Introduction
Cannabis use is prevalent in the United States (Johnston et al., Reference Johnston, Miech, O’Malley, Bachman, Schulenberg and Patrick2020) and is associated with various cognitive decrements. Because most studies are cross-sectional, differences observed between users and non-users could be due to premorbid differences as opposed to neurotoxic effects. Longitudinal studies that follow substance naïve individuals into use initiation may help to address these interpretive complexities.
Case-control studies indicate relative performance decrements in cannabis users (CU) in attention (Dougherty et al., Reference Dougherty, Mathias, Dawes, Furr, Charles, Liguori and Acheson2013; Fontes et al., Reference Fontes, Bolla, Cunha, Almeida, Jungerman, Laranjeira and Lacerda2011; Jacobus et al., Reference Jacobus, Squeglia, Infante, Castro, Brumback, Meruelo and Tapert2015; Lisdahl & Price, Reference Lisdahl and Price2012), processing speed (Jacobus et al., Reference Jacobus, Squeglia, Infante, Castro, Brumback, Meruelo and Tapert2015; Petker et al., Reference Petker, Owens, Amlung, Oshri, Sweet and MacKillop2019), and psychomotor abilities (Bolla et al., Reference Bolla, Brown, Eldreth, Tate and Cadet2002; Lisdahl & Price, Reference Lisdahl and Price2012). Relative decrements have also been observed in executive functions such as inhibitory control (Battisti et al., Reference Battisti, Roodenrys, Johnstone, Pesa, Hermens and Solowij2010; Bolla et al., Reference Bolla, Brown, Eldreth, Tate and Cadet2002; Fontes et al., Reference Fontes, Bolla, Cunha, Almeida, Jungerman, Laranjeira and Lacerda2011; Gruber, Sagar, Dahlgren, Racine, & Lukas, Reference Gruber, Sagar, Dahlgren, Racine and Lukas2012) and set-shifting (Fontes et al., Reference Fontes, Bolla, Cunha, Almeida, Jungerman, Laranjeira and Lacerda2011; Gruber, Dahlgren, Sagar, Gönenç, & Killgore, Reference Gruber, Dahlgren, Sagar, Gönenc and Killgore2012; Gruber, Sagar et al., Reference Gruber, Sagar, Dahlgren, Racine and Lukas2012; Lane, Cherek, Tcheremissine, Steinberg, & Sharon, Reference Lane, Cherek, Tcheremissine, Steinberg and Sharon2007). Findings are inconsistent for working memory and spatial processes (Becker, Collins, & Luciana, Reference Becker, Collins and Luciana2014; Harvey, Sellman, Porter, & Frampton, Reference Harvey, Sellman, Porter and Frampton2007).
A robust finding in the literature involves verbal learning and memory, typically measured by word list-learning tasks such as the Rey Auditory Verbal Learning Task (RAVLT) or the California Verbal Learning Task (Broyd, van Hell, Beale, Yucel, & Solowij, Reference Broyd, van Hell, Beale, Yucel and Solowij2016; Grant, Gonzalez, Carey, Natarajan, & Wolfson, Reference Grant, Gonzalez, Carey, Natarajan and Wolfson2003). Numerous laboratories have demonstrated verbal learning and memory decrements in adolescent and adult CU (Becker et al., Reference Becker, Collins and Luciana2014; Gonzalez et al., Reference Gonzalez, Schuster, Mermelstein, Vassileva, Martin and Diviak2012; Gruber et al., Reference Gruber, Sagar, Dahlgren, Racine and Lukas2012; Harvey et al., Reference Harvey, Sellman, Porter and Frampton2007; Solowij et al., Reference Solowij, Jones, Rozman, Davis, Ciarrochi, Heaven and Yücel2011; Tait, Mackinnon, & Christensen, Reference Tait, Mackinnon and Christensen2011) which may persist over time with continued use (Becker et al., Reference Becker, Collins, Schultz, Urošević, Schmaling and Luciana2018; Jacobus et al., Reference Jacobus, Squeglia, Infante, Castro, Brumback, Meruelo and Tapert2015), perhaps improving with abstinence (Bolla et al., Reference Bolla, Brown, Eldreth, Tate and Cadet2002; Hanson et al., Reference Hanson, Winward, Schweinsburg, Medina, Brown and Tapert2010; Scott et al., Reference Scott, Slomiak, Jones, Rosen, Moore and Gur2018).
Studies of decision-making that focus on CU and utilize measures such as the Iowa Gambling Task (IGT) are inconsistent, finding in many cases that frequent heavy users show relative weaknesses (Becker et al., Reference Becker, Collins and Luciana2014; Casey & Cservenka, Reference Casey and Cservenka2020; Fridberg et al., Reference Fridberg, Queller, Ahn, Kim, Bishara, Busemeyer and Stout2010, Grant, Chamberlain, Schreiber, & Odlaug, Reference Grant, Chamberlain, Schreiber and Odlaug2012; Moreno et al., Reference Moreno, Estevez, Zaldivar, Montes, Gutiérrez-Ferre, Esteban and Flores2012; Solowij et al., Reference Solowij, Jones, Rozman, Davis, Ciarrochi, Heaven and Yücel2012; Verdejo-Garcia et al., Reference Verdejo-Garcia, Benbrook, Funderburk, David, Cadet and Bolla2007; Whitlow et al., Reference Whitlow, Liguori, Livengood, Hart, Mussat-Whitlow, Lamborn and Porrino2004) although others report no effects (Dougherty et al., Reference Dougherty, Mathias, Dawes, Furr, Charles, Liguori and Acheson2013; Gilman et al., Reference Gilman, Calderon, Curran and Evins2015; Gonzalez et al., Reference Gonzalez, Schuster, Mermelstein, Vassileva, Martin and Diviak2012). Nearly all of these studies focus on young adults.
