Introduction
Interpreters translate from a source language (SL) into a target language (TL). To do this, they need to first arrive at a semantic message by decoding SL lexical and syntactic information and then find corresponding TL lexical expressions and syntactic structures to express the semantic message in the TL. Interpreters often have to execute these tasks under time pressure (in either simultaneous or consecutive interpreting). It has been proposed that interpreters actively anticipate or predict upcoming linguistic information (e.g., words, syntax) in both the SL and the TL (Amos & Pickering, Reference Amos and Pickering2020; Chernov, Reference Chernov, Lambert and Moser-Mercer1994; Moser, Reference Moser, Gerver and Sinaiko1978). In this paper, we ask whether interpreters make more predictions in SL comprehension than in regular language comprehension in order to maximize timely delivery of interpreting, and if that's the case, whether such a predictive advantage in SL comprehension is constrained by cognitive resources.
Linguistic prediction in regular language comprehension
Linguistic prediction refers to the pre-activation of representations at different linguistic (e.g., syntactic, semantic, lexical, or even phonological/orthographic) levels (Pickering & Garrod, Reference Pickering and Garrod2007, Reference Pickering and Garrod2013). At the semantic level, comprehenders anticipate upcoming referents. For example, upon hearing the verb eat in the sentence The boy will eat the cake, they anticipate something edible (e.g., a cake) to be mentioned next (Altmann & Kamide, Reference Altmann and Kamide1999; Kamide, Altmann & Haywood, Reference Kamide, Altmann and Haywood2003). People also predict the syntactic role of an upcoming word/phrase (Arai, van Gompel & Scheepers, Reference Arai, van Gompel and Scheepers2007; Chen, Tan, Deng & Xu, Reference Chen, Tan, Deng and Xu2010; Staub & Clifton, Reference Staub and Clifton2006). For instance, Staub and Clifton (Reference Staub and Clifton2006) showed that, in reading a sentence like Either Linda bought the red car or her husband leased the green one, the fact that either headed a clause (instead of a prepositional object) led participants to expect or to also head a clause and accordingly to predict her husband as the subject of an upcoming clause (rather than treating it as part of the red car or her husband).
Importantly for the current research, people predict upcoming lexico-semantics in a sentence. For example, people are faster at reading, or even more likely to skip, a word when it is contextually predictable (e.g., voice in I could tell he was mad by the tone of his voice) than when it is unpredictable (Brothers, Swaab & Traxler, Reference Brothers, Swaab and Traxler2015; Rayner, Slattery, Drieghe & Liversedge, Reference Rayner, Slattery, Drieghe and Liversedge2011; Traxler & Foss, Reference Traxler and Foss2000). While it is possible that such lexico-semantic facilitation results from ease of integration (e.g., it is easier to integrate a predicted than unpredicted word into the context), there is evidence that at least some lexico-semantic information of the predicted word is pre-activated by the context. Kwon, Sturt, and Liu (Reference Kwon, Sturt and Liu2017) manipulated the relative clause Zhang1 Yi4mo2 zhi3dao3 de (lit., “Zhang Yimou directed RELATIVIZER …”) to be followed by a noun phrase consisting of a demonstrative, a classifier, and a noun that was predicted (zhe4 bu4 dian4ying3, lit., “this CLASSIFIER movie”) or not (zhe4 chang3 yan3chu1, lit, “this CLASSIFIER show”). Critically, the predicted noun and the non-predicted noun require distinct classifiers (i.e., bu4 vs. chang3); therefore, if people predict the lexico-semantics of dian4ying3, they should have difficulty in comprehending a classifier that does not match the predicted noun (e.g., chang3 compared to bu4). Indeed, Kwon et al. showed that people exhibited N400 (an ERP component reflecting difficulty in semantic interpretation) upon reading a classifier that mismatched the predicted noun, suggesting that participants pre-activated lexico-semantic information of dian4ying3 (hence the classifier-noun mismatch effect). In addition, people may even make fine-grained predictions about the phonological form of an upcoming word (DeLong, Urbach & Kutas, Reference DeLong, Urbach and Kutas2005; Ito, Corley, Pickering, Martin & Nieuwland, Reference Ito, Corley, Pickering, Martin and Nieuwland2016). For instance, the sentential context The student is going to the library to borrow a … implies book as an upcoming word. Ito et al. (Reference Ito, Corley, Pickering, Martin and Nieuwland2016) showed that a word related in form to the predicted word (e.g., hook) elicited a reduced N400 effect compared to a word that is unrelated in form to the predicted word (e.g., sofa), suggesting fine-grained prediction of the word form book, which in turn also activated the word hook.
Lexico-semantic prediction is also observed in second language (L2) comprehension. In a visual world paradigm study, Ito, Corley and Pickering (Reference Ito, Corley and Pickering2018) had native and L2 speakers of English listen to a sentence such as The lady will fold the scarf while viewing an array of objects (the target object scarf together with a guitar, a piano, and a pair of high heels); they then clicked on the mentioned object (i.e., the scarf). Both native and L2 speakers predictively looked at a foldable object (e.g., a scarf) before the target noun was mentioned and did so to the same extent, suggesting that they were equally good at making use of prior context to anticipate upcoming semantic information in a sentence.
Foucart, Martin, Moreno and Costa (Reference Foucart, Martin, Moreno and Costa2014) further showed that L2 speakers can predict lexico-syntactic properties of upcoming words. They manipulated, in Spanish, whether a word was contextually predicted or not. For example, a context (translated from Spanish) like The pirate had the secret map, but he never found … led to the prediction of the word treasure (which requires a masculine pre-noun article in Spanish) but not cave (which requires a feminine pre-noun article). They showed that native speakers of Spanish showed an N400 effect when the article mismatched the predicted noun in gender; importantly, a similar N400 effect was also observed in L2 speakers of Spanish, suggesting the prediction of lexico-syntactic properties in L2 comprehension.
However, there is also evidence that some aspects of linguistic prediction are more limited in L2 compared to native language comprehension. Ito, Pickering and Corley (Reference Ito, Corley and Pickering2018) had native English-speaking and L2 English-speaking participants listen to a sentence with a predictable target word (e.g., cloud in The tourists expected rain when the sun went behind the cloud) while viewing a display of objects including the target object (a cloud) and an object whose name was phonologically similar to cloud (a clown). They showed that, while native speakers looked more to both the cloud and the clown before the target word cloud was heard, L2 speakers predictively looked only to the cloud but not the clown. This finding suggests that L2 speakers may not predict word forms to the same extent as native speakers do. Chun and Kaan (Reference Chun and Kaan2019) also showed that, when comprehending complex structures (e.g., relative clauses), L2 speakers made semantic predictions, but in a delayed manner compared to native speakers.
In summary, people predict upcoming linguistic information in language comprehension; importantly, L2 sentence comprehension also involves linguistic prediction, especially of lexico-semantic and lexico-syntactic information.
