Policy Significance Statement
Digital advancements—inter alia the Internet of (every)Thing whereby all material and immaterial acts of the universe become a data point—make it possible that anything gets researched.Footnote 1 It implies that almost all research and science to be data intensive and interconnected with researchers producing and sharing increasing volumes of data. Although digital technology enables this transition, what makes this drive to the datafication of science and research irreversible is the data version of Metcalfe’s “law” of intangiblesFootnote 2 that is the value of a data set increases with the number of other data sets being made available; allowing more correlations and cross linking. Hence the importance of open data: a completely digitized but “closed” science is indeed only incrementally better than its analogue versions. Just like with the FAANG’sFootnote 3 of this world, will the benefit for science only exponentially increase if the data sets are openly available and reproducible. In the following, this paper identifies the potential benefits of data sharing and open science, supported by artificial intelligence tools and services, and dives into the challenges to make data open and findable, accessible, interoperable, and reusable (FAIR).
1. The Promise: Scientific Knowledge will be “Liquid”
Given this irreversibility of data driven and reproducible science and the role machines will play in that, it is foreseeable that the production of scientific knowledge will be more like a constant flow of updated data driven outputs, rather than a unique publication/article of some sort. Indeed, the future of scholarly publishing will be more based on the publication of data/insights with the article as a narrative.
For open data to be valuable, reproducibility is a sine qua non (King, Reference King2011; Piwowar et al., Reference Piwowar, Vision and Whitlock2011) and—equally important as most of the societal grand challenges require several sciences to work together—essential for interdisciplinarity.
This trend correlates with the already ongoing observed epistemic shift in the rationale of science: from demonstrating the absolute truth via a unique narrative (article or publication), to the best possible understanding what at that moment is needed to move forward in the production of knowledge to address problem “X” (de Regt, Reference de Regt2017).
Science in the 21st century will be thus be more “liquid,” enabled by open science and data practices and supported or even co-produced by artificial intelligence (AI) tools and services, and thus a continuous flow of knowledge produced and used by (mainly) machines and people. In this paradigm, an article will be the “atomic” entity and often the least important output of the knowledge stream and scholarship production. Publishing will offer in the first place a platform where all parts of the knowledge stream will be made available as such via peer review.
The new frontier in open science as well as where most of future revenue will be made, will be via value added data services (such as mining, intelligence, and networking) for people and machines. The use of AI is on the rise in society, but also on all aspects of research and science: what can be put in an algorithm will be put; the machines and deep learning add factor “X.”