Several meta-analyses have attempted to resolve these discrepancies. In an analysis of 11 studies including 623 CU and 409 non- or minimal users, Grant et al. (Reference Grant, Gonzalez, Carey, Natarajan and Wolfson2003) reported a small effect of long-term cannabis consumption on learning and memory but negligible effects for other cognitive domains. Scott and colleagues (Reference Scott, Slomiak, Jones, Rosen, Moore and Gur2018) as well as Schreiner and Dunn (Reference Schreiner and Dunn2012) concurred that the largest effects are observed for learning, memory, executive functioning, processing speed, and attention. A synthesis of meta-analyses published before 2019 (Duperrouzel, Granja, Pacheco-Colón, & Gonzalez, Reference Duperrouzel, Granja, Pacheco-Colón and Gonzalez2020) concluded that among non-clinical samples of CU, decrements in the learning of new information consistently showed the largest effect sizes. Figuerido et al. (Reference Figueiredo, Tolomeo, Steele and Baldacchino2020) analyzed 13 studies, including 499 chronic cannabis users and 883 controls with minimal or no lifetime cannabis use. Chronic cannabis use was associated with relative decrements in cognitive impulsivity, flexibility, attention, short-term verbal memory, and long-term verbal memory. In another recent meta-analysis of 30 studies that included 849 adult long-term recreational CU and 764 controls (Lovell et al., Reference Lovell, Akhurst, Padgett, Garry and Matthews2020), cannabis use was associated with significant but small-magnitude decrements in executive function, learning, and memory. More moderate decrements were noted for decision making. Cannabis use duration and age of onset did not influence outcomes.
Thus, verbal learning and executive functions such as decision-making should be the focus of longitudinal studies, which are needed to assess whether cognitive differences between users and non-users predate cannabis use onset, whether age of use onset influences long-range cognitive performance, and whether use in higher amounts or frequencies over time is associated with greater performance decrements (Gonzalez, Pacheco-Colón, Duperrouzel, & Hawes, Reference Gonzalez, Pacheco-Colón, Duperrouzel and Hawes2017). The ongoing Adolescent Brain Cognitive Development (ABCD) study will contribute to the goal of following participants from no cannabis use into cannabis use initiation (Luciana et al., Reference Luciana, Bjork, Nagel, Barch, Gonzalez, Nixon and Banich2018), but post-initiation data is not yet available. Fried, Watkinson, and Gray (Reference Fried, Watkinson and Gray2005) were perhaps the first to follow participants from infancy to ages 17–21, identifying 113 participants who could be classified over time as controls, light cannabis users, heavy users, or former users. They measured IQ, processing speed, memory, attention, and abstract reasoning at ages 9–12 and again at ages 17–21. After controlling for pre-cannabis-initiation test performance as well as confounds such as parental income and education, academic history, age, sex, maternal age, and prenatal substance exposure, current heavy users had significantly lower IQs, influenced by visual processing speed, relative to non-users. They also displayed relatively poor immediate and delayed memory.
Similar to Fried et al. (Reference Fried, Watkinson and Gray2005), Meier and colleagues (Reference Meier, Caspi, Ambler, Harrington, Houts, Keefe and Moffitt2012) followed a birth cohort of 1,037 individuals, identifying 874 participants who either remained abstinent or who reported using cannabis at least 4 times a week for 1, 2, or 3+ study waves. Participants with persistent cannabis dependence exhibited greater IQ declines from ages 7 to 13 to 38 relative to non-users, even when controlling for relevant covariates. Critics suggested that the findings were due to confounding SES and personality factors (Daly, Reference Daly2013; Rogeberg, Reference Rogeberg2013) as well as sample size attenuation over time. A subsequent co-twin control study (Jackson et al., Reference Jackson, Isen, Khoddam, Irons, Tuvblad, Iacono and Baker2016) found that lower IQ scores in young adult CU were attributable to premorbid characteristics. Castellanos-Ryan, Pingault, Parent, Vitaro, Tremblay, and Séguin (Reference Castellanos-Ryan, Pingault, Parent, Vitaro, Tremblay and Seguin2017) observed an association between cannabis use and decrements in verbal IQ in a community sample of boys that was accounted for by level of educational attainment. A prospective analysis of participants in the Avon Longitudinal Study of Parents and Children found that heavy cannabis use before age 15 was associated with lower IQ when controlling for childhood IQ (Mokrysz, Landy, Gage, Munafò, Rosier, & Curran, Reference Mokrysz, Landy, Gage, Munafò, Roiser and Curran2016). This relationship was attenuated when use of other substances was controlled, with cigarette use having the most marked influence. More recently, substance use patterns and performance on a neurocognitive battery were tracked annually in a community sample over 14 years, beginning when participants were ages 12–15 (Infante, Nguyen-Louie, Worley, Courtney, Coronado, & Jacobus, Reference Infante, Nguyen-Louie, Worley, Courtney, Coronado and Jacobus2020). After accounting for age, a greater mean percent days of cannabis use was associated with worse performance on measures of inhibitory control and visuospatial functioning, but not verbal memory or processing speed. Increased alcohol use was associated with visuospatial decrements, further highlighting the importance of examining the influences of each substance.
Finally, Barthelemy et al. (Reference Barthelemy, Richardson, Heeren, Chen, Liebschutz, Forman and Rose-Jacobs2019) prospectively assessed an urban African-American sample with intrauterine substance exposure (n = 119), examining associations between various aspects of verbal learning/memory and cannabis use as it emerged over time. Individuals with cannabis use onset before age 16 showed a decline in structured verbal learning (story memory) performance between adolescence and young adulthood. Trajectories of learning ability were impacted by factors such as educational attainment, recent substance use, and presence of psychopathology.
Together, these studies affirm conclusions drawn by Gonzalez et al. (Reference Gonzalez, Pacheco-Colón, Duperrouzel and Hawes2017): even within longitudinal studies, the magnitude of observed effects of CU on cognition are modest in size (consistent with cross-sectional meta-analyses), performance decrements tend to be observed only among the heaviest or more frequent CU, and control over relevant confounds, such as sociodemographic factors and comorbid substance use, is needed. Many of the aforementioned studies had gaps of nearly 6–7 years or more between measurements of pre- and post-initiation cognition (Castellanos-Ryan et al., Reference Castellanos-Ryan, Pingault, Parent, Vitaro, Tremblay and Seguin2017; Meier et al., Reference Meier, Caspi, Ambler, Harrington, Houts, Keefe and Moffitt2012; Mokrysz et al., Reference Mokrysz, Landy, Gage, Munafò, Roiser and Curran2016). Minimizing the amount of time between measurements permits a more fine-grained analysis of when cognitive decrements emerge relative to use onset.