Mechanisms underlying linguistic prediction
Pickering and Gambi (Reference Pickering and Gambi2018) proposed that, while prediction may occur as a result of spreading activation between related representations (e.g., book can be activated via semantic association with student and library in The student is going to the library to borrow …), a more effective mechanism at work is prediction-by-production. That is, comprehenders use their production system to covertly imitate an unfolding sentence they are comprehending, and thereby predict upcoming information in the same way that they would complete the sentence themselves (Dell & Chang, Reference Dell and Chang2014; Pickering & Garrod, Reference Pickering and Garrod2007, Reference Pickering and Garrod2013; Pickering & Gambi, Reference Pickering and Gambi2018). For instance, upon hearing The student is going to the library to borrow …, listeners covertly imitate the production of the sentence fragment and are likely to continue the sentence with a book, even before book is heard. Pickering and Gambi (Reference Pickering and Gambi2018) regarded prediction-by-production as the central mechanism for prediction in language comprehension.
Indeed, prediction-by-production has gained much empirical support. Martin, Branzi and Bar (Reference Martin, Branzi and Bar2018) had people read Spanish sentences which ended with an article and a contextually predicted noun. If the article mismatched in gender with the predicted noun, it generated an enhanced N400 when readers simultaneously tapped their tongue or listened to syllables, but not when they additionally uttered syllables, suggesting that linguistic prediction is limited when the production system is engaged in another task (e.g., syllable production). Moreover, Rommers, Dell and Benjamin (Reference Rommers, Dell and Benjamin2020) had participants read, aloud or silently, sentences where the final word was either contextually predictable or unpredictable. When subsequently asked whether they had seen a word before, participants showed a production effect, with better memory for words that had been read aloud than silently. More importantly, the production effect was smaller for predictable than unpredictable words, suggesting that participants used covert production to predict a word. Further supporting the hypothesis that predicting a word involves covert production, they showed that participants were less accurate at recalling whether they had read a word aloud or silently if the word was predictable than unpredictable in the sentence. These results highlight the critical role of covert language production in linguistic prediction.
It has also been suggested that making prediction via production requires time and cognitive resources and thus prediction-by-production may be reduced (if not impossible) when there is not enough time or cognitive resources (Pickering & Gambi, Reference Pickering and Gambi2018). Older adults, for instance, are shown to engage less linguistic prediction compared to younger adults, presumably due to their limited cognitive resources (DeLong, Groppe, Urbach & Kutas, Reference DeLong, Groppe, Urbach and Kutas2012). People also show less or delayed prediction if they have a smaller working memory span (Huettig & Janse, Reference Huettig and Janse2016) or when they are under a cognitive load (Ito, Corley, et al., Reference Ito, Corley and Pickering2018). These results indicate that prediction-by-production is constrained by the availability of cognitive resources, and more importantly, linguistic prediction may be limited in a cognitively demanding linguistic task such as interpreting (Amos & Pickering, Reference Amos and Pickering2020).
Linguistic prediction in interpreting
Many theoretical models of interpreting have highlighted the importance of anticipating upcoming SL (i.e., source language) and TL (i.e., target language) during interpreting. For instance, Moser's (Reference Moser, Gerver and Sinaiko1978) interpreting processing model regards prediction as a vital strategy that helps to conserve processing capacity and facilitate SL comprehension and TL delivery. Chernov's (Reference Chernov, Lambert and Moser-Mercer1994) probability prognosis model stipulates that interpreters probabilistically predict SL at different linguistic levels, which facilitates TL delivery. Interpreting practitioners also often view prediction as an important interpreting skill and even a constituent of interpreting competence (Gile, Reference Gile2009; Setton, Reference Setton2005). More recently, Amos and Pickering (Reference Amos and Pickering2020) proposed that simultaneous interpreting involves SL prediction via prediction-by-production. That is, interpreters covertly simulate what the speaker is likely to say next (e.g., predicting book upon hearing The student is going to the library to borrow…); such prediction then facilitates pre-activation of TL representations (e.g., the translation equivalent of book), leading to better fluency in simultaneous interpreting.
There have been observations in support of predictive TL production in interpreting. Corpus analyses of interpreters’ output showed that professional interpreters often anticipatorily produce translation equivalents of upcoming SL words, especially when word order is different between SL and TL, a finding that is often viewed as evidence that interpreters adopt prediction in interpreting (e.g., Jörg, Reference Jörg, Snell-Hornby, Jettmarová and Kaindl1997; van Besien, Reference van Besien1999; Wilss, Reference Wilss, Gerver and Sinaiko1978). These observations were corroborated by an experimental study by Hodzik and Williams (Reference Hodzik and Williams2017), who had English–German simultaneous interpreters and non-interpreters translate the German verb-final sentences into English. They found that both groups occasionally translated the final verb in advance before it was actually heard. Note that these “premature” productions could not be speech errors, as they were translations of German verbs that were to appear later in the SL; instead, these results suggest that interpreting (by interpreters or novice people) involves anticipation of SL content and advance preparation of the TL materials.
As language comprehension has been shown to engage linguistic prediction, we should expect SL comprehension (which is a special form of language comprehension; see Amos, Reference Amos2020, for discussion) in interpreting to also make use of the predictive mechanism. What is less clear is whether SL comprehension involves more or less prediction of upcoming linguistic content compared to regular language comprehension. On the one hand, there is evidence that linguistic prediction is cognitively costly such that prediction is reduced or delayed under higher cognitive load (e.g., Chun & Kaan, Reference Chun and Kaan2019; Ito, Corley et al., Reference Ito, Corley and Pickering2018). Given that interpreting is a cognitively demanding task (e.g., Hyönä, Tommola & Alaja, Reference Hyönä, Tommola and Alaja1995), interpreters may have limited cognitive resources available to fuel linguistic prediction; therefore, one should expect interpreters to engage linguistic prediction to a lesser extent in SL comprehension in interpreting than in regular language comprehension. On the other hand, it is possible that interpreters may have an enhanced mechanism for linguistic prediction in order to facilitate delivery of interpreting (Chernov, Reference Chernov, Lambert and Moser-Mercer1994; Moser, Reference Moser, Gerver and Sinaiko1978) such that they predict more in SL comprehension than in regular language comprehension. Indeed, there is some tentative evidence that (professional) interpreters may have seasoned predictive machinery that enables them to engage in prediction to a greater extent than non-interpreters even in daily language communication (Fan, Reference Fan2013; Lozano-Argüelles, Sagarra & Casillas, Reference Lozano-Argüelles, Sagarra and Casillas2020; Lozano-Argüelles & Sagarra, Reference Lozano-Argüelles and Sagarra2021).
The current study thus addresses whether interpreters make more predictions in SL comprehension than in regular comprehension and whether the predictive advantage in SL comprehension in interpreting (if any) is constrained by cognitive resources. In particular, we focused on consecutive interpreting, where, unlike in simultaneous interpreting, the interpreter comprehends a chunk of SL before s/he starts interpreting (when SL is paused). Thus, consecutive interpreting offers a good test case for us to examine whether interpreters are predictive in SL comprehension, in addition to being predictive in TL production (e.g., Hodzik & Williams, Reference Hodzik and Williams2017).
To address the above question, we need an experimental task that taps into processes of SL comprehension and a control task that taps into the processes of regular language comprehension but otherwise resembles as much as possible SL comprehension in the experimental task. In particular, SL comprehension in interpreting involves decoding a SL utterance or text and keeping that information in working memory for guiding subsequent production in the TL. It is thus more complicated than typical comprehension tasks used in psycholinguistic studies (e.g., reading to later answer a yes/no question), where participants do not have to actively maintain the linguistic information, as they would do if they were to read to later interpret (as in our experiments).