AI services for scienceFootnote 4 are already being made along the research process: data discovery and analysis and knowledge extraction out of research artefacts are accelerated with the use of AI. AI technologies also help to maximize the efficiency of the publishing process and make peer-review more objectiveFootnote 5 (Table 1).
Table 1. Examples of AI services for science already being developed
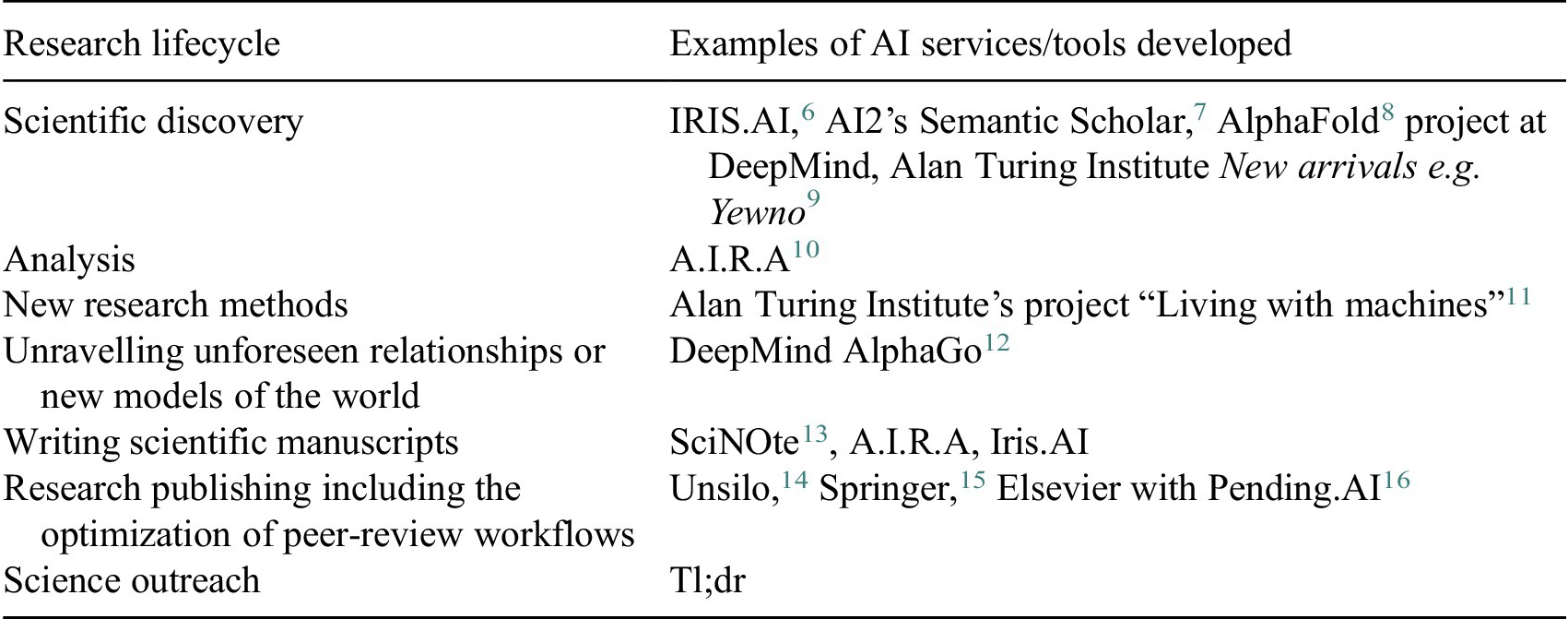
Abbreviation: AI, artificial intelligence.
Source: Authors’ research based on public sources, 2021.
Ultimately, actionable knowledge and translation of its benefits to society will be handled by humans in the “machine era” for decades to come. But as computers are indispensable research assistants, we need to make what we publish understandable to them.
The availability of data that are “FAIR by design” and shared Application Programming Interfaces (APIs) will allow new ways of collaboration between scientists and machines to make the best use of research digital objects of any kind. The more findable, accessible, interoperable, and reusable (FAIR) data resources will become available, the more it will be possible to use AI to extract and analyze new valuable information. The main challenge is to master the interoperability and quality of research data.
2. FAIR Data are Essential … But It will not Happen Sui Generis
The opportunity costs of having non-FAIR data are estimated at least €10.2bn every year in Europe alone (European Commission, 2019).Footnote 17 In addition, there are also a number of consequences from not having FAIR which could not be reliably estimated, such as impact on research quality, economic turnover, or machine readability of research data. Even so, FAIR principles as such seem however to be relatively unknown to the community (Digital Science, Reference Fane, Ayris, Hahnel, Hrynaszkiewicz, Baynes and Farell2019).
Moving to “FAIR-by-design” digital research outputs requires further efforts to develop, refine and adopt shared vocabularies, ontologies, metadata specifications, and standards, as well as increasing the supply and professionalization of data stewardship,Footnote 18 data repositories and data services in Europe and globally.
The European Open Science agenda contained the ambition to make FAIR data sharing the default for scientific research by 2020. To support as much as possible the proliferation of data that are FAIR, the emphasis has evolved from encouraging open access to research data for those projects funded by the EC (in Horizon 2020) to making research data open by default in Horizon Europe, following the principle “as open as possible, as closed as necessary” (Burgelman et al., Reference Burgelman, Pascu, Szkuta, Von Schomberg, Karalopoulos, Repanas and Schouppe2019; Budroni et al., Reference Budroni, Burgelman and Schouppe2019) taking into account the need to balance openness and protection of scientific information, commercialization and Intellectual Property Rights, privacy concerns, and security.
