 22 July 2022
Abstract
An abstract is not available for this content so a preview has been provided. As you have access to this content, a full PDF is available via the ‘Save PDF’ action button.
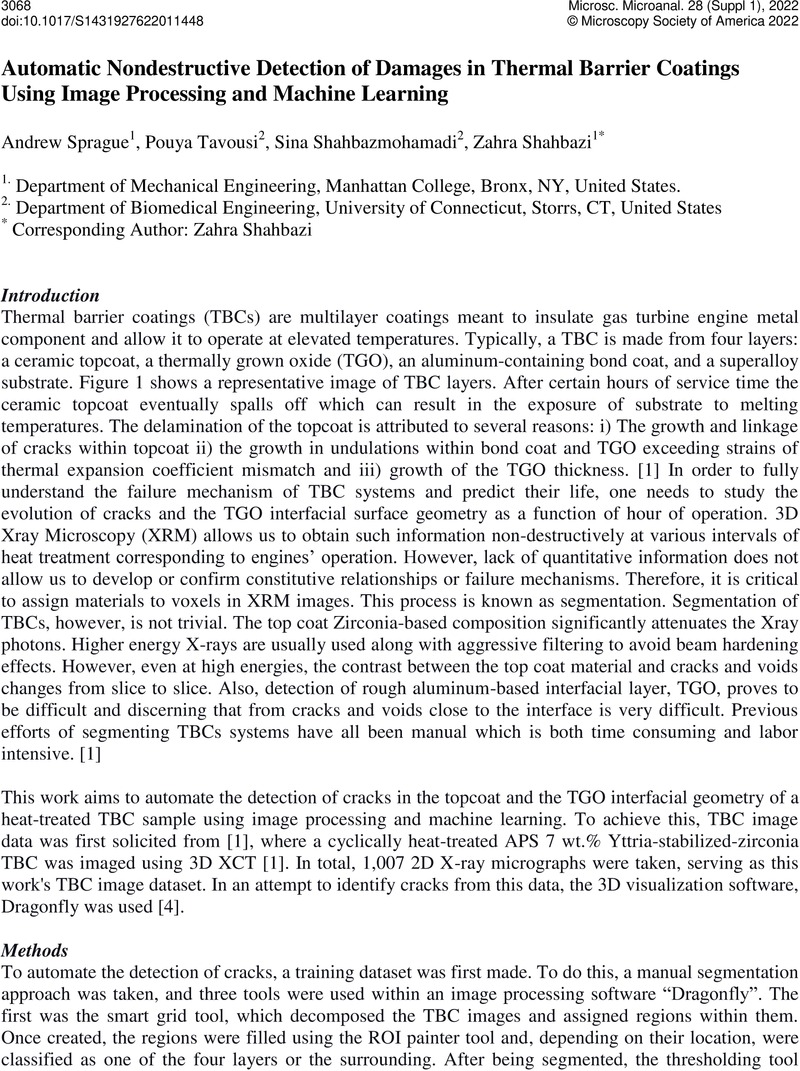
- Type
- Artificial Intelligence, Instrument Automation, And High-dimensional Data Analytics for Microscopy and Microanalysis
- Information
- Copyright
- Copyright © Microscopy Society of America 2022
References
Ahmadian, S., et al. “Three-dimensional X-ray micro-computed tomography of cracks in a furnace cycled air plasma sprayed thermal barrier coating”. (2014)10.1016/j.scriptamat.2014.10.026CrossRefGoogle Scholar
Kastner, J., Heinzl, C. “X-ray Computed Tomography for Non-destructive Testing and Materials Characterization”. (2015)10.1007/978-1-4471-6741-9_8CrossRefGoogle Scholar
Ahmadi, B., et al. “Non-destructive Automatic Die-Level Defect Detection of Counterfeit Microelectronics using Machine Vision.”Google Scholar
About Deep Learning. Dragonfly Deep Learning | About Deep Learning | ORS. (n.d.). Retrieved October 5, 2021, from https://www.theobjects.com/dragonfly/deep-learning.html.Google Scholar