To examine verbal learning, memory and decision-making before versus after substance use initiation, the current study utilized data from a prospective longitudinal study that followed adolescents and young adults over 10 years, with a comprehensive neurocognitive battery and substance-use information collected every two years. We identified participants who initiated cannabis as well as alcohol use (n = 47) as well as those who initiated alcohol but not cannabis use (n = 39). Motivation for the tasks included in this analysis derives from our prior findings (Becker et al., Reference Becker, Collins and Luciana2014, Reference Becker, Collins, Schultz, Urošević, Schmaling and Luciana2018), which focused on the longitudinal progression of cognitive performance in a college sample that had already initiated cannabis use at their baseline. These daily CUs showed significant performance decrements, as compared to non-using controls, in verbal memory (RAVLT) as well as IGT-based decision-making. The relative weaknesses in memory persisted over time with continued heavy use. That analysis could not address whether decrements were evident prior to cannabis use onset or the extent to which comparable performance decrements might have been observed in equivalently heavy users of alcohol but not cannabis. Thus, the current study followed individuals from before substance use initiation into cannabis and/or alcohol use, allowing post-initiation learning, memory, and decision-making performance to be evaluated relative to pre-initiation performance for users of each substance. Based on the literature suggesting maximal impairments post-initiation in heavier or more frequent cannabis users (Gonzalez et al., Reference Gonzalez, Pacheco-Colón, Duperrouzel and Hawes2017), we hypothesized that more frequent cannabis users would produce significantly lower RAVLT and IGT scores relative to alcohol-only and infrequent cannabis users.
Methods
The study was approved by the University of Minnesota’s human subjects committee (protocol 0405M59982). Participants ranged in age from 9 to 23 years at baseline, and were invited to complete four subsequent assessments, spaced approximately two years apart. For minors, families were recruited through a community database maintained by the University of Minnesota Institute of Child Development. When their child was born, parents throughout the metro area indicated an interest in participating in University-sponsored research, allowing adolescents to be identified within the database. Additionally, invitation postcards were mailed to nonacademic University employees who might be parents. Young adults (aged 18+) were recruited through community postings.
At baseline, families completed a phone screening followed by an in-person clinical assessment (Kiddie-SADS-Present and Lifetime Version (KSADS-PL): Kaufman, Birmaher, Brent, Rao, & Ryan, Reference Kaufman, Birmaher, Brent, Rao and Ryan1996) that determined study eligibility. The KSADS-PL is a semi-structured interview with excellent psychometric properties, administered in this study to the youth and parent, that assesses developmental and social history as well as Axis-I childhood and adult disorders as defined by DSM-IV (American Psychiatric Association, 2000). Baseline exclusions included histories of neurological or psychiatric disorders, preterm birth, other birth complications, current or past substance abuse, prior head injury, learning disabilities, current psychoactive prescription use, non-native English speaking, and uncorrected vision/hearing. As this study also involved neuroimaging (not presented here), non-right-handers and those with imaging contraindications were excluded.
At baseline, 197 individuals were enrolled. Substance use was assessed using the Personal Experiences Inventory (PEI: Henley & Winters, Reference Henley and Winters1989), the Achenbach Youth and Adult Self-Report scales (Achenbach & Rescorla, Reference Achenbach and Rescorla2001, Reference Achenbach and Rescorla2003), and the KSADS-PL. The KSADS-PL includes screening questions that query presence/absence of specific substances used as well as more comprehensive questions about problematic use if an individual reports regular ingestion of a given substance. The Achenbach scales include questions about amounts of nicotine used daily in the past six months. The PEI self-report questionnaire queries substance use frequencies, including alcohol and cannabis, for the prior 5 years (or lifetime for adolescents), prior 12 months, and prior 3 months. Participants indicated on a five-point scale the frequency of use within the designated time period. Responses to the adolescent and adult PEI versions were harmonized to eliminate minor differences in response formats. Values of 0 indicated no use of a particular substance; 1 = 1–5 uses; 2 = 6–20 uses; 3 = 21–49 uses; 4 = 50–99 uses; 5 = over 100 uses within the specified time period.
Verbal learning and memory were assessed at each study wave with the Rey Auditory Verbal Learning Test (RAVLT: Rey, Reference Rey1993) under standardized conditions. Participants were read a 15-word list over 5 learning trials and were instructed to state as many words as they could remember following each trial. Following five learning trials, an interference trial of 15 different words (List B) was presented and recalled, followed by immediate recall of words from the first list. Thirty minutes later, participants recalled words from the first list (delayed recall). Alternate forms with different word lists were used across study waves.
Performance metrics reflected verbal learning (total correct recollections across the first five learning trials), new learning (performance on the first trial as well as the List B interference trial, averaged), immediate recall, and 30-minute delayed recall. Delayed recall divided by the total correct on the last learning trial (Trial 5) was calculated to quantify the proportion of information consolidated over time (Takagi et al., Reference Takagi, Yücel, Cotton, Baliz, Tucker, Elkins and Lubman2011).
Participants completed a computerized 100-trial variant of the Iowa Gambling Task (IGT: Almy, Kuskowski, Malone, Myers, & Luciana, Reference Almy, Kuskowski, Malone, Myers and Luciana2018; Hooper, Luciana, Conklin, & Yarger, Reference Hooper, Luciana, Conklin and Yarger2004), a measure of feedback-guided decision making. Participants began with a fixed amount of loaned money and then selected from four simulated card decks on each trial. Participants received feedback on monetary gains and losses from each choice. Choices were unlimited from each deck. Selections from two decks resulted in long-term monetary gains (advantageous decks). Selections from two decks resulted in long-term losses (disadvantageous decks). Deck contingencies are described in Almy et al. (Reference Almy, Kuskowski, Malone, Myers and Luciana2018). Participants kept earned winnings, which did not exceed $5.00. The layout of the four decks was shuffled across study waves.
Performance metrics included the difference between the participants’ total number of advantageous and disadvantageous deck choices (a) across all 100 trials as well as (b) within the first 40 trials, summed, which reflect decision-making under ambiguity, and (c) the last 60 trials, summed, which reflect decision-making under risk (Almy et al., Reference Almy, Kuskowski, Malone, Myers and Luciana2018). For the RAVLT and IGT, raw scores were used in the analyses.
IQ was estimated from performance on the full Wechsler Abbreviated Scale of Intelligence (WASI: Wechsler, Reference Wechsler1999). Demographic data, including socioeconomic, health, and family information, were collected from parents (for minor participants) and from participants (if over age 18).
Statistical approach
Data were analyzed using SPSS version 26 for Windows. After examining participants’ reported past five years’ cannabis and alcohol usage levels across assessments using the PEI and KSADS, participants were identified who completed more than one assessment (n = 168 of 197). Of these, n = 99 were substance naïve at baseline, and either initiated substance use during the course of the study (n = 86) or remained substance naïve (n = 13). This analysis focuses on the 86 individuals who initiated substance use. Those classified as persistent non-users were not included, because the sample is small, they were younger at study intake than the substance use initiators, and they completed significantly fewer assessment waves. Of 86 substance use initiators, n = 39 initiated use of alcohol but not cannabis; n = 47 initiated cannabis use. The study wave at which use initiation was detected was recorded as the post-initiation time point. The study wave prior to that one was recorded as the pre-initiation time point. Of those who initiated cannabis use, all also initiated alcohol use. Those who initiated cannabis use comprised two groups (Fried, Watkinson, James, & Gray, Reference Fried, Watkinson, James and Gray2002; Fried et al., Reference Fried, Watkinson and Gray2005; Gonzalez et al., Reference Gonzalez, Pacheco-Colón, Duperrouzel and Hawes2017) based on their frequencies of reported use: infrequent cannabis users (n = 29) and moderately frequent cannabis users (n = 18). Infrequent cannabis users transitioned from no reported use at one time point to 1–5 uses within the past year at the post-initiation time point. Moderately frequent cannabis users transitioned from no use at one time point to six or more uses within the past year post-initiation. Longitudinal retention was generally acceptable. Of the 86 substance use initiators, n = 63 were retained through the last assessment (wave 5) of the study, n = 72 were retained through wave 4, n = 81 were retained through wave 3, and n = 86 were retained through wave 2.
For alcohol initiators (n = 39), 25 were retained through wave 5; 32 were retained through wave 4, 35 were retained through wave 3, and 39 were retained through wave 2. For less frequent marijuana initiators (n = 29), 24 were retained through wave 5, 26 were retained through wave 4, and 28 were retained through wave 3, and 29 were retained through wave 2. For more frequent marijuana initiators (n = 18), 14 were retained through wave 5, 15 were retained through wave 4, 17 were retained through wave 3, and 18 were retained through wave 2.
Table 1 indicates when in the course of the study alcohol and cannabis use initiation were detected in each group.
Table 1. Substance use initiation patterns across time by group

Values represent numbers of individuals who reported substance use initiation at each assessment wave post-baseline. All were substance naïve at the Wave 1 baseline assessment.
The groups were compared using one-way analyses of variance on age, IQ, parental (average of mother and father) years of education, family income, number of study visits before substance use initiation was identified, and substance use frequencies after initiation. Gender and race/ethnicity distributions were compared between groups using chi-square tests.
RAVLT and IGT performance metrics were based on raw scores and were analyzed as follows. Pre-initiation performance was contrasted between groups using generalized linear models with cognitive performance as a univariate factor and group as a between-subjects factor. Parental education was covaried (rationale below). The difference between pre-initiation and post-initiation performance was calculated for each cognitive variable of interest and contrasted by group using ANCOVA; covariates included pre-initiation performance and parental education. As planned follow-up tests for each ANCOVA, a priori contrasts on the estimated marginal means were conducted pairwise between groups, with Bonferroni adjustment of the nominal p < .05 threshold for statistical significance.
In addition, hierarchical linear regressions were utilized for the full sample of substance use initiators, regardless of group status, to assess the extent to which quantitative frequencies of cannabis, alcohol, and nicotine use contributed to observed cognitive function post-initiation, controlling for pre-initiation performance levels as well as parental education.
Results
Sample characteristics
As indicated in Table 2, the 86 substance use initiators were from middle to upper-middle class backgrounds and had estimated IQs in the above average range. When sub-grouped, those who were infrequent versus moderately frequent users of cannabis were comparable in gender distribution, pre-initiation age, post-initiation age, ethnic composition, estimated IQ scores, self-reported frequencies of alcohol use in the past year, and the number of study visits prior to the capture of substance use initiation. Parental education levels were significantly lower for moderately frequent cannabis users versus infrequent cannabis users. Nicotine use was higher in the moderately frequent cannabis users post-initiation as compared to the other groups but was infrequent overall: only 7 of 86 individuals reported any nicotine use. Cannabis use frequencies differed between groups. Alcohol-only users as compared to cannabis users had higher levels of parental education, higher family incomes, and lower frequencies of alcohol, nicotine, and cannabis use. Thus, the groups varied in frequencies of alcohol, nicotine, and cannabis use but also in parental education.
Table 2. Demographics: alcohol and cannabis use initiators
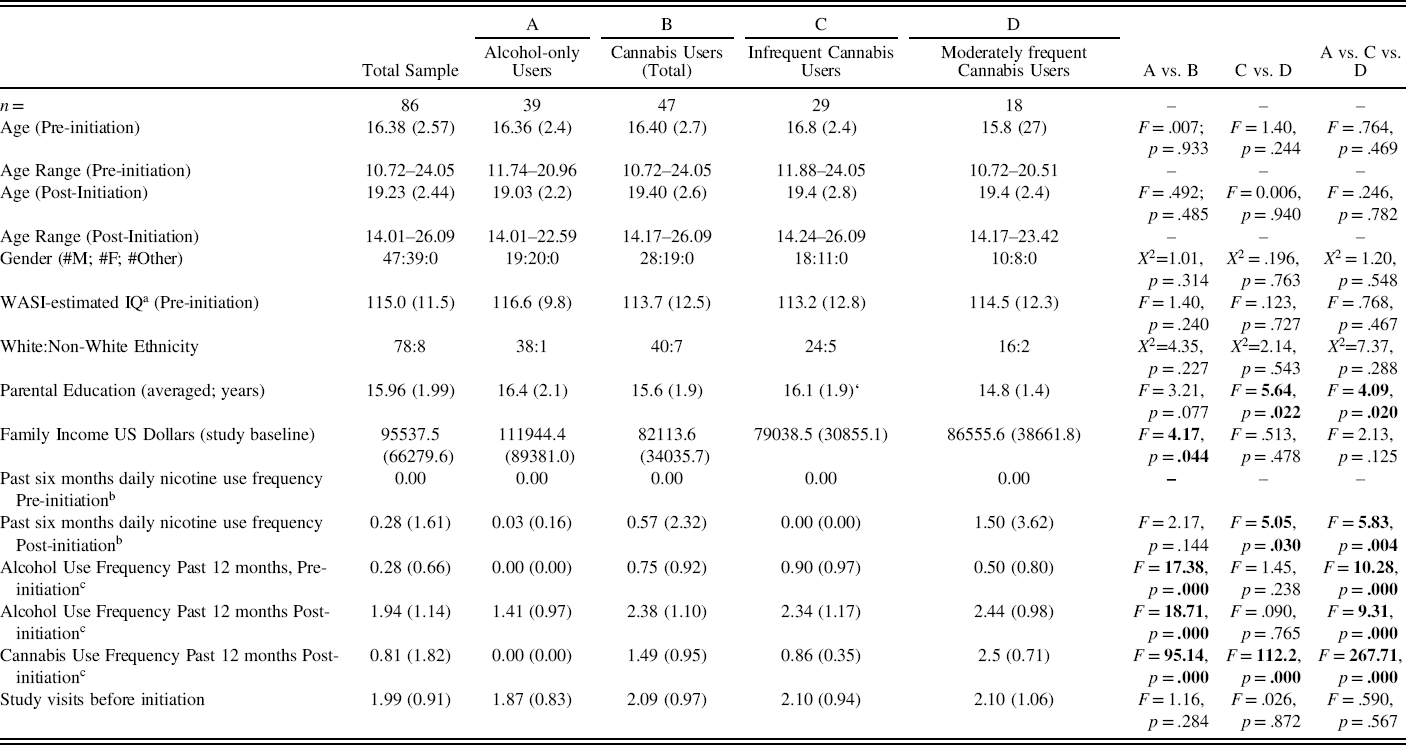
Unless otherwise indicated, values represent means and, in parentheses, one standard deviation.