The move to open data means that researchers have to consider what data their research will produce and how the data will be made available. Practices with regard to data management, storage, and sharing differ widely across disciplines. A data management plan provides information on these issues, including metadata and standards, identifies suitable data repositories that will provide a unique and persistent identification of their data sets, curation and preservation and data sharing.
There are numerous legal issues in a research data environment, for example, regulation of copyright, ownership, and intellectual property for research dataFootnote 19; limitations to the sharing of research data that contains personal information by privacy requirements.Footnote 20 The “barriers to the free flow of data are caused by the legal uncertainty surrounding the emerging issues on “data ownership” or control, (re)usability and access to/transfer of data and liability arising from the use of data.”Footnote 21
The academic community is particularly concerned by these challenges (Aspesi et al., Reference Aspesi, Allen, Crow, Daugherty, Joseph, McArthur and Shockey2019), for example, ownership of data; (open) procurement of information tools and services, transparency of the algorithms, portability of the results, and sensitive data.
A broad range of changes (policy, cultural, and technical) would be needed to turn FAIR into reality in Europe (European Commission, 2018a). FAIR Digital Objects would be needed to enable discovery, citation, and reuse; data services to support FAIR; interoperability frameworks to incorporate research community practices; a distributed, federated infrastructure to unlock the potential of analysis and data integration; skills for data science and data stewardship; incentives for open science (metrics and indicators); and funding for FAIR to bring strong return on investment.
The use of trusted or certified data management environments like the European Open Science Cloud (EOSC)Footnote 22 (European Commission, 2018b) will be required for research data in some Horizon Europe work programs. Fostering the FAIR principles and data interoperability in the scientific communityFootnote 23 is an important landmark. These ongoing actions are paving the way but the long tail of science still needs further support and coordination at national and European level.
Publicly funded science should be a “commons.” The EOSC will enhance the possibilities for researchers to find, share and reuse publications, data, and software leading to new insights and innovations, higher research productivity and improved reproducibility in science. Europe is the first in the world to do that and EOSC the place of the science commons in Europe for data-intensive science and innovation (the “Web for FAIR data and servicesFootnote 24).
3. The Bottleneck of Open Data: Data Stewardship
Currently, researchers—and the machines they use to crawl the data universe—spend significant time in the process of transforming and mapping data, for lack of standards, services, or culture. An open research labor force of data scientists is needed, with expertise in analytics, code and workflows, statistics, machine learning, data mining, and data management.
The data steward, with strong domain knowledge and the ability to apply this know-how within organizations to create value, has become an invaluable asset to manage data better. But data roles continue to evolve. Whereas once it was expected that data scientists be responsible for every aspect of the data life cycle, data engineers will be needed to work alongside data scientists and analytics specialists that develop analytics tools to deliver that value and bring the data to life. Open Science specialists and data stewards would be needed to publish research in an open and FAIR way. Finally, a wider set of digital skillsFootnote 25 are needed in a wide range of data-related profiles, but also skills to manage software, code and orchestrate data-intensive workflows.
Today it seems that the major hurdle for fully deploying open data is the time needed to acquire skills and expertise and handle the data. The transition to open data will not go sui generis but researchers will understand the benefits—and funders will invest in skills (at the lab level, the headcount and the costs incurred should also be considered)—for faster and better science (Mons, Reference Mons2020) estimates the need for data stewards in Europe today to be around 500,000 (for every 20 people that generate data one data steward is needed).Footnote 26 If it is accepted that all science will very soon become data driven science than it follows a special effort is done to raise the level of data stewardship. And if even only 1% of the estimated 10 billion a year Europe invest in data infrastructures is allocated for that task, plenty of money will be available.
So money is not a problem, making the right priorities is.