Thus, we adopted the reading to interpret/recall method previously devised by Macizo and Bajo (Reference Macizo and Bajo2006) as our experimental paradigm to examine possible differences in processing between SL comprehension in consecutive interpreting (i.e., reading to later interpret) and regular language comprehension (reading to later recall). In reading to interpret, participants read a sentence (in a self-paced, word-by-word manner) and then, at the end of the sentence, interpret it into a target language. Note that while reading to interpret differs from typical consecutive interpreting in having participants read instead of listening to the SL, it actually resembles typical consecutive interpreting in all core cognitive processes, including decoding input (SL) input, keeping decoded information and preparing TL in working memory, and finally preparing a TL utterance on the basis of memorized information. In fact, sight interpreting (Agrifoglio, Reference Agrifoglio2004; Lambert, Reference Lambert2004) is a form of interpreting involving written SL input and spoken TL output. Recent research has also begun to use text reading as a method to tap into cognitive processes underlying interpreting (Seeber, Keller & Hervais-Adelman, Reference Seeber, Keller and Hervais-Adelman2020). In addition, from a psycholinguistic perspective, the reading-to-interpret/recall paradigm enables researchers to examine processing times on a word-by-word basis, thus allowing for the examination of SL prediction.
The paradigm also affords an ideal control task. In reading to recall, participants read a sentence and they are to later recall the sentence (in either a verbatim or paraphrasing manner); thus, they will need to decode the input sentence and also keep the linguistic information in working memory in order to later guide language production (i.e., recall). Note that there is much evidence that people do not keep a verbatim memory of a sentence if they read it to later recall; instead, they extract the meaning of the sentence and regenerate a sentence based on the memorized meaning (e.g., Lombardi & Potter, Reference Lombardi and Potter1992; Potter & Lombardi, Reference Potter and Lombardi1990). Therefore, there is a close parallel between reading to later recall and reading to later interpret.
Importantly, the interpreting and recall tasks in the paradigm differ in one critical aspect that we set out to examine (as previously researchers did too): reading-to-interpret, but not reading-to-recall, involves the parallel processing of TL representations while the SL is being comprehended (Macizo & Bajo, Reference Macizo and Bajo2006), especially when interpreting from a second language into a native language, as our participants did (Dong & Lin, Reference Dong and Lin2013). This feature of the paradigm thus enables us to examine whether the parallel processing of TL representations additionally facilitates linguistic prediction of the input (SL) sentence content.
Below, we report two experiments (Experiments 1a/b and 2) using the reading-to-interpret/recall paradigm to investigate whether SL comprehension in interpreting (as reflected in reading to interpret) involves more or less lexico-semantic prediction than regular language comprehension (as reflected in reading to recall). We manipulated a critical word to be predictable or unpredictable from earlier sentential context (e.g., Without the sunglasses/hat, the sun will hurt your eyes on the beach). We measured the reading times of the critical word and its following regions. If people predict the lexico-semantics of the critical word, we should expect critical the word and/or following words to be read faster when the critical word is predictable than when it is unpredictable (a prediction effect). Thus, we compared the prediction effect between reading to interpret and reading to recall. In Experiment 1a and 1b, Chinese–English bilinguals with interpreting training/experienceFootnote 1 read a sentence to later interpret or to later recall. In Experiment 2, we additionally manipulated cognitive load by having participants keep a low load (one sentence) or high load (two sentences) of linguistic content in memory to later recall/interpret; we tested whether cognitive load affects prediction in SL comprehension. Both experiments were conducted online as a result of the COVID-19 pandemic.
Experiment 1a and 1b
In Experiment 1a and 1b, we examined whether bilinguals with interpreting training/experience make more lexico-semantic predictions when they read a sentence to later interpret than to later recall. In particular, we manipulated a critical word to be predictable or unpredictable in a sentence (e.g., Without the sunglasses/hat, the sun will hurt your eyes on the beach, where the critical word is eyes). We expect interpreters to read the critical word and/or following words faster when the critical word is predictable than when it is unpredictable (a prediction effect). More importantly, if interpreters make more predictions in SL comprehension than in regular language comprehension, we should expect a larger prediction effect in reading to interpret than in reading to recall; we refer to larger prediction effect as the predictive advantage in SL comprehension in interpreting (as compared to regular language comprehension). Experiment 1a and 1b had exactly the same design. We conducted Experiment 1a first; then, in order to be sure that we could trust the findings from the online experiment, we conducted a replication (Experiment 1b), using more and improved target items (see Design and materials below).
Method
Participants
Experiment 1a used 52 Chinese–English bilinguals with prior interpreting training and/or experience (45 females; mean age = 23.0, SD = 1.6, range = 21–30) and Experiment 1b used another 50 bilinguals from the same population (47 females; mean age = 23.0, SD = 2.7, range = 19–31). These participants were all native speakers of Mandarin Chinese, who were undergraduate or postgraduate students majoring in English at Chinese universities. They reported to have had interpreting training courses in universities ranging from one month to more than 2 years before the experiment (with the interpreting courses covering 1.5-6 hours each week and mostly focusing on consecutive interpreting between Chinese and English) and/or professional experience in interpreting between Chinese and English. Their average age of acquisition for English was 8.8 years (SD = 2.3, range: 4–14) in Experiment 1a and 8.7 (SD = 2.4, range: 4–13) in Experiment 1b, with English proficiency ranging from intermediate to high level (corresponding to B1 to C2 in the Common European Framework) as assessed by a Quick Placement Test (UCLES, 2001). All of them reported normal hearing, normal (or corrected-to-normal) vision, and no language disorders. Participants received 60 RMB as a reward after completing the experiment. Each participant in this study only took part in one experiment/pretest.
Design and materials
Both experiments adopted a design of 2 (predictability: predictable vs. unpredictable) x 2 (task: reading to recall vs. reading to interpret); both variables were manipulated within participants and items, with a block design for the second variable. To norm experimental materials for Experiment 1a, we first constructed 90 pairs of semantically plausible sentences where a critical word (e.g., eyes) is predictable or unpredictable on the basis of the preceding sentential context (e.g., Without the sunglasses/hat, the sun will hurt your eyes on the beach). To maximize the likelihood that a critical word is predictable from the preceding sentential context, we recruited another 99 participants (from the same population as those in the main experiments) to complete a cloze test on the online survey platform Qualtrics (https://www.qualtrics.com). The sentence context prior to the critical word (from either predictable or unpredictable version; e.g., Without the sunglasses, the sun will hurt your ___) was presented in writing and participants were instructed to supply the missing part by typing into a text box. We calculated the cloze probability (probability of a critical word supplied in the sentence completion among valid responses) for each sentence context. Eventually, for Experiment 1a, we selected 48 sentences of which the context in the predictable condition yielded a high cloze probability for the critical word (M = 87.8%, SD = 9.0%, range = 61.1%-100%) while the context in the unpredictable condition yielded a much lower cloze probability (M = 12.5%, SD = 11.6%, range = 0%-42.1%). The critical word had an average length of 5 letters and an average frequency of 126.2 per million in SUBTLEX-US (Brysbaert & New, Reference Brysbaert and New2009). There were also 64 filler sentences similar in length to the target sentences (for details of experimental materials, see osf.io/yrxh5/).
Experiment 1b improved over Experiment 1a in terms of the target sentences. In particular, to increase the number of items, we constructed another 42 pairs of sentences and conducted another cloze test among 55 participants (again from the same population as those in the main experiments). With materials from this test, we increased the target sentences to 72 (we also excluded two target sentences from Experiment 1a that had identical morphemes in the prior sentential context and target word; e.g., air passengers and airport). For this new set of target sentences in Experiment 1b, the cloze probability in the predictable condition (M = 85.7%, SD = 10.0%, range = 61.1% – 100%) was substantially higher than in the unpredictable condition (M = 12.0%, SD = 11.6%, range = 0% – 42.1%). The critical word had a mean length of 6.1 letters and a mean frequency of 158.9 per million in SUBTLEX-US. There were also 24 filler sentences (this number was reduced from 64 in Experiment 1a in order to avoid a lengthy online experiment). Other design details of the materials in Experiment 1b were identical to those in Experiment 1a.
In both Experiment 1a and 1b, we kept the predictable and unpredictable versions of a target sentence as close as possible by manipulating no more than five words to make an unpredictable version out of the predictable version (e.g., in Without the sunglasses/hat, the sun will hurt your eyes on the beach, there is a difference of one word between the two versions: sunglasses vs. hat); in addition, change of words occurred at least three words prior to the critical word to minimize potential spill-over effects from these changed words to the critical word. In other words, the two versions had exactly the same number of words (ranging from 10 to 20), had the same position in the sentence for the critical word (ranging from the 6th to the 17th among the target items), were identical from at least two words prior to the critical word and all the way to the end (e.g., … the sun will hurt your eyes on the beach), and were identical in syntactic structure. In order to allow for the detection of any potential spill-over effect from the critical word, the critical word was always followed by at least two words before the end of the sentence.
Thus, Experiment 1a had 48 target sentences and 72 fillers and Experiment 1b had 72 target sentences and 24 fillers. The sentences were further paired with two tasks (reading to recall vs. reading to interpret). By arranging the items in a Latin-square design, four experimental lists were created such that each participant only saw each version of a target sentence once in the experiment.
Procedure
The experiment was run online on Gorilla (http://www.gorilla.sc), an experimental platform that allowed the collection of reaction time data (e.g., in self-paced reading; Anwyl-Irvine, Massonnié, Flitton, Kirkham & Evershed, Reference Anwyl-Irvine, Massonnié, Flitton, Kirkham and Evershed2020). Following Macizo and Bajo (Reference Macizo and Bajo2006), we had participants read an English sentence in a word-by-word self-paced manner and then performed an interpreting or recall task. At the beginning of each block, participants were instructed regarding whether to interpret or to recall a sentence. A trial began with a fixation cross followed by a sentence, presented word by word at the centre of the webpage in a self-paced manner at a press of the spacebar. The interval between consecutive key presses was recorded as the reading time for each word. Participants were instructed not to take notes during the reading. Following the end of the sentence was a task cue (“Translate” or “Recall”). In the interpreting task, participants interpreted the English sentence they had just read into Mandarin Chinese; in the recall task, participants verbally recalled the sentence (in English). At the end of interpreting/recalling, participants pressed the spacebar to proceed to the next trial and the verbal response was digitally recorded.
An experiment consisted of four blocks of 12 target sentences and 16 filler sentences in Experiment 1a and four blocks of 18 target sentences and 6 filler sentences in Experiment 1b (with a total of 112 and 96 sentences in total respectively in the two experiments). Two blocks were assigned to the interpreting task and the other two to the recall task, with the order of the tasks counterbalanced across participants (i.e., half of them did the two interpreting blocks first and the other half the two recall blocks first). Each task was preceded by 3 (in Experiment 1a) or 4 (in Experiment 1b) practice sentences and the sentences in a block were presented in a random order. Participants were allowed to take breaks between blocks, though they were required to finish the experiment within 2.5 hours. On average, the two experiments respectively lasted for about 70 and 50 minutes (including breaks).
Results
Recorded interpreting and recall outputs were manually checked by one of the authors and a research assistant. In particular, we examined whether a recall or interpreting output was accurate regarding the manipulated sentential context (e.g., without the sunglasses/hat) and the critical word (e.g., eyes). An error in one of these two key regions was treated as a critical content error and the trial was removed from further analyses (206 and 289 trials, or 8.25% and 8.02% of all target trials respectively in Experiment 1a and 1b). We also removed 36 and 40 trials (1.44% and 1.11% of all the target trials) respectively in the two experiments where there was a task error (e.g., recalling the original sentence when the task instruction was to interpret) and removed 34 and 66 trials (1.36% and 1.83% of all the target trials) where partial or no verbal output was recorded due to technical failure or carelessness of participants. One participant in each experiment was excluded altogether from further analyses for having more than 40% of the target trials removed. The mean error rates by task and predictability in Experiment 1a and 1b were summarized in Table A1 in the appendix.
For the remaining trials (from 51 participants in Experiment 1a and 49 participants in Experiment 1b), following prior practice in self-paced reading research (e.g., Futrell, Gibson, Tily, Blank, Vishnevetsky, Piantadosi & Fedorenko, Reference Futrell, Gibson, Tily, Blank, Vishnevetsky, Piantadosi and Fedorenko2021; Luke & Christianson, Reference Luke and Christianson2013), we first excluded RTs less than 100 ms as outliers, for these brief RTs were likely due to accidental key presses without participants actually reading the word. Then, we further excluded RTs that were more than 3 SDs beyond the mean of each experimental condition for each region in the two experiments (159 and 308 RTs, 1.27% and 1.71% of all the target RTs respectively in Experiment 1a and 1b). The remaining RTs were log-transformed to reduce the right-tail skewness (Baayen, Reference Baayen2008). We conducted linear mixed-effect (LME) regression analyses on the log-transformed RTs, using the lme4 package (Bates, Mächler, Bolker & Walker, Reference Bates, Mächler, Bolker and Walker2015) together with the lmerTest package (Kuznetsova, Brockhof & Christensen, Reference Kuznetsova, Brockhof and Christensen2017) for estimating p-values via the Satterthwaite approximation method. Since the predictable and unpredictable versions were identical at the critical word, and identical minimally two words preceding and after the critical word, we chose to run separate analyses for only these five reading regions of the target sentences, including the second (C-2) and the first (C-1) word preceding the critical word, the critical word (C), the first (C+1) and the second (C+2) word following the critical word (see Fig. 1). Both predictors were contrast-coded (task: reading to recall = -0.5, reading to interpret = 0.5; predictability: predictable = -0.5, unpredictable = 0.5). The fixed effects included the main effects of both predictors and their interaction. Following a recent proposal (Bates, Kliegl, Vasishth & Baayen, Reference Bates, Kliegl, Vasishth and Baayen2015; Matuschek, Kliegl, Vasishth, Baayen & Bates, Reference Matuschek, Kliegl, Vasishth, Baayen and Bates2017), we selected the maximal random effect structure justified by the data, using a forward model comparison approach and using the alpha level of .2 instead of .05 to guard against anti-conservativity. To further pinpoint the locus of a significant interaction effect, the data was also fitted with a model by recoding the categorical predictors in terms of dummy coding, and pairwise comparisons between different conditions were conducted via the emmeans package (Lenth, Singmann, Love, Buerkner & Herve, Reference Lenth, Singmann, Love, Buerkner and Herve2018). All the data analyses were performed in R (R Core Team, 2020). The experimental data and analytical scripts are available in osf.io/yrxh5/.

Fig. 1. Log RTs for the critical word and surrounding words in self-paced reading in Experiment 1a (left panel) and Experiment 1b (right panel). Int = reading to interpret; rec = reading to recall; pred: = predictable; unpred = unpredictable.
As shown in Table 1 and Table 2, our analysis revealed a significant effect of predictability (i.e., a prediction effect) across several regions in both experiments (for all the five regions in Experiment 1a and for the C-1, C and C+2 region in Experiment 1b), with shorter RTs in these regions in the predicable than unpredictable condition. Moreover, we also observed a significant or marginally significant effect of task in some regions in both experiments (for the C-1, C+1 and C+2 region in Experiment 1a and for the C-1 and C+1 region in Experiment 1b), with slower RTs in reading to recall than reading to interpret.
Table 1. LME results for Experiment 1a. Regression coefficients (βs) and their SEs are standardized; the intercept stands for the mean across all conditions. Significant effects in bold. Pred = predictability.

Note. The formula of the final LME models for the five reading regions were as follows:
C-2: lmer(logRT~Task*Pred+(1+Task|Subject)+(1+Task|Item);
C-1: lmer(logRT~Task*Pred+(1+Task|Subject)+(1|Item);
C: lmer(logRT~ Task*Pred+(1+Task+Pred|Subject)+(1+Task+Pred|Item);
C+1: lmer(logRT~ Task*Pred+(1+Task|Subject)+(1|Item);
C+2: lmer(logRT~ Task*Pred+(1+Task+Pred|Subject)+(1|Item).
Table 2. LME results for Experiment 1b. Regression coefficients (βs) and their SEs are standardized; the intercept stands for the mean across all conditions. Significant effects in bold. Pred = predictability.

Note. The formula of the final LME models for the five reading regions were as follows:
C-2: lmer(logRT~Task*Pred+(1+Task|Subject)+(1+Pred+Task:Pred|Item);
C-1: lmer(logRT~Task*Pred+(1+Task|Subject)+(1+Task:Pred|Item);
C: lmer(logRT~ Task*Pred+(1+Task+Pred|Subject)+(1+Pred+Task+Task: Pred|Item);
C+1: lmer(logRT~ Task*Pred+(1+Task|Subject)+(1+Pred|Item);
C+2: lmer(logRT~ Task*Pred+(1+Task|Subject)+(1+Pred|Item).
Critically, our analysis found a significant interaction of task and predictability in both experiments. In Experiment 1a, the significant interaction effect emerged in the C (the critical word) and C+1 region. For the C region, RTs in the predictable condition were significantly faster than those in the unpredictable condition in both tasks (reading to interpret: β = -0.177, SE = 0.03, t = -5.13, df = 95.1, p <. 001; reading to recall: β = -0.088, SE = 0.03, t =−2.59, df = 90.5, p =.011). However, the magnitude of the prediction effect (i.e., difference in RTs between predictable and unpredictable condition) was much larger in reading to interpret (284 ms) than reading to recall (75 ms). For the C+ 1 region, further analyses showed a significant predictability effect in reading to interpret (β = -0.079, SE = 0.02, t = -3.38, df = 2024, p =.001) but not in reading to recall (β = -0.013, SE = 0.02, t = -0.56, df = 2022, p =. 578).
In Experiment 1b, the significant interaction effect also emerged in the C+1 region. As in Experiment 1a, a significant effect of predictability was found in reading to interpret (β = -0.053, SE = 0.02, t = -2.38, df = 191, p =.019) but not in reading to recall (β = 0.010, SE = 0.02, t = 0.46, df = 197, p =.648). Thus, the results of both experiments found a stronger predictability effect when people read a sentence to later interpret it than to recall it.
Discussion
In both Experiment 1a and 1b, bilinguals with interpreting training/experience read an English sentence word by word in a self-paced manner; they later either interpreted it into Chinese or recalled it in English. Participants were quicker at reading the critical word and/or the following word(s), which is consistent with earlier findings that people anticipate upcoming linguistic materials (e.g., Brothers et al., Reference Brothers, Swaab and Traxler2015; Kwon et al., Reference Kwon, Sturt and Liu2017), and also do so even in L2 reading (e.g., Foucart et al., Reference Foucart, Martin, Moreno and Costa2014; Ito, Corley, et al., Reference Ito, Corley and Pickering2018; Ito, Pickering, et al., Reference Ito, Pickering and Corley2018). Indeed, participants might even read words before the critical word faster in the predictable than unpredictable condition (see main effects of predictability in C-2 and C-1 region in Experiment 1a and in C-1 region in Experiment 1b). As the critical word was often a noun preceded by a determiner and/or an adjective (e.g., your before eyes), it is likely that these “early” prediction effects prior to the critical word reflected quicker reading of the determiner and/or adjective before the predicted noun. More importantly, we found that participants were more likely to engage in lexico-semantic prediction when reading to later interpret, as suggested by the larger prediction effects in reading to interpret than to recall. These results confirm our hypothesis that interpreters predict to a greater extent in SL comprehension in interpreting than in regular language comprehension.
Experiment 2
In this experiment, we examined whether the predictive advantage in reading to interpret over reading to recall is constrained by cognitive resources. To do this, apart from the 2 (predictability: predictable vs. unpredictable) x 2 (task: reading to interpret vs. reading to recall) design in Experiment 1a and 1b, we also manipulated cognitive load. In the low-load condition, participants read one sentence to later recall/interpret; in the high-load condition, they read two sentences (and the target sentence was always the second one) to later interpret/recall. Note that it is important to ensure that the two sentences were unrelated in discourse; otherwise, two sentences in the high-load condition would change the predictability of the critical word between the high- and low-load conditions and we would not be able to conclude whether any difference in prediction between the loads was due to availability of cognitive resources or due to changed predictability.
If the tendency to predict SL in interpreting is constrained by the availability of cognitive resources, we should expect enhanced prediction in SL comprehension (as compared to regular language comprehension) in the low-load condition (as we observed in Experiment 1a and 1b) but not in the high-load condition.
Method
Participants
Another 64 Chinese–English bilinguals (55 females; average age = 23.4, SD = 3.2, range = 18-31) from the same population as those in Experiments 1a and 1b participated in the experiment. As in Experiment 1, the participants reported having interpreting training courses in universities ranging from one month to more than 2 years and/or professional interpreting experience. They had an average age of acquisition for English of 8.0 (SD = 2.5, range = 4-13) and intermediate to high levels of English proficiency (corresponding to B1 to C1 in the Common European Framework) as assessed by the Quick Placement Test. All of them reported normal hearing, normal (or corrected-to-normal) vision, and no language disorders. Participants received 60 RMB as a reward after completing the experiment.
Design and materials
Experiment 2 adopted a design of 2 (predictability: predictable vs. unpredictable) x 2 (task: reading to interpret vs. reading to recall) x 2 (load: high vs. low load). The experimental materials were based on those in Experiment 1b, with 72 target sentences and 24 filler ones. Sentences in the low-load condition were the same as those in Experiment 1b. To create materials for the high load condition, we selected 48 simple sentences (4 to 6 words in each sentence), which were adapted from the BKΒ sentence lists (Bench, Kowal & Bamford, Reference Bench, Kowal and Bamford1979). In each of the eight lists we created according to Latin square design, we created 36 high-load target items (and also 12 filler sentence items) by pairing up each sentence in the low-load sentence with a BKΒ sentence (such that while there was only one sentence in each item in the low-load condition, there were a pair of unrelated sentences in each item in the high-load condition). We took care to make sure that there was no semantic relatedness between the BKB sentence and the target sentence in the pair, and all the BKB sentences paired with target sentences had the same number of words (5 words).
Procedure
The procedure was the same as that in Experiment 1a and 1b for trials in the low-load condition. For a trial in the high-load condition, after seeing a fixation cross, participants read the first (BKΒ) sentence, and then, after seeing another fixation, read the second sentence, before they recalled/interpreted the sentences. The experiment consisted of four blocks (two blocks for reading to interpret and the other two blocks for reading to recall, with the two tasks counterbalanced across participants). Each block had 18 target trials (half predictable and half unpredictable) and 6 filler trials (thus 24 trials in total, half low-load and half high-load, intermixed in a block). Each task was preceded by a set of 4 practice items. The whole experiment took approximately 60 min.
Results
As before, two researchers checked recall and interpreting outputs. Two participants were lost due to recording failure in the online experiment. For the remaining participants, we removed from further analyses 580 trials with a critical content error (12.99% of all the target trials), 13 trials with a task error (0.29%), and 86 trials with no or partial verbal response (1.93%). The overall error rates for different conditions in Experiment 2 were summarized in Table A1 in Appendix. As a result of these removals, another two participants were excluded altogether for having more than 40% of their target trials removed and another one participant for failing to correctly recall or interpret the first sentence in more than 40% of the trials in the high load condition. For the remaining data (from 59 participants), we further excluded RTs smaller than 100ms, as well as RTs more than 3 SDs beyond the mean of each experimental condition for each region (355 RTs, or 1.59% of all the target RTs).
The overall RTs of different reading regions in different conditions were shown in Fig 2 (see also Table A2 for more details of the effects). As in Experiment 1a and 1b, our analyses (see Table 3) revealed a significant effect of predictability across several regions (C-1, C, C+1 and C+2), with faster RTs in the predictable condition than in the unpredictable condition. Participants were also faster at reading in the C+2 region if the task was to interpret versus to recall.

Fig 2. RTs for the critical word and surrounding words in self-paced reading in Experiment 2 for the low-load (left panel) and high-load condition (right panel). Int = reading to interpret; rec = reading to recall; pred: = predictable; unpred = unpredictable.
Table 3. LME results for Experiment 2. Regression coefficients (βs) and their SEs are standardized; the intercept stands for the mean across all conditions. Significant effects in bold. Pred = predictability.
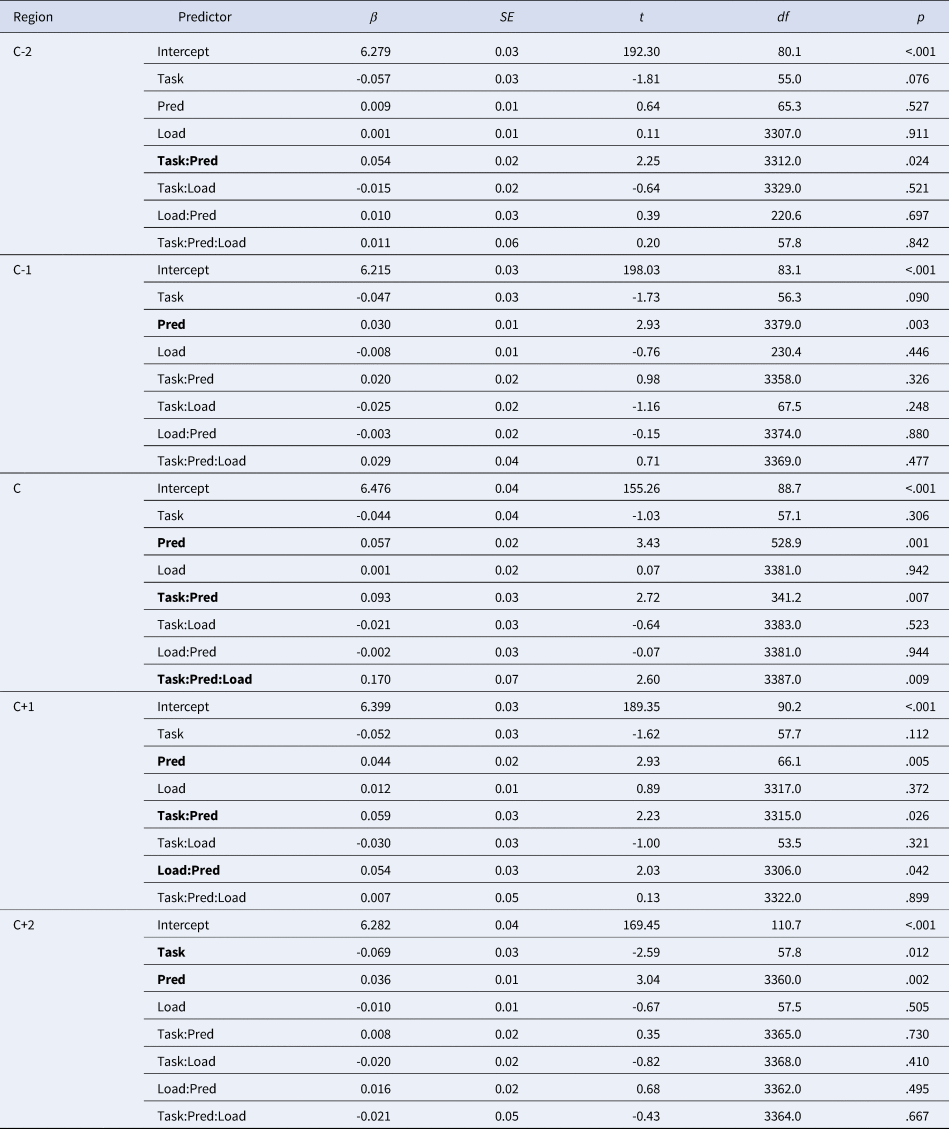
Note. The formula of the final LME models for the five reading regions were as follows:
C-2:lmer(logRT~Task*Pred*Load+(1+Task+Task:Pred:Load|Subject)+(1+Pred+Pred:Load|Item);
C-1: lmer(logRT~Task*Pred*Load +(1+Task|Subject)+(1+Load|Item)+(0+Task:Load|Item);
C: lmer(logRT~ Task*Pred*Load +(1+Task+Task:Pred|Subject)+(1+Pred|Item);
C+1: lmer(logRT~ Task*Pred*Load (1+Task+Task:Load|Subject)+(1+Pred|Item);
C+2: lmer(logRT~ Task*Pred*Load +(1+Task+Load|Subject)+(1|Item).
In addition, we observed a significant two-way interaction of load and predictability in the C+1 region. As in Experiment 1a and 1b, in the low-load condition (where participants read just the target sentence to later recall/interpret, as in Experiments 1a and 1b), there was a prediction effect, with faster RTs in the predictable condition than in the unpredictable condition (β = -0.071, SE = 0.02, t = -3.56, df = 202, p =.001); however, in the high-load condition (where participants first read a BKB sentence and then the target sentence before recalling/interpreting both sentences), such a prediction effect was not observed (β = -0.017, SE = 0.02, t = -0.83, df = 207, p = .407). This finding suggests that a high cognitive load limited participants’ ability to engage in linguistic prediction.
We also replicated the findings in Experiment 1a and 1b that participants engaged more in prediction in reading to interpret than to recall. In particular, we observed an interaction between task and predictability in three regions (C-2, C and C+1 regions). As in Experiment 1a and 1b, in reading to interpret, the prediction effect was significant or approaching significance (C-2 region: β = -0.036, SE = 0.02, t = -1.89, df = 185, p =. 060; C region: β = -0.104, SE = 0.02, t = -4.24, df = 67.7, p < .001; C+1 region: β = -0.074, SE = 0.02, t = -3.67, df = 207, p < .001). In contrast, in reading to recall, the prediction effect was non-significant (C-2 region: β = 0.017, SE = 0.02, t = 0.92, df = 181, p = .361; C region: β = -0.011, SE = 0.02, t = -0.46, df = 412.3, p = .646; C+1 region: β = -0.014, SE = 0.02, t = -0.70, df = 204, p = .487).
More importantly, the two-way interaction between task and predictability was further modulated by cognitive load in the reading of the critical word (the C region), suggesting that the predictive advantage in reading to interpret compared to reading to recall (as indicated by the interaction effect of task and predictability) differed between the low- and high-load conditions. In the low load condition, there was a significant interaction between task and predictability (β = 0.178, SE = 0.05, t = 3.78, df = 989.7, p <.001). Further analyses revealed a significant prediction effect in reading to interpret (β = -0.146, SE = 0.03, t = -4.31, df = 239, p <.001) but not in reading to recall (β = 0.033, SE = 0.03, t = 0.99, df = 1145, p = .322), thus replicating the predictive advantage in SL comprehension in Experiment 1a and 1b. In contrast, in the high load condition, the interaction between task and predictability was non-significant (β = 0.008, SE = 0.05, t = 0.16, df = 1013.0, p = .874), suggesting the prediction effect was comparable between the two reading tasks.
Discussion
We manipulated the load of information that participants needed to hold in working memory (one sentence vs. two sentences) for a later task (recalling or interpreting). We again observed that participants engaged in more lexico-semantic prediction in reading: they read a critical word and/or the following words more quickly when the critical word was predictable than when it was unpredictable from prior sentence context. Confirming Experiments 1a and 1b, participants predicted to a greater extent in reading to interpret than to recall (as indicated by the interaction between task and predictability in the critical word region and other regions). As in Experiments 1a and 1b, we also observed an “early” prediction effect prior to the critical word, again probably because of quicker reading of a determiner before a predicted noun (see Table A2 in Appendix for more details of the prediction effects across the experiments). Interestingly, this early prediction effect was also greater in reading to interpret than to recall, as suggested by the interaction between task and predictability in the C-2 region. Furthermore, replicating earlier findings (DeLong et al., Reference DeLong, Groppe, Urbach and Kutas2012; Huettig & Janse, Reference Huettig and Janse2016; Ito, Corley, et al., Reference Ito, Corley and Pickering2018), we also observed that linguistic prediction was constrained by cognitive load, with reduced prediction when participants had to keep more linguistic information in working memory. Importantly, we replicated our earlier findings that participants engaged in more prediction when they read to interpret than to recall. More importantly, the significant three-way interaction among task, predictability, and cognitive load suggests that, while interpreters predict to a greater extent in SL comprehension than in regular language comprehension, such a predictive advantage requires cognitive resources and thus disappears when cognitive resources are limited. Such a finding supports the hypothesis that prediction in interpreting consumes cognitive resources (Amos & Pickering, Reference Amos and Pickering2020).
General Discussion
In two experiments, we showed that bilinguals with prior interpreting training/experience are faster at reading a word if it is made contextually predictable than otherwise, a finding that replicates earlier demonstrations of lexico-semantic prediction in language comprehension (e.g., Kwon et al., Reference Kwon, Sturt and Liu2017; Foucart et al., Reference Foucart, Martin, Moreno and Costa2014). More importantly, we also showed that participants predict lexico-semantic information to a greater extent when they read to later interpret than to recall, suggesting that interpretersFootnote 2 make more use of linguistic prediction in SL comprehension in interpreting than in regular language comprehension. Such a predictive advantage in interpreting, however, disappeared when participants were under a high cognitive load, which suggests that the predictive machinery in SL comprehension requires cognitive resources (Amos & Pickering, Reference Amos and Pickering2020).
But is it possible that the effect of predictability reflects integration instead of prediction of the critical word? That is, in reading Without the sunglasses/hat, the sun will hurt your eyes on the beach, participants might not predict lexico-semantics of eyes but instead more easily integrate it into the prior context in the predictable condition than in the unpredictable condition. While this is possible, there is evidence that in predictive contexts like those in our experiments, people do pre-activate certain lexico-semantic content of a word, as suggested by prediction effects on a classifier (e.g., Kwon et al., Reference Kwon, Sturt and Liu2017) or article (e.g., Martin et al., Reference Martin, Branzi and Bar2018) preceding a predicted noun phrase. Thus, while it may not lead to the prediction of exact lexical representations or syntactic forms, prior context can at least generate certain expectations about upcoming lexico-semantic content and prepare comprehenders for that information (Ferreira & Chantavarin, Reference Ferreira and Chantavarin2018). The finding that interpreters are predictive of SL content is consistent with the recent hypothesis that interpreters use predictive processing in both SL and TL processing to ensure timely delivery (e.g., Amos & Pickering, Reference Amos and Pickering2020). In fact, the finding that SL comprehension in interpreting involves more prediction than regular language comprehension suggests that interpreters make extra use of the prediction machinery to anticipate SL input and to better prepare TL production (e.g., Chernov, Reference Chernov, Lambert and Moser-Mercer1994; Moser, Reference Moser, Gerver and Sinaiko1978).
But what enables interpreters to have more prediction in SL comprehension in interpreting than in regular language comprehension? One could argue that reading to recall and reading to interpret differ in the depth of processing, with participants adopting shallower processing, and hence a smaller prediction effect, in the former task than in the latter task. For example, when participants read a sentence to later recall, they might just quickly skim through the text prior to the critical word (e.g., Without the sunglasses/hat, the sun will hurt) and arrive at only a more cursory apprehension of the contextual information (e.g., Sanford, Reference Sanford2002; Sanford & Sturt, Reference Sanford and Sturt2002), in turn leading to a reduced prediction effect when the critical word was encountered. However, such an account would predict that reading times should be shorter in reading to recall than in reading to interpret, contrary to our observation: reading times were comparable between the two tasks in most reading regions (as reflected by the non-significant main effects of task; see Table 2 and 3).
Moreover, if the difference in depth processing led to different prediction effects between tasks, effects of other lexical characteristics such as word frequency should also have been affected (see also Brothers, Swaab & Traxler, Reference Brothers, Swaab and Traxler2017). Following Brothers et al. (Reference Brothers, Swaab and Traxler2017), we performed an analysis to test whether the frequency effect for critical words differed between the two tasks (i.e., interaction between frequency and task). Our results revealed no significant interactions between frequency and task in our experiments (Experiment 1a: β = 0.006, SE = 0.02, t = 0.26, df = 106.6, p = .793; Experiment 1b: β = -0.035, SE = 0.02, t = -1.40, df = 47.2, p = .167; Experiment 2: β = -0.022, SE = 0.02, t = -1.11, df = 98.6, p = .270)Footnote 3. This suggested that the difference in the prediction effect could not be attributed to differences in processing depth or general attentional demands between the two tasks.
The comparable reading times between reading to recall and reading to interpret is also incompatible with the possibility that, in the reading to recall task, participants focused on memorizing and rehearsing the surface form of a sentence and were therefore discouraged from engaging in lexico-semantic prediction. If the account were to be true, one should expect participants to be much slower in reading a sentence to be later recalled than to be later interpreted, contrary to what we observed. In fact, as we discussed earlier, there is much evidence that people do not engage in verbatim memorization when they read sentences to later recall (Lombardi & Potter, Reference Lombardi and Potter1992; Potter & Lombardi, Reference Potter and Lombardi1990).
A more plausible explanation for the enhanced prediction in SL comprehension is that enhanced SL prediction in interpreting results from the need for timely TL delivery. While consecutive interpreting differs from simultaneous interpreting in how quickly the TL needs to be delivered, there is evidence that consecutive interpreters do activate TL (e.g., lexical or syntactic) representations that correspond to SL input during SL comprehension (i.e., parallel processing of TL) in order to guarantee speedy TL delivery at the end of the SL (e.g., Macizo & Bajo, Reference Macizo and Bajo2006), especially when they interpret from L2 into L1 (e.g., Dong & Lin, Reference Dong and Lin2013). Such parallel processing not only enables consecutive interpreters to start interpreting right after the end of SL delivery, but also enables them to avoid disfluency during TL output. Note that TL is often delivered after a relatively large chunk of SL in consecutive interpreting (compared to simultaneous interpreting); therefore, TL disfluency is likely to occur if no parallel processing of TL took place during SL comprehension and all the transcoding of a large amount of SL information into TL information had to take place in TL production.
We therefore put forward an account whereby SL prediction is facilitated by parallel processing of TL in SL comprehension (see Figure 3). If SL input is lexico-semantically predictive of a particular word in the SL, interpreters can then, in TL preparation, anticipate and covertly produce the TL equivalent of the predicted word, which can feed back to further enhance the prediction of the SL word. For instance, in reading the English SL input without the sunglasses/hat, the sun will hurt your eyes on the beach, consecutive interpreters covertly produce key TL (i.e., Chinese) lexical and syntactic representations, which can lead to the covert production of yan3jing1 (“eyes”), therefore enhancing the prediction of the SL word eyes.

Figure 3. Prediction-by-production in both SL and TL in the SL comprehension of Without the sunglasses, the sun will hurt your … before eyes is comprehended. The words in green are covertly produced words in respectively the SL and the TL and arrows represent facilitation of the incoming word SL word eyes from covertly produced words. Note that the output of TL parallel processing can be TL phrases and sentence fragments instead of fully-form sentences (the phrases in the box of parallel processing of TL).
The predictive machinery in SL comprehension in interpreting requires cognitive “fuel”, as Amos and Pickering (Reference Amos and Pickering2020) have hypothesized. That is, when interpreters are under a high cognitive load, SL comprehension (i.e., reading to interpret) is no more predictive than regular language comprehension (i.e., reading to recall). This finding is congruent with earlier observations that linguistic prediction is costly, as they are used to a lesser extent by people with more limited cognitive resources (DeLong et al., Reference DeLong, Groppe, Urbach and Kutas2012; Huettig & Janse, Reference Huettig and Janse2016; Ito, Corley, et al., Reference Ito, Corley and Pickering2018). In our proposed mechanism in Figure 3, it is likely that the covert production of the TL word yan3jing1 (“eyes”) is discouraged in parallel TL processing when there are not enough cognitive resources. For example, it is possible that working memory is prioritized for memorizing source language words in the high-load condition such that there is not enough working memory support for making TL predictions, hence resulting in the comparability in predictive processing between SL comprehension and regular language comprehension.
Our findings and the proposed mechanism in Figure 3 extend the theoretical account of linguistic prediction in simultaneous interpreting offered in Amos and Pickering (Reference Amos and Pickering2020). While they assumed that (simultaneous) interpreting involves the interpreter simulating the speaker's SL production, hence leading to SL prediction (as people do in regular language comprehension), our findings further showed that interpreters engage in more extensive SL prediction. Our findings also suggest that predicted content may feed bi-directionally between SL and TL. That is, while predicted SL content can feed into TL production preparation, leading to timely delivery of interpreting, as argued by Amos and Pickering (Reference Amos and Pickering2020), predicted TL content may also feed back to facilitate SL prediction (which could be one of the reasons why SL comprehension involves more prediction, as we argued above). In addition, our study also demonstrated that, instead of focusing on TL output, interpreting research should also examine SL comprehension in order to gain a more comprehensive understanding of the cognitive processes underlying interpreting.
Finally, we would like to discuss possible limitations of the current study and directions for future research. First of all, our paradigm involved reading SL sentences instead of listening to them as in typical consecutive interpreting (though see Seeber et al., Reference Seeber, Keller and Hervais-Adelman2020). It is worthwhile too for future research to investigate whether the predictive advantage in SL comprehension can be replicated in more realistic settings of consecutive interpreting involving spoken SL comprehension instead of written SL comprehension. Second, our participants were bilinguals with prior interpreting training/experience instead of professional interpreters. It remains to be tested if similar results can be obtained when professional interpreters are used. Third, it is worthwhile for future research to consider the effect of interpreting direction. Parallel processing of TL is engaged to a greater extent in L2-to-L1 interpreting compared to L1-to-L2 interpreting (e.g., Dong & Lin, Reference Dong and Lin2013). If the enhanced prediction in SL comprehension is indeed a feedback effect due to TL parallel processing, we should then expect a smaller prediction advantage in L2-to-L1 than L1-to-L2 interpreting, a prediction that we leave for future research. Finally, further research can investigate whether repeated enhanced prediction in interpreting practice may lead interpreters to develop specialized predictive machinery in regular language comprehension. As we mentioned briefly before, there is tentative evidence that professional interpreters may indeed engage more predictive processing than non-interpreters even in daily communication (Fan, Reference Fan2013; Lozano-Argüelles et al., Reference Lozano-Argüelles, Sagarra and Casillas2020; Lozano-Argüelles & Sagarra, Reference Lozano-Argüelles and Sagarra2021).
In summary, we showed that interpreters engage in predictive language processing to a greater extent in source language comprehension in consecutive interpreting than in regular language comprehension (e.g., comprehension for recall). Such enhanced prediction in interpreting is reduced when interpreters are under a high cognitive load.
Acknowledgments
All experimental materials, data, and analytical scripts are publicly available at Open Science Framework (osf.io/yrxh5/).
The first two authors contributed equally to the research. The research was supported by a GRF grant (Project Number: 14600220) and a CUHK Faculty of Arts internal grant, both awarded to Z.G. Cai. We thank Zebo Xu, Xufeng Duan and Wenyi Zhang for their help in data collection and thank Aine Ito and David Haslett for comments on an earlier version of the manuscript.
Appendix
Table A1. Error rates and the standard error of mean (in bracket) in Exp1 and Exp 2. Pred = predictability;

Table A2. Mean reading times (in milliseconds) and the standard error of mean (in brackets) of C and C +1 regions across tasks and conditions in Exp1 and Exp 2. Pred = predictability; Diff = difference of mean reading times between predictable and unpredictable conditions (prediction effects).