aWASI-estimated IQs are based on four subtests: Vocabulary, Matrix Reasoning, Similarities, and Block Design.
bNicotine use = the number of reported uses per day in the past six months assessed by the Achenbach self-report inventories.
cAlcohol and cannabis use frequencies for the twelve months prior to each assessment were assessed with the Personal Experiences Inventory (PEI): 0=0 use; 1=1–5 times; 2 = 6–20 times; 3 = 21–49 times; 4=50–99 times.
Bivariate correlations between demographic variables and task performance are shown for descriptive purposes in Table 3. Pre-initiation task performance was examined in relation to demographic variables. Parental education was marginally associated with the RAVLT total learning score (summed performance across trials 1–5: r = .201, p = .067) and significantly associated with the IGT total number of advantageous relative to disadvantageous choices (r = .418, p = .000). Age was also associated with this IGT total score (r = .226, p = .040) but not with RAVLT outcomes. IQ was significantly associated with IGT performance (r = .295, p = .007) but not with RAVLT outcomes.
Table 3. Bivariate correlations between demographic variables and pre-initiation RAVLT and IGT performance metrics

* two-tailed p < .05; ** two-tailed p < .01; Values represent Pearson r coefficients.
Pre-initiation cognitive performance
Pre-initiation task performance was contrasted between groups using ANCOVAs, controlling for parental education (Table 4). RAVLT new learning (the average of Trial 1 and List B total correct raw scores) differed between groups: F (2,80) = 3.79, p = .027, η p 2 = .09. Follow-up pairwise contrasts indicated that those who subsequently initiated moderately frequent cannabis use produced lower scores than those who initiated alcohol-only (p = .039) as well as infrequent cannabis (p = .045) use (see Figure 1).
Table 4. Pre- and post-initiation neuropsychological test scores for participants who initiated infrequent use of cannabis, moderately frequent use of cannabis, and use of alcohol but not cannabis
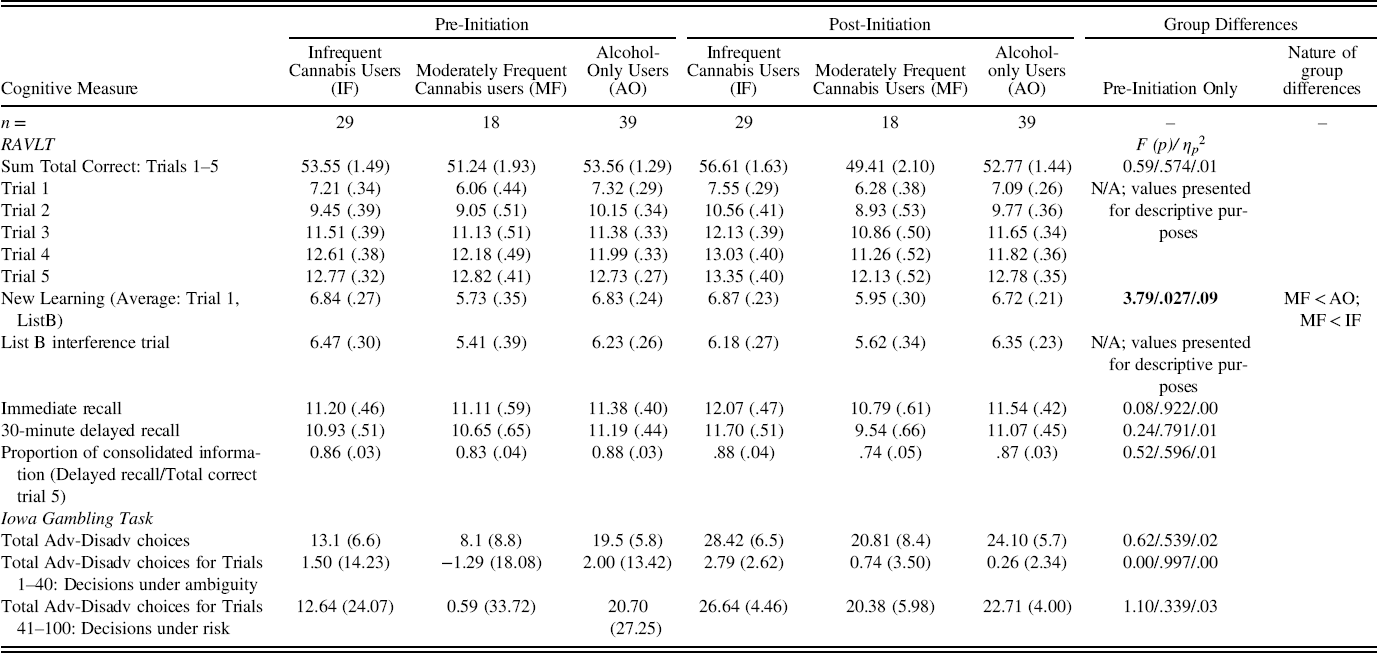
Notes. Estimated marginal means and standard errors are presented, controlling for parental education. Comparisons where p-values are statistically significant (p<.05) are bolded. Unless otherwise specified, values represent raw total correct scores for each trial of the RAVLT; raw scores were also used for IGT calculations.
Change in cognitive performance with substance use initiation
When change scores were similarly contrasted between the groups (Table 5), group differences were observed in total verbal learning (sum of raw scores across the five RAVLT learning trials), F(2,78) = 3.70, p = .03, η p2 = .09, with declines in performance over time in the moderately frequent cannabis users relative to infrequent cannabis users (p = .04).
Table 5. Pre- and post-initiation difference scores by group

Estimated marginal means and standard errors are presented, controlling for parental education and pre-initiation performance.
Comparisons where p-values are statistically significant (p<.05) are bolded.
The magnitude of change over time in 30-minute delayed recall also varied by group, F(2,78) = 4.55, p = .014, η p2 = .10. The moderately frequent cannabis users showed declines in delayed recall over time relative to infrequent cannabis users (p = .011). Neither group differed from alcohol-only users (Figure 2). Accordingly, we examined the post-initiation proportion of learned information consolidated over time (total raw score for delayed recall divided by total raw score for Trial 5). The proportion of consolidated information differed between groups, F(2,79) = 3.45, p = .037, η p2 = .08, with moderately frequent cannabis users performing marginally worse (retaining M = .74, SE = .05 of learned information) than each of the other two groups (alcohol-only users: M = .87, SE = .03, p = .064; infrequent cannabis users: M = .88, SE = .04, p = .051). As indicated in Table 5, this finding is reduced in significance (p = .053) when difference scores are analyzed.

Fig. 1. Pre-initiation RAVLT new learning scores were calculated by averaging the total raw correct responses to trial 1 and List B. Values represent estimated marginal means ± the 95% confidence interval. As indicated, there was a main effect of group on performance with worse performance in the moderately frequent cannabis users relative to alcohol-only users (p = .039) and infrequent cannabis users (p = .045).

Fig. 2. Differences between pre- and post-initiation RAVLT 30-minute delayed recall scores are presented for each group, controlling for parental education and pre-initiation performance. Values are estimated marginal means ± the 95% confidence interval. Positive scores reflect increases over time; negative scores reflect decreases over time. Those who initiated moderately frequent use of cannabis showed declines in performance from pre-to-post-initiation relative to less frequent users of cannabis (p = .011).
IGT performance was evaluated by analyzing group differences in decision-making performance (total number of advantageous minus disadvantageous choices) over all 100 trials both before and after substance use initiation. Performance under conditions of initial ambiguity (first 40 trials) and under conditions of known risk (last 60 trials) was also evaluated (Almy et al., Reference Almy, Kuskowski, Malone, Myers and Luciana2018). No significant group differences were detected (Tables 4 and 5).
Contributions of cannabis, alcohol, and nicotine use to post-initiation RAVLT performance
Hierarchical regressions using continuous substance-use variables, as compared to ANCOVA groupings, confirmed that the observed effects were due to levels of cannabis and not alcohol use. Nicotine use exerted an effect on some performance variables. Analyses focused on the RAVLT total learning score across trials 1–5, RAVLT new learning, RAVLT 30-minute delayed recall total correct, and the RAVLT proportion of consolidated information. For each of these analyses, the dependent variable was post-initiation level of performance. Step 1 predictors included parental education and pre-initiation levels of performance. Step 2 predictors included post-initiation alcohol use and post-initiation nicotine use. Step 3 predictors included post-initiation cannabis use. See Table 6 for full statistics.
Table 6. Prediction of post-initiation learning and memory performance from substance use frequencies

For the analysis of new learning, Step 1 model was significant, but Steps 2 and 3 were not incrementally predictive. Significant unique predictors of better post-initiation performance included higher parental education and higher pre-initiation performance levels. For the total words learned across trials 1–5, Step 3 model was incrementally significant. Significant unique predictors of better post-initiation performance included higher levels of pre-initiation performance as well as lower frequencies of cannabis use. For 30-minute delayed recall as well as proportion of information retained over time, again Step 3 model was incrementally significant. Significant unique predictors of better post-initiation performance for both delayed memory variables included better pre-initiation performance as well as lower frequencies of cannabis use. Additionally, a lower frequency of nicotine use was associated with a greater proportion of information consolidated over the RAVLT recall period (p = .047) and with the absolute level of delayed recall (p = .037). Alcohol use frequency was not a significant independent predictor of any performance variable.
Discussion
Although many studies provide evidence of relative decrements in cognition in recreational cannabis users, few have followed adolescents from a substance naïve pre-initiation period into the initiation of use. Fewer still have distinguished the effects of cannabis, nicotine, and alcohol use on cognitive outcomes even though cannabis users frequently report use of other substances (Mejia, Wade, Baca, Diaz, & Jacobus, Reference Mejia, Wade, Baca, Diaz and Jacobus2021; Patrick, Terry-McElrath, Lee, & Schulenberg, Reference Patrick, Terry-McElrath, Lee and Schulenberg2019). This study expanded upon our prior analyses of cognition in daily cannabis users (Becker et al., 2014, Reference Becker, Collins, Schultz, Urošević, Schmaling and Luciana2018) and focused on the Rey Auditory Verbal Learning Task, a commonly used measure of verbal learning and memory, as well as the Iowa Gambling Task, a measure of feedback-guided decision-making.
Concordant with recent reviews and meta-analyses (Broyd et al., Reference Broyd, van Hell, Beale, Yucel and Solowij2016; Lovell et al., Reference Lovell, Akhurst, Padgett, Garry and Matthews2020; Schoeler, Kambeitz, Behlke, Murray, & Bhattacharyya, Reference Schoeler, Kambeitz, Behlke, Murray and Bhattacharyya2016) indicating that decrements in verbal learning and memory performance are among the most consistently observed cognitive effects in the context of cannabis use and that these functions may be longitudinally impacted by use (Gonzalez et al., Reference Gonzalez, Pacheco-Colón, Duperrouzel and Hawes2017), we observed that overall verbal learning across the first five trials of the RAVLT was diminished after the onset of moderately frequent cannabis use, after controlling for parental education and premorbid variations. The observed performance decrements were not associated with post-initiation alcohol or nicotine use, as suggested by our regression analyses, which was important to demonstrate given that alcohol use was associated with deviations in RAVLT-based verbal learning in a recent co-twin control analysis of adolescent users (Malone, Wilson, Bair, McGue, & Iacono, Reference Malone, Wilson, Bair, McGue and Iacono2020). Moderately frequent cannabis users also showed pre-initiation decrements in new learning performance, consistent with the idea that those with poor baseline executive function, such as working memory, are vulnerable to behavioral dysregulation including reduced control of substance use (Khurana, Romer, Betancourt, & Hurt, Reference Khurana, Romer, Betancourt and Hurt2017; Kim-Spoon et al., Reference Kim-Spoon, Kahn, Lauharatanahirun, Deater-Deckard, Bickel, Chiu and King-Casas2017).
Learning ability, as well as working memory, can be impacted by general ability (Mohn, Sundet, & Rund, Reference Mohn, Sundet and Rund2014) and by age, though it should be emphasized that participants had mean estimated IQs in the above-average range, and age did not vary between groups. Moreover, age and IQ were not associated with RAVLT performance. However, parental education significantly predicted RAVLT new learning and total learning performance, and parental education levels were lower particularly for those who reported moderately frequent cannabis use. To explain the pattern that we observed, one might speculate that additional pre-initiation factors may have impacted performance. For instance, higher levels of state anxiety that contribute to higher frequencies of cannabis use (e.g., as self-medication) could interfere with learning and memory performance on more challenging tasks. However, recent reviews and empirical assessments challenge this notion and suggest that, in fact, verbal memory tests are not markedly vulnerable to test anxiety in neuropsychological patients (Gass & Curiel, Reference Gass and Curiel2011; Dorenkamp & Vik, Reference Dorenkamp and Vik2018) nor are they vulnerable to the impacts of acute stressors (Hoffman & al’Absi, Reference Hoffman and al Absi2004) in healthy volunteers. A critical difference between those studies and the present study is that they focused on adult samples. Accordingly, additional studies addressing whether test anxiety might impact verbal memory performance in adolescence and young adults are needed.
A major finding from this study is our observation of relatively greater post-initiation declines in delayed memory recall following list learning in moderately frequent cannabis users, a decline that was not observed in infrequent cannabis users and alcohol users. This finding is consistent with cross-sectional work (Jacobus et al., Reference Jacobus, Squeglia, Infante, Castro, Brumback, Meruelo and Tapert2015; Solowij et al., Reference Solowij, Jones, Rozman, Davis, Ciarrochi, Heaven and Yücel2011) as well as Barthelemy et al. (Reference Barthelemy, Richardson, Heeren, Chen, Liebschutz, Forman and Rose-Jacobs2019) who also observed verbal memory declines over time in adolescent users. The finding of a decrement in only the most frequent users of cannabis provides evidence in support of a dose–response association as suggested by others (Gonzalez et al., Reference Gonzalez, Pacheco-Colón, Duperrouzel and Hawes2017). Moreover, the finding of worse verbal memory after but not before substance use initiation adds interpretive clarity to our prior observation of similar decrements in chronic users (Becker et al., Reference Becker, Collins, Schultz, Urošević, Schmaling and Luciana2018). Poor verbal memory in the context of daily life might contribute to the adverse educational and psychosocial outcomes frequently observed in adolescent CU (Silins et al., Reference Silins, Horwood, Patton, Fergusson, Olsson, Hutchinson and Mattick2014). While Infante et al. (Reference Infante, Nguyen-Louie, Worley, Courtney, Coronado and Jacobus2020) did not observe decrements in verbal learning or memory as a function of alcohol or cannabis use in their 14-year follow-up of adolescent users, that finding was unexpected and the length of the follow-up period may have impacted their findings.
Leveraging continuous substance use-frequency variables, we supported the observed between-group differences with regression analyses indicating that, independent of group membership, post-initiation frequencies of cannabis use predicted worse verbal memory performance independently of both alcohol use frequencies and pre-initiation performance levels. Higher levels of nicotine use also conferred verbal memory disadvantages, but in the current study, nicotine use was rare and largely comorbid with cannabis use in smokers/vapers, thus the study was not optimized to fully dissociate effects of the two substances. Co-use of nicotine is common in cannabis-using adolescents, often at higher levels than we observed (Mejia et al., Reference Mejia, Wade, Baca, Diaz and Jacobus2021; Kennedy, Caraballo, Rolle, & Rock, Reference Kennedy, Caraballo, Rolle and Rock2016; Schauer, Berg, Kegler, Donovan, & Windle, Reference Schauer, Berg, Kegler, Donovan and Windle2016). A recent review (Mejia et al., Reference Mejia, Wade, Baca, Diaz and Jacobus2021) noted that although attempts have been made to isolate independent effects of each substance, focused studies of co-users are limited currently with only 9 human and three preclinical studies available for analysis. Three human studies of adolescent or young adult users (Hindocha, Freeman, Xia, Shaban, & Curran, Reference Hindocha, Freeman, Xia, Shaban and Curran2017; Jacobsen, Pugh, Constable, Westerveld, & Mencl, Reference Jacobsen, Pugh, Constable, Westerveld and Mencl2007; Schuster, Crane, Mermelstein, & Gonzalez, Reference Schuster, Crane, Mermelstein and Gonzalez2015) suggest that nicotine use may, under some conditions, exert a protective effect on episodic memory processes in CU, particularly when used proximal to the time of cognitive testing. This is not the pattern of results that we observed, and the nicotine-using participants in the current study were abstinent on the day of testing. Preclinical evidence is more mixed, suggesting that females may be more vulnerable to adverse effects of co-use on memory processes (Mateos et al., Reference Mateos, Borcel, Loriga, Luesu, Bini, Llorente and Viveros2011). A more comprehensive effort to dissociate the impacts of nicotine and cannabis co-use is clearly needed, particularly given recent accelerations in vaping activity among adolescents. This activity has been associated with both e-cigarettes containing nicotine as well as the vaping of cannabis products, further supporting the notion that the effects of each substance as well as their interactions should be a focus of continued study (Chadi, Hadland, & Harris, Reference Chadi, Hadland and Harris2019).
While we did not use functional magnetic resonance imaging to address directly the neural mechanisms that underpin the verbal learning and memory decrements in moderately frequent cannabis users, an abundance of evidence indicates that hippocampal functioning is disrupted by THC (Kruk-Slomka, Dzik, Budzynska, & Biala, Reference Kruk-Slomka, Dzik, Budzynska and Biala2017; Solowij & Battisti, Reference Solowij and Battisti2008). The endogenous cannabinoid system includes two broad receptor types (CB1 and CB2). CB1 receptors are distributed throughout the cortex, amygdala, hippocampus, striatum, and cerebellum. Preclinical work in adult animals indicates that CB1 agonists, including THC, impair learning acquisition as demonstrated through several hippocampally sensitive paradigms (Kruk-Slomka et al., Reference Kruk-Slomka, Dzik, Budzynska and Biala2017; Lisman & Grace, Reference Lisman and Grace2005; Lupica, Hu, Devinsky, & Hoffman, Reference Lupica, Hu, Devinsky and Hoffman2017). Long-term potentiation and depression, core substrates of learning, are disrupted by THC (Kruk-Slomka et al., Reference Kruk-Slomka, Dzik, Budzynska and Biala2017). Moreover, hippocampal circuits interact with the prefrontal cortex, which facilitates strategic aspects of free recall (Long, Oztekin, & Badre, Reference Long, Oztekin and Badre2010) as required by the RAVLT. Via connections between the hippocampal CA3 subfield and midbrain dopamine neurons, THC disruption of hippocampal circuitry alters incentive motivation, impacting reward salience (Loureiro, Renard, Zunder, & Laviolette, Reference Loureiro, Renard, Zunder and Laviolette2015; Lupica, Riegel, & Hoffman, Reference Lupica, Riegel and Hoffman2004).
Contrary to these predicted effects of cannabis use on motivational processes, cannabis use was not associated with IGT performance. This pattern was unexpected given that impulsive decision-making is increasingly recognized as a vulnerability factor for substance misuse (Lovell et al., Reference Lovell, Akhurst, Padgett, Garry and Matthews2020; Mallorquí-Bagué et al., Reference Mallorquí-Bagué, Fagundo, Jimenez-Murcia, de la Torre, Baños, Botella and Fernández-Aranda2016; Verdejo-Garcia et al., Reference Verdejo-Garcia, Benbrook, Funderburk, David, Cadet and Bolla2007), and several studies, including those from our own lab (Becker et al., Reference Becker, Collins and Luciana2014), suggest IGT-based decision-making impairments in young adults. IGT-based decision-making shows pronounced developmental change during adolescents (Almy et al., Reference Almy, Kuskowski, Malone, Myers and Luciana2018), and individual variation in performance was pronounced for participants in the current analysis. Despite shuffling the spatial layout of IGT deck contingencies over assessment waves, it may have been difficult for us to demonstrate longitudinal declines in performance given that the novelty of the task is reduced after a participant detects the presence of a set of contingencies to guide deck choices. This knowledge might facilitate decision-making on subsequent re-testing (Almy et al., Reference Almy, Kuskowski, Malone, Myers and Luciana2018). It may also be that motivated decision-making processes are affected primarily by clinical levels of substance abuse and dependence (Ernst et al., Reference Ernst, Grant, London, Contoreggi, Kimes and Spurgeon2003), rather than light-to-moderate use levels as reported in this study.
Limitations
Our sample size, while similar to other longitudinal studies of substance use initiators (Barthelemy et al., Reference Barthelemy, Richardson, Heeren, Chen, Liebschutz, Forman and Rose-Jacobs2019; Fried et al., Reference Fried, Watkinson and Gray2005), is modest, particularly as compared to recently initiated epidemiologically informed samples such as ABCD. Although the sample matched local demographics at study onset, there is limited representation of non-white and Hispanic ethnic and racial groups as well as individuals in low socioeconomic strata. While the results may not generalize to disadvantaged groups who may experience cumulative adversities over time (Green, Doherty, & Ensminger, Reference Green, Doherty and Ensminger2017), the observation of cognitive declines post-initiation in more advantaged individuals who are assumed to have greater levels of cognitive reserve (Cutuli, Ladrón de Guevara-Miranda, Castilla-Ortega, Santín, & Sampedro-Piquero, Reference Cutuli, Ladrón de Guevara-Miranda, Castilla-Ortega, Santín and Sampedro-Piquero2019) is significant. To mitigate the potential effects of sociodemographic factors that may impact substance misuse (Swendsen et al., Reference Swendsen, Conway, Degenhardt and Kessler2009), all analyses controlled for parental education. Larger studies (e.g., ABCD) will be better powered to disentangle the effects of socioeconomic variables on performance trajectories and will likely be better powered to include a control group of persistent non-users. In addition, while we assessed at each assessment wave whether individuals were actively engaged in substance use and how frequently they used, we did not undertake a more fine-grained assessment of quantity of cannabis used per occasion of use. Going forward, a more detailed assessment of cannabis use with regard to actual grams used and the potency of cannabis products used, especially as concentrates grow in popularity, will be increasingly important for future studies that attempt to draw conclusions regarding dose–response associations (Hindocha, Nordberg, & Tomko, Reference Hindocha, Norberg and Tomko2018). The same is true for nicotine.
Because this study was initially designed to address adolescent brain and behavioral development, we did not conduct formal drug screenings. We asked about the timing of recent substance use, and 24 hours of self-reported abstinence was required. Research assistants observed participants for signs of acute or residual intoxication. While we cannot exclude the possibility that participants engaged in substance use prior to arrival, cognitive testing was unlikely to have been impacted, since participants arrived for testing at 8 AM, typically with a parent, and cognitive testing began several hours after the start of each session. Nonetheless, external validation of recent abstinence remains a best practice (e.g., Infante et al., Reference Infante, Nguyen-Louie, Worley, Courtney, Coronado and Jacobus2020).
The study is notable for several strengths, including a multi-wave longitudinal assessment and the incorporation of well-validated measures of learning, memory, and decision-making, enhancing the rigor of the work. This is one of the first studies to demonstrate significant declines in verbal learning and memory pre-to-post-substance use-initiation in a non-clinical sample. It may be the first to incorporate a group of alcohol-only users as a control group. Findings affirm that verbal learning and memory should be a continued focus as we investigate the longitudinal impacts of adolescent and young adult cannabis use on the brain, behavior, and long-range outcomes.
FINANCIAL SUPPORT
The research presented in this report was supported by the National Institute on Drug Abuse and National Institute on Alcohol Abuse and Alcoholism of the National Institutes of Health under award numbers DA017943 and AA020033 awarded to M. Luciana. The content is solely the responsibility of the authors and does not necessarily represent the official views of the National Institutes of Health. HW was supported by a National Science Foundation Predoctoral Fellowship. NA was supported by a Multicultural Research Opportunity Program grant awarded by the University of Minnesota.
CONFLICTS OF INTEREST
The authors have no conflicts to disclose.