How and what a business plan of open research data would look like and entail is a less straightforward issue. Not the least because up till now no one ever asked for a business plan for science either (and all intentions to quantify the impact of science have only given unsatisfactory responses).
Within the EOSC community this issue of what a business plan for EOSC could be has been discussed and it is suggested that several models could co-exist (European Commission, 2018b): a Direct Support Model, when an institute receives a grant from a funding entity to build/operate the resource and make it available to other grantees of the funding entity (however, the ability of certain researchers to access these resources may be restricted, i.e., nongrantees of the funding entity cannot access to the resources); a Cloud Coin Model—based on a certification program for commercial and noncommercial providers of scientifically useful services (“cloud coins”); or a Hybrid Model, i.e., combination of Direct Support Model and Cloud Coin Model.
Which of these will in the end make it, no one can know, because if so, that business plan would simply exist already.
4. The “Achilles Heel” of Open Data: Rewards and Incentives for Researchers to Make their Data Open
Scientific knowledge activity today is only incentivized by one metric: the impact factor of the author. This means that out of the whole activity of a scientist, only one product is rewarded: the article. Which means that all the work that is done before that and without which the article would never exist, is not taking into account into as a key performance indicator so to speak.
This single indicator incentive system worked for analogue science but is clearly not fit for a data driven science future where the production of open data set will be at least, if not more, important for the progress of science than the article.
It follows that the production of relevant open data sets should become a key indicator for measuring scientific performance.
Only if researchers are indeed rewarded for their data activity, will it make sense for them to invest time and resources into it.
First steps have been made, for example, by the EU, for example, Open Science Platform (European Commission, Reference Lawrence2020) and cOAlition S,Footnote 27 as well as around the world.Footnote 28 The momentum is now building up to launch a coalition of research actorsFootnote 29 to make a step change toward a science system that delivers higher quality and more impactful results. So, what could possibly go wrong?
The value of ORD but also the risks for ORD for 21st century science are unprecedented. The speed with which the scientific community was able to react to the CORONA challenge, based on open transfer of knowledge will most likely become the best user case for open data. However, minimal safeguards need to be put in place for open research data to stay open. The policy challenge is how to avoid misuse by public and private actors (Zuboff, Reference Zuboff2019) and dependability of all kind of providers (like in OA). ORD should be as open as possible as closed as needed and unintended use should not be allowed or only with consent.
AI that is “accountable to society” (Smith, Reference Smith2019) is one of the top technology policy issues that will shape the science of the 21st century. Machines must remain accountable to people and people who create AI technology must remain accountable to society as a whole Face recognition technology and its use by governments and law enforcement is controversial.Footnote 30 Private technology companies alone cannot be trusted to safely manage the data they collect.Footnote 31
“We cannot just complain about how tech is transforming our world; we need to invent the transformation.”Footnote 32 Solutions should be global in natureFootnote 33: smart deals could be signed with publishers and platform providersFootnote 34; compliance could be embedded in the design of the ORD services and standards (GDPR compliant) or the architecture of the sharing and access system; public data banks could be created hosting key data sets and trusted third parties could be envisaged to outsource independent data handling (like the credit card system). Eventually, people could gain power with the platforms through “mediators of individual data.”Footnote 35
But fundamentally the world should accept that open science data is a commons: to the benefit of all.
Funding Statement
This work received no specific grant from any funding agency, commercial or not-for-profit sectors.
Competing Interests
The authors whose names are listed immediately below certify that they have NO affiliations with or involvement in any organization or entity with any financial interest (such as honoraria; educational grants; participation in speakers’ bureaus; membership, employment, consultancies, stock ownership, or other equity interest; and expert testimony or patent licensing arrangements), or nonfinancial interest (such as personal or professional relationships, affiliations, knowledge, or beliefs) in the subject matter or materials discussed in this manuscript.
Author Contributions
All authors contributed equally to the article.
Data Availability Statement
Data availability is not applicable to this article as no new data were created or analyzed in this study.
Comments
No Comments have been published for this